Learnability with Indirect Supervision Signals
NIPS 2020(2020)
摘要
Learning from indirect supervision signals is important in real-world AI applications when, often, gold labels are missing or too costly. In this paper, we develop a unified theoretical framework for multi-class classification when the supervision is provided by a variable that contains nonzero mutual information with the gold label. The nature of this problem is determined by (i) the transition probability from the gold labels to the indirect supervision variables and (ii) the learner's prior knowledge about the transition. Our framework relaxes assumptions made in the literature, and supports learning with unknown, non-invertible and instance-dependent transitions. Our theory introduces a novel concept called \emph{separation}, which characterizes the learnability and generalization bounds. We also demonstrate the application of our framework via concrete novel results in a variety of learning scenarios such as learning with superset annotations and joint supervision signals.
更多查看译文
关键词
indirect supervision signals
AI 理解论文
溯源树
样例
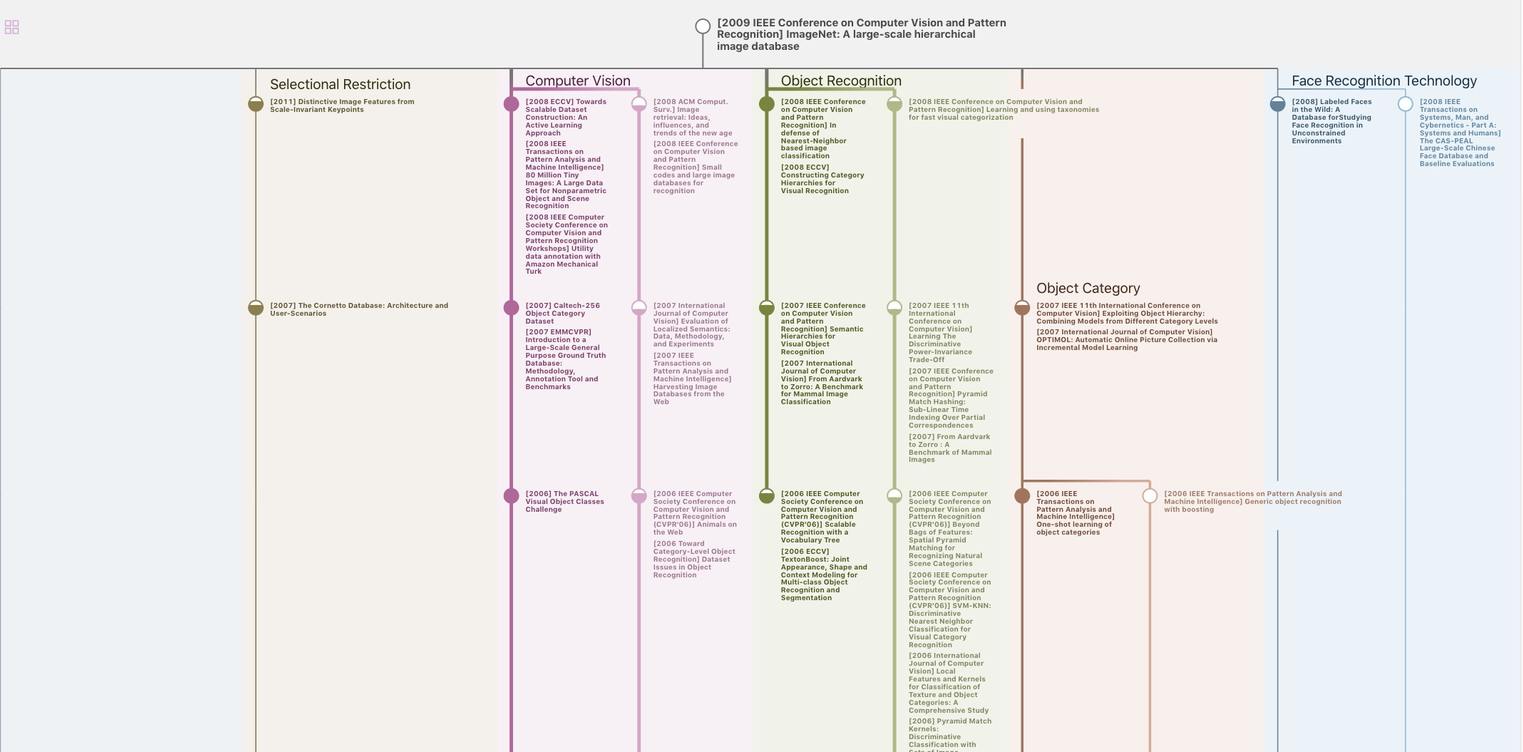
生成溯源树,研究论文发展脉络
Chat Paper
正在生成论文摘要