Fair Hierarchical Clustering
NIPS 2020(2020)
Abstract
As machine learning has become more prevalent, researchers have begun to recognize the necessity of ensuring machine learning systems are fair. Recently, there has been an interest in defining a notion of fairness that mitigates over-representation in traditional clustering. In this paper we extend this notion to hierarchical clustering, where the goal is to recursively partition the data to optimize a specific objective. For various natural objectives, we obtain simple, efficient algorithms to find a provably good fair hierarchical clustering. Empirically, we show that our algorithms can find a fair hierarchical clustering, with only a negligible loss in the objective.
MoreTranslated text
AI Read Science
Must-Reading Tree
Example
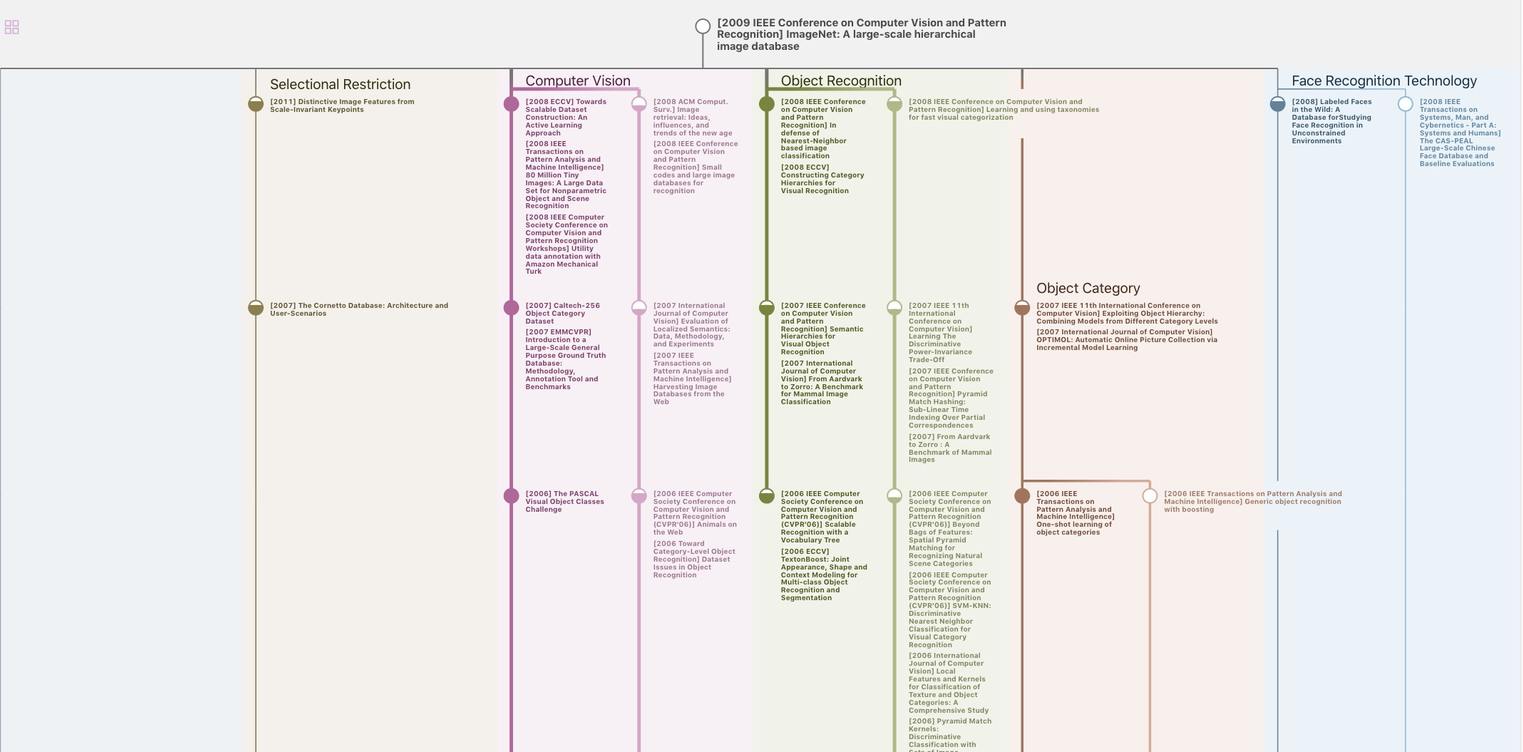
Generate MRT to find the research sequence of this paper
Chat Paper
Summary is being generated by the instructions you defined