Video Moment Localization using Object Evidence and Reverse Captioning
arxiv(2020)
摘要
We address the problem of language-based temporal localization of moments in untrimmed videos. Compared to temporal localization with fixed categories, this problem is more challenging as the language-based queries have no predefined activity classes and may also contain complex descriptions. Current state-of-the-art model MAC addresses it by mining activity concepts from both video and language modalities. This method encodes the semantic activity concepts from the verb/object pair in a language query and leverages visual activity concepts from video activity classification prediction scores. We propose "Multi-faceted VideoMoment Localizer" (MML), an extension of MAC model by the introduction of visual object evidence via object segmentation masks and video understanding features via video captioning. Furthermore, we improve language modelling in sentence embedding. We experimented on Charades-STA dataset and identified that MML outperforms MAC baseline by 4.93% and 1.70% on R@1 and R@5metrics respectively. Our code and pre-trained model are publicly available at https://github.com/madhawav/MML.
更多查看译文
关键词
object evidence,captioning,localization,video
AI 理解论文
溯源树
样例
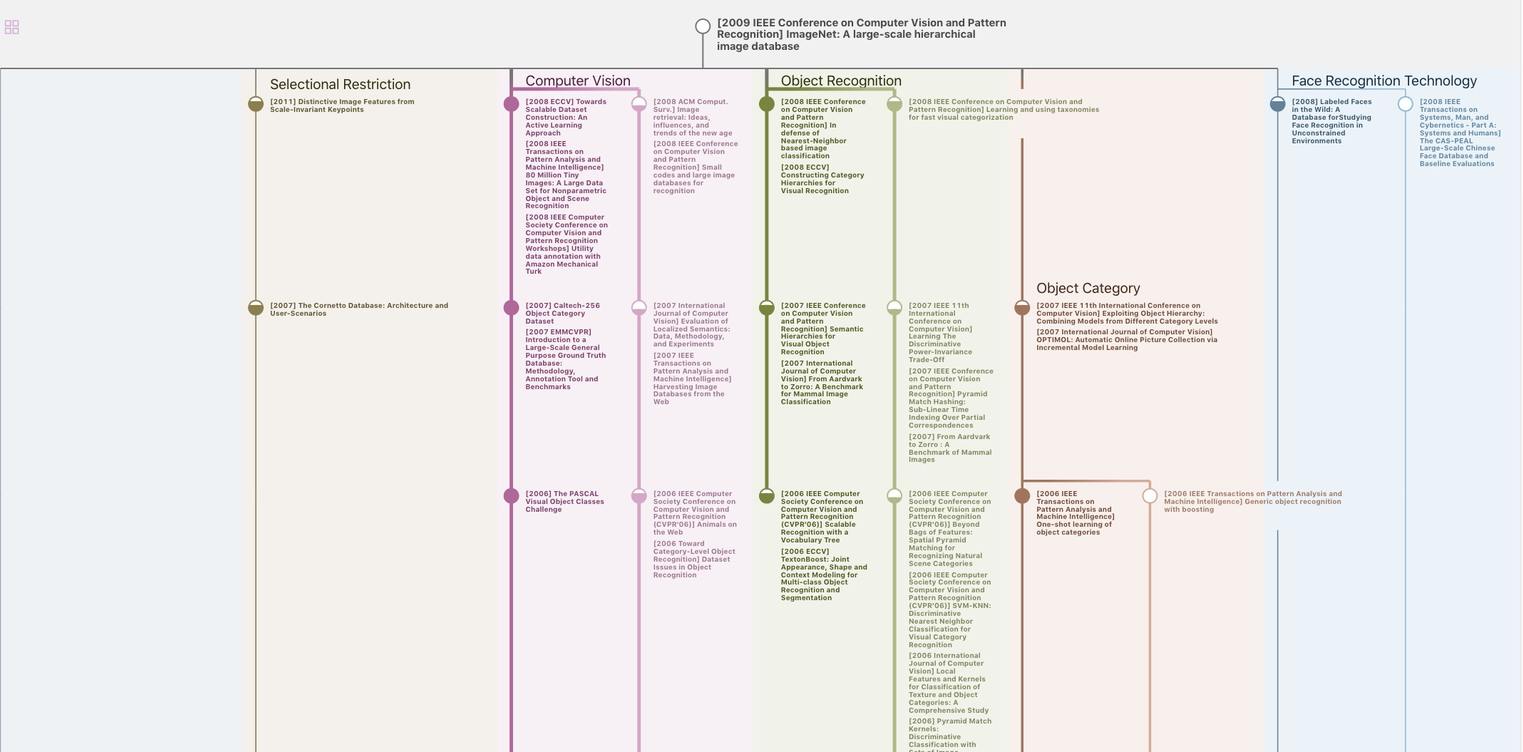
生成溯源树,研究论文发展脉络
Chat Paper
正在生成论文摘要