SAMBA: safe model-based & active reinforcement learning
MACHINE LEARNING(2022)
摘要
In this paper, we propose SAMBA, a novel framework for safe reinforcement learning that combines aspects from probabilistic modelling, information theory, and statistics. Our method builds upon PILCO to enable active exploration using novel acquisition functions for out-of-sample Gaussian process evaluation optimised through a multi-objective problem that supports conditional-value-at-risk constraints. We evaluate our algorithm on a variety of safe dynamical system benchmarks involving both low and high-dimensional state representations. Our results show orders of magnitude reductions in samples and violations compared to state-of-the-art methods. Lastly, we provide intuition as to the effectiveness of the framework by a detailed analysis of our acquisition functions and safety constraints.
更多查看译文
关键词
Gaussian process,Safe reinforcement learning,Active learning
AI 理解论文
溯源树
样例
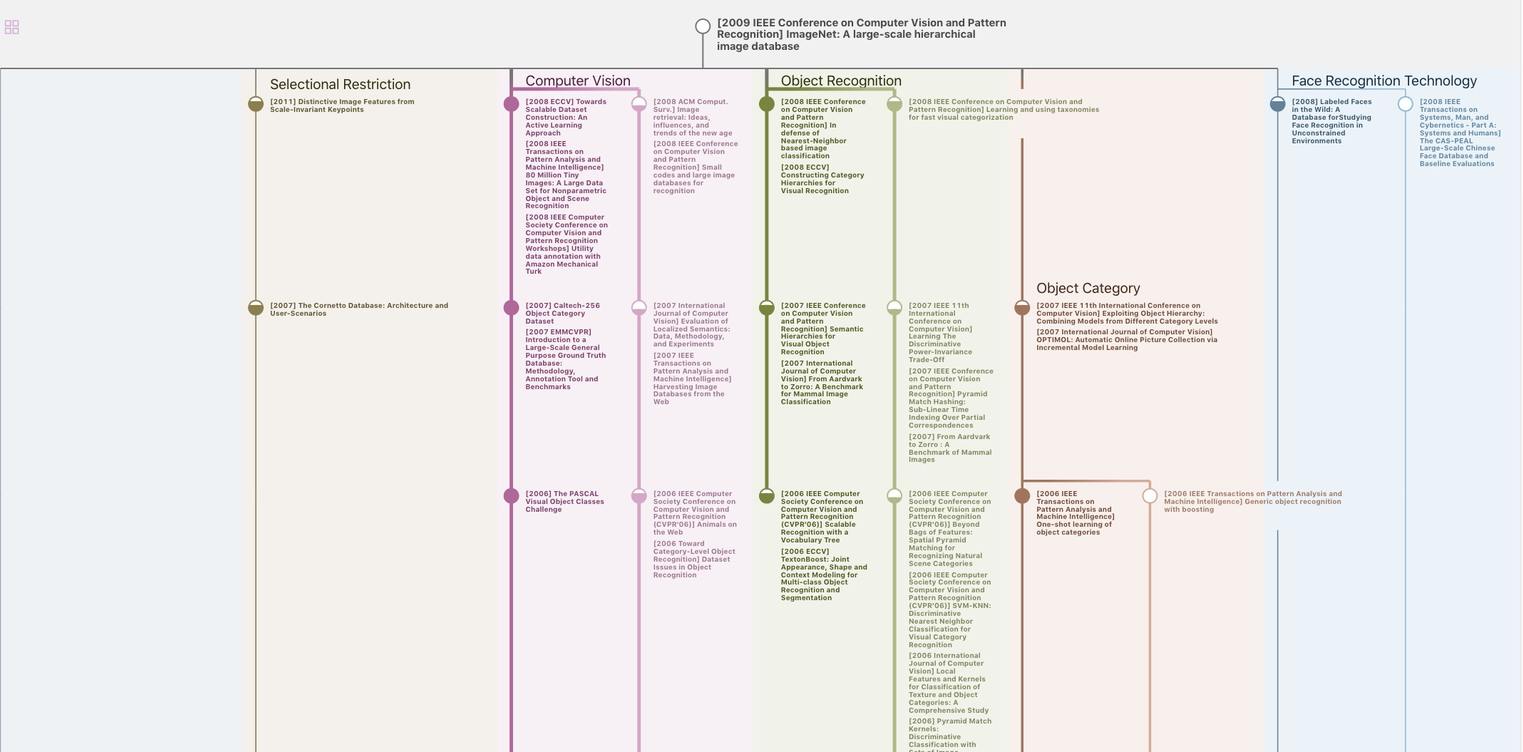
生成溯源树,研究论文发展脉络
Chat Paper
正在生成论文摘要