Using Blackbox ML Techniques to Diagnose QoE Problems for an IPTV Service
NOMS 2020 - 2020 IEEE/IFIP Network Operations and Management Symposium(2020)
Abstract
IPTV services continue to disrupt the traditional cable-based TV delivery and grow in popularity. However, they also face challenges related to the delivery of stable and problem free video streams to the end users while minimizing downtime due to the inherent complexity and uncertainty associated with the IP based networks. In this work, we share our experience on addressing this service assurance problem for a commercial IPTV service provider. The key focus here is on the root cause inference aspects of the service assurance aimed at providing QoE problem hints with localization in the delivery network to the technicians of network service provider. To solve this problem, we investigate various ML-based model interpretability approaches to extract insights from predictive models built for the alarm generation. Key focus is on Blackbox model interpretability approaches namely LIME and SHAP with their associated pros and cons while at the same time comparing these techniques to the popular model agnostic (i.e., Whitebox) way of explainability based on XGB. One of the findings is that SHAP Blackbox technique is most suitable for diagnosing IPTV QoE problems. However, having the downside of long computational time, for cases where the predictive model is not tree-based such as XGB or Random Forest.
MoreTranslated text
Key words
Machine Learning (ML),STB (Set-top-box),Internet Protocol Television (IPTV),Service Assurance,Prediction,Quality of Experience (QoE),LIME (Local Interpretable Model-agnostic Explanations),SHAP (SHapley Additive exPlanations),Bayesian Network (BN)
AI Read Science
Must-Reading Tree
Example
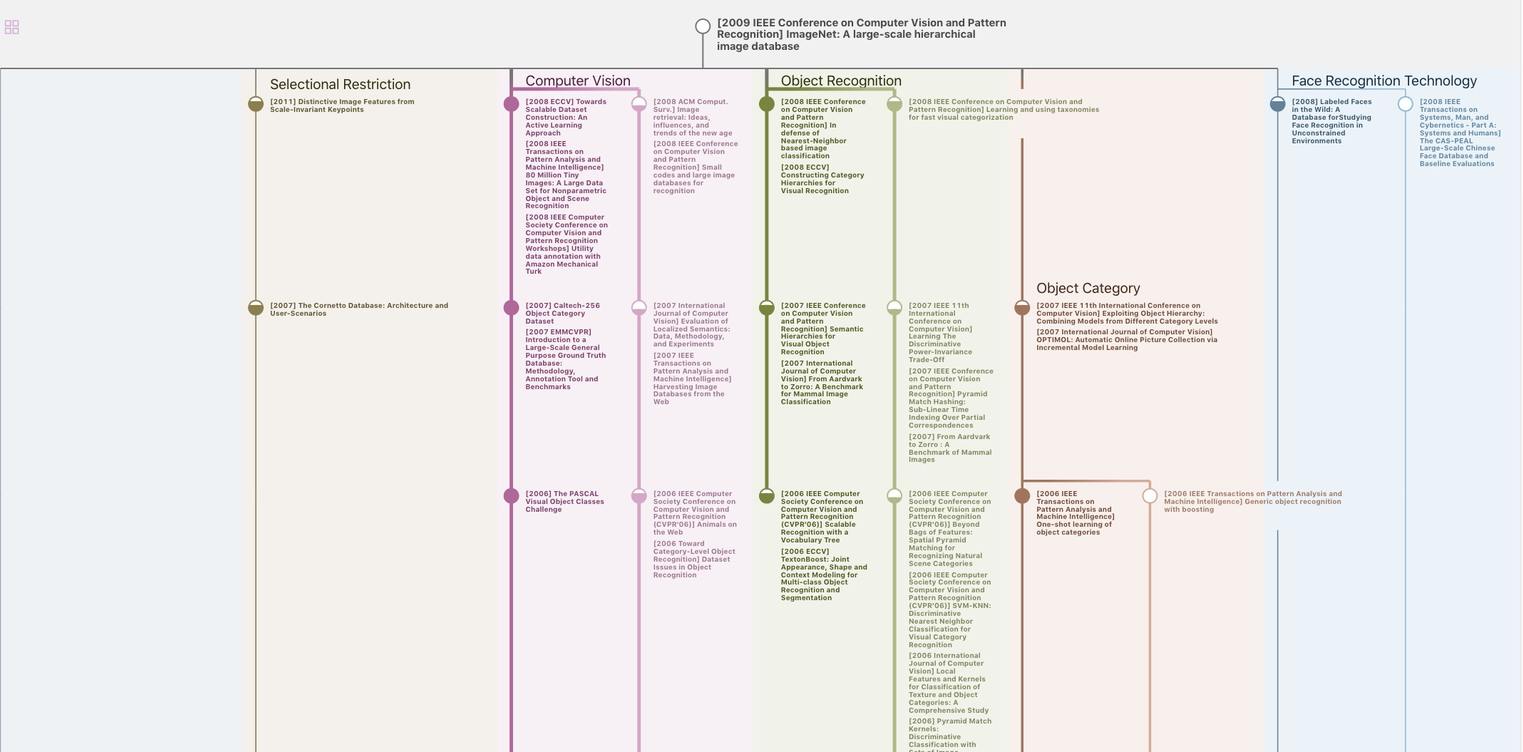
Generate MRT to find the research sequence of this paper
Chat Paper
Summary is being generated by the instructions you defined