To Blend Or Not To Blend? A Framework For Nationwide Landsat-Modis Data Selection For Crop Yield Prediction
REMOTE SENSING(2020)
摘要
The onus for monitoring crop growth from space is its ability to be applied anytime and anywhere, to produce crop yield estimates that are consistent at both the subfield scale for farming management strategies and the country level for national crop yield assessment. Historically, the requirements for satellites to successfully monitor crop growth and yield differed depending on the extent of the area being monitored. Diverging imaging capabilities can be reconciled by blending images from high-temporal-frequency (HTF) and high-spatial-resolution (HSR) sensors to produce images that possess both HTF and HSR characteristics across large areas. We evaluated the relative performance of Moderate Resolution Imaging Spectroradiometer (MODIS), Landsat, and blended imagery for crop yield estimates (2009-2015) using a carbon-turnover yield model deployed across the Australian cropping area. Based on the fraction of missing Landsat observations, we further developed a parsimonious framework to inform when and where blending is beneficial for nationwide crop yield prediction at a finer scale (i.e., the 25-m pixel resolution). Landsat provided the best yield predictions when no observations were missing, which occurred in 17% of the cropping area of Australia. Blending was preferred when <42% of Landsat observations were missing, which occurred in 33% of the cropping area of Australia. MODIS produced a lower prediction error when >= 42% of the Landsat images were missing (similar to 50% of the cropping area). By identifying when and where blending outperforms predictions from either Landsat or MODIS, the proposed framework enables more accurate monitoring of biophysical processes and yields, while keeping computational costs low.
更多查看译文
关键词
MODIS,Landsat,data blending,crop yield prediction,gap-filling
AI 理解论文
溯源树
样例
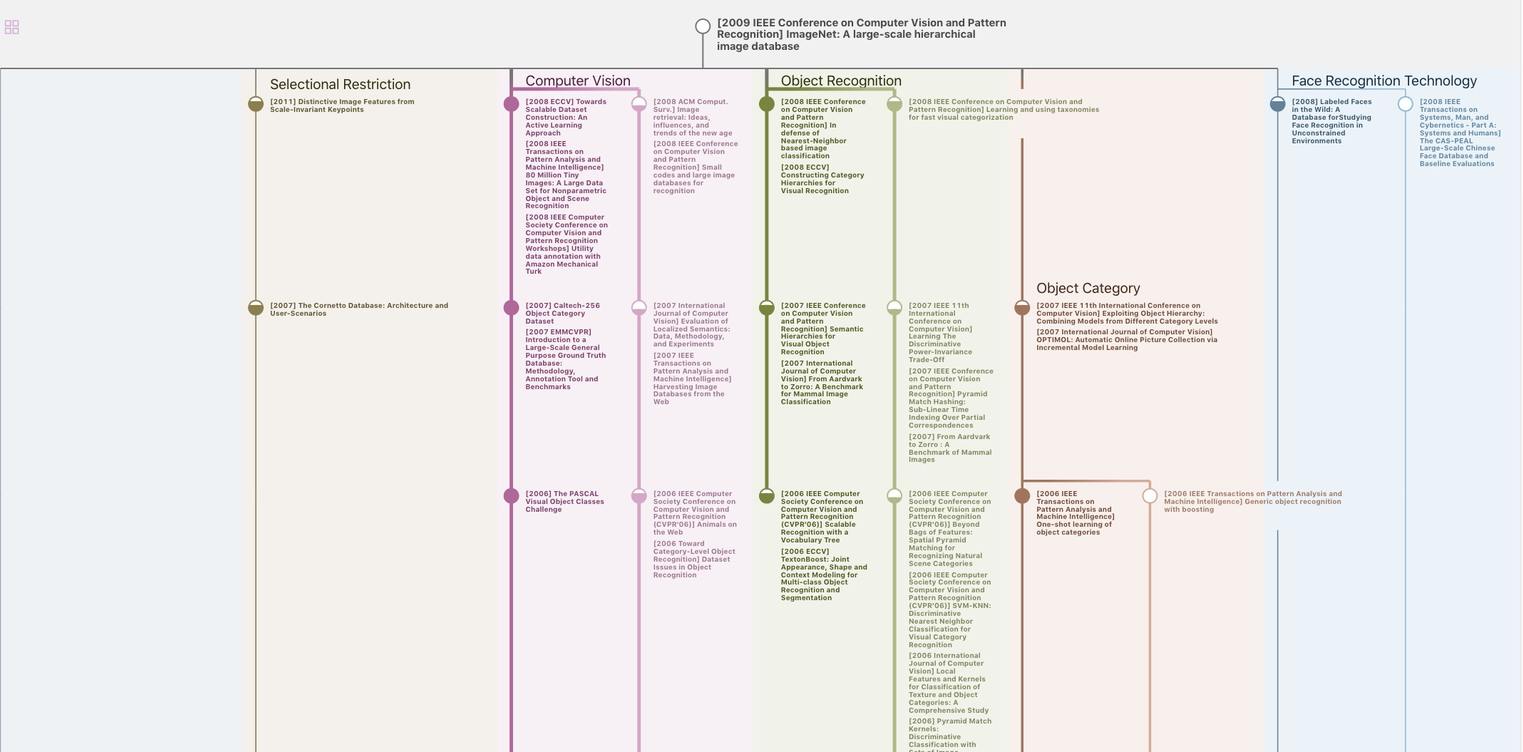
生成溯源树,研究论文发展脉络
Chat Paper
正在生成论文摘要