Defining a synthetic data generator for realistic electric vehicle charging sessions
e-Energy '20: The Eleventh ACM International Conference on Future Energy Systems Virtual Event Australia June, 2020(2020)
摘要
Electric vehicle (EV) charging stations have become prominent in electricity grids in the past years. Analysis of EV charging sessions is useful for flexibility analysis, load balancing, offering incentives to customers, etc. Yet, limited availability of such EV sessions' data hinders further development in these fields. Addressing this need for publicly available and realistic data, we develop a synthetic data generator (SDG) for EV charging sessions. Our SDG assumes the EV inter-arrival time to follow an exponential distribution. Departure times are modeled by defining a conditional probability density function (pdf) for connection times. This pdf for connection time and required energy is fitted by Gaussian mixture models. Since we train our SDG using a large real-world dataset, its output is realistic.
更多查看译文
AI 理解论文
溯源树
样例
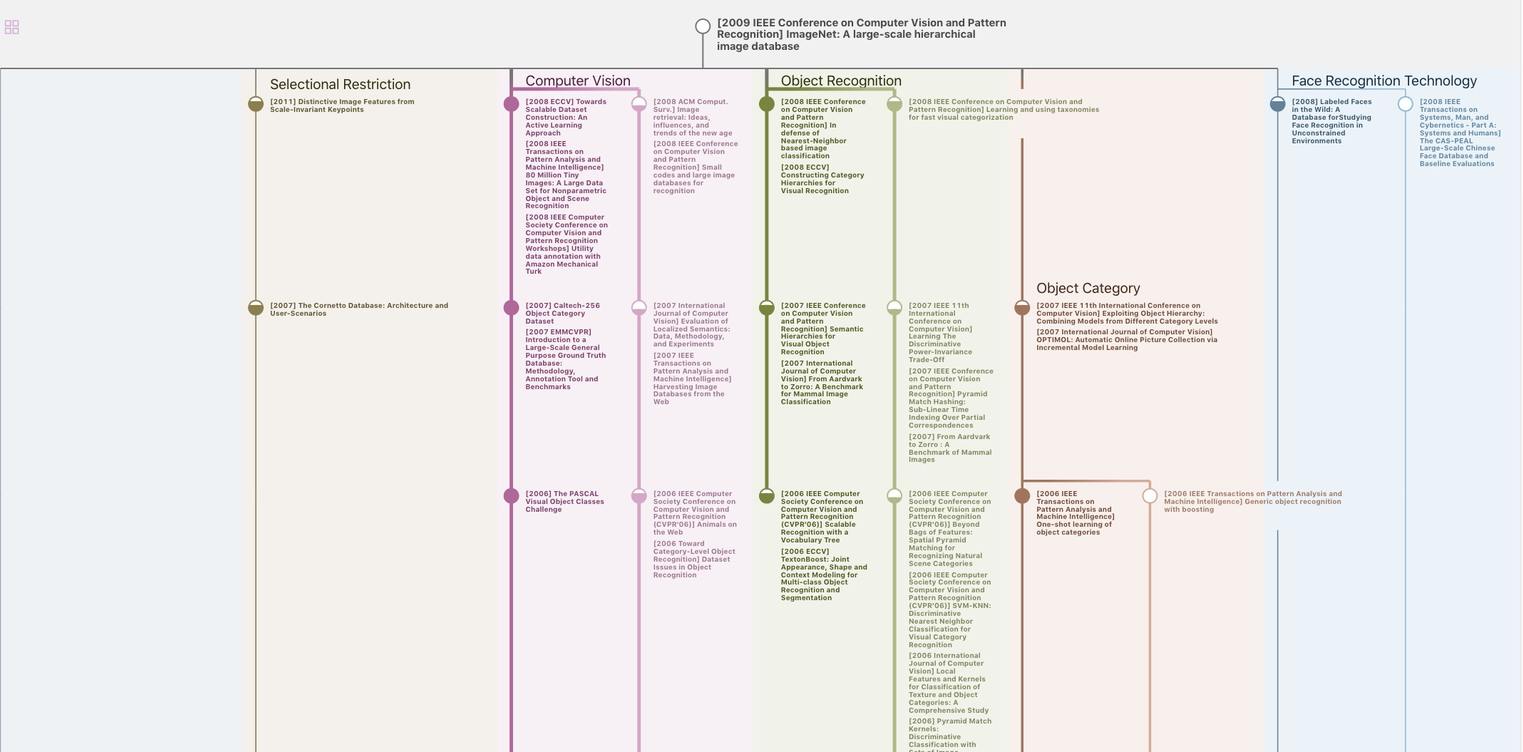
生成溯源树,研究论文发展脉络
Chat Paper
正在生成论文摘要