Neural Ensemble Search for Performant and Calibrated Predictions
arxiv(2020)
摘要
Ensembles of neural networks achieve superior performance compared to stand-alone networks not only in terms of accuracy on in-distribution data but also on data with distributional shift alongside improved uncertainty calibration. Diversity among networks in an ensemble is believed to be key for building strong ensembles, but typical approaches only ensemble different weight vectors of a fixed architecture. Instead, we investigate neural architecture search (NAS) for explicitly constructing ensembles to exploit diversity among networks of varying architectures and to achieve robustness against distributional shift. By directly optimizing ensemble performance, our methods implicitly encourage diversity among networks, without the need to explicitly define diversity. We find that the resulting ensembles are more diverse compared to ensembles composed of a fixed architecture and are therefore also more powerful. We show significant improvements in ensemble performance on image classification tasks both for in-distribution data and during distributional shift with better uncertainty calibration.
更多查看译文
关键词
neural ensemble search,uncertainty estimation
AI 理解论文
溯源树
样例
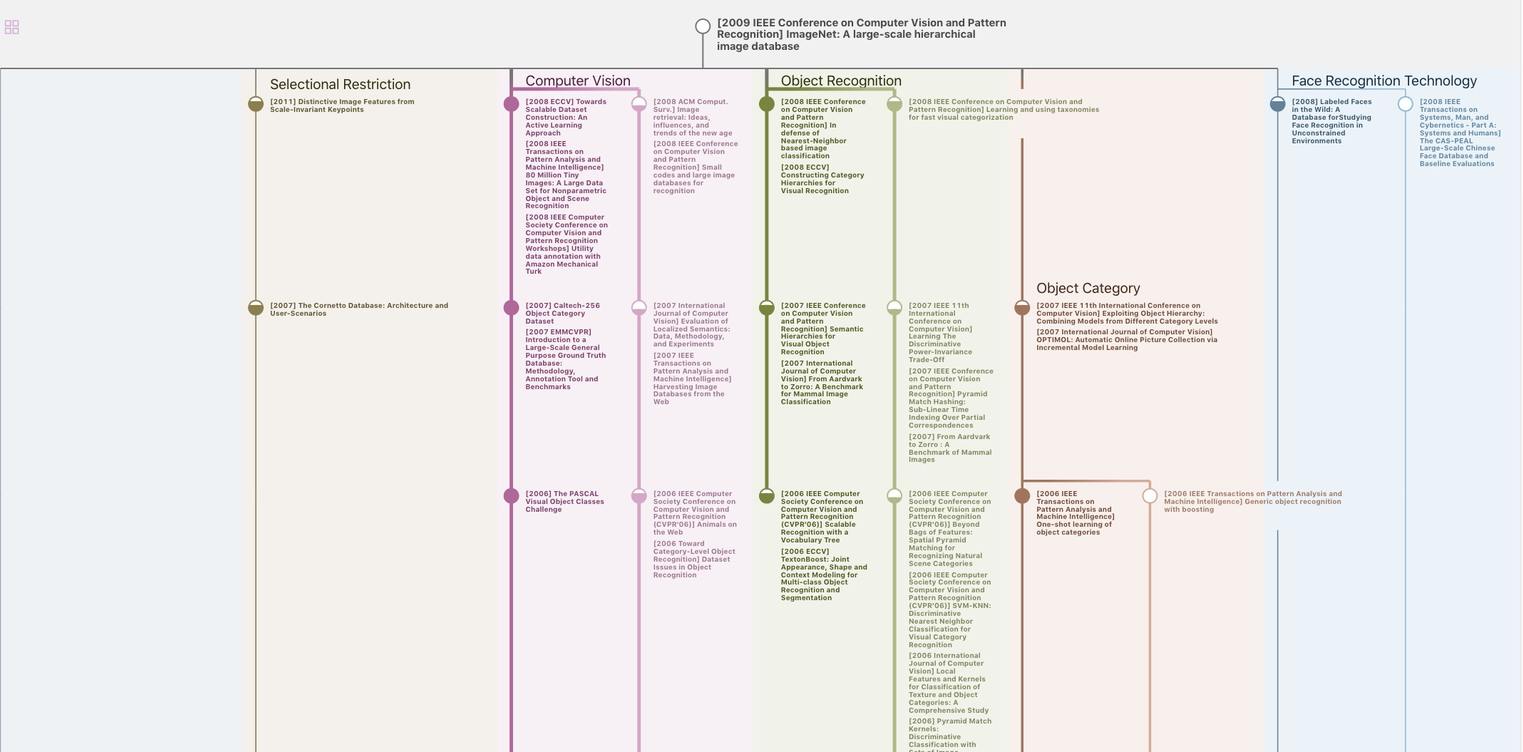
生成溯源树,研究论文发展脉络
Chat Paper
正在生成论文摘要