Deep Dense and Convolutional Autoencoders for Unsupervised Anomaly Detection in Machine Condition Sounds
arxiv(2020)
摘要
This technical report describes two methods that were developed for Task 2 of the DCASE 2020 challenge. The challenge involves an unsupervised learning to detect anomalous sounds, thus only normal machine working condition samples are available during the training process. The two methods involve deep autoencoders, based on dense and convolutional architectures that use melspectogram processed sound features. Experiments were held, using the six machine type datasets of the challenge. Overall, competitive results were achieved by the proposed dense and convolutional AE, outperforming the baseline challenge method.
更多查看译文
关键词
unsupervised anomaly detection,convolutional autoencoders,deep dense,machine condition
AI 理解论文
溯源树
样例
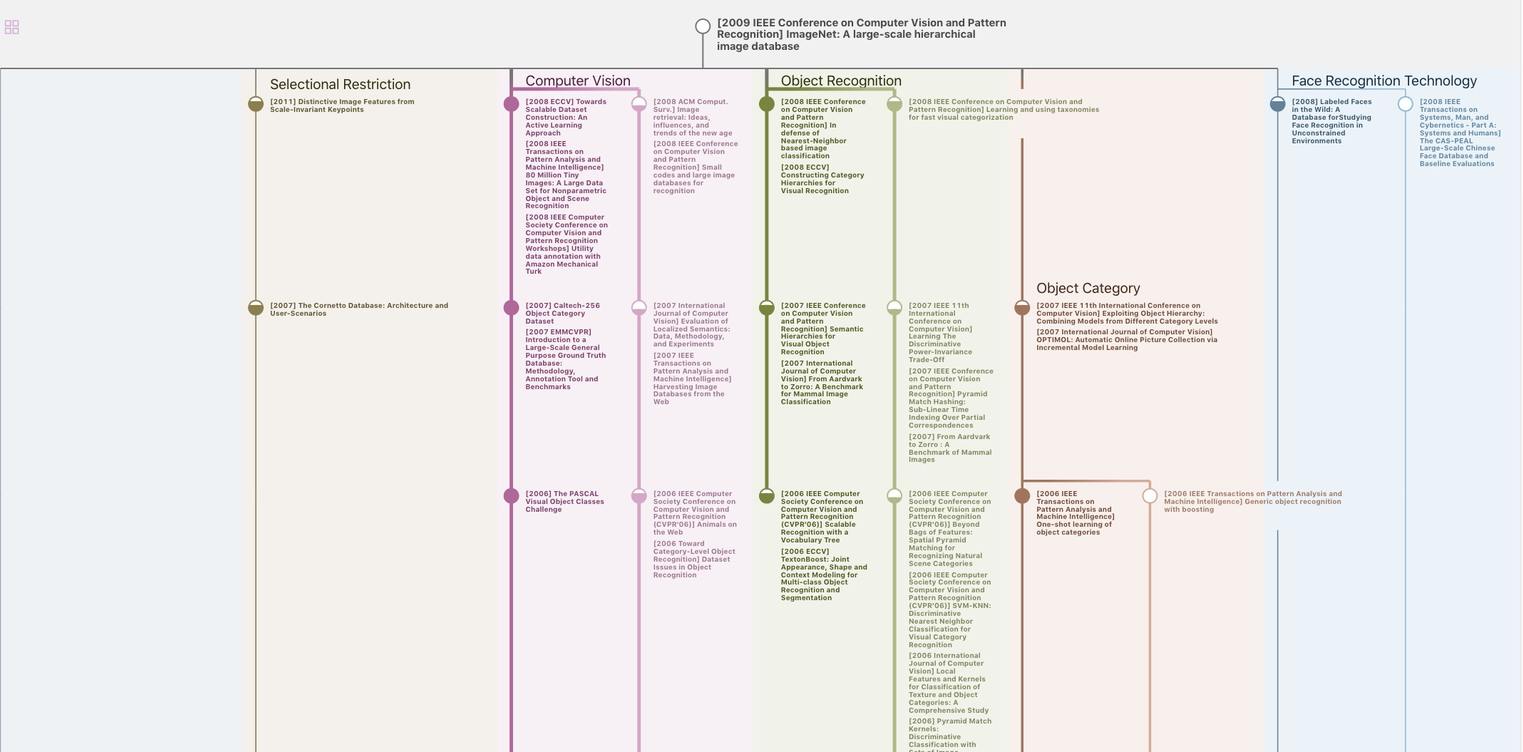
生成溯源树,研究论文发展脉络
Chat Paper
正在生成论文摘要