Entropic gradient descent algorithms and wide flat minima
JOURNAL OF STATISTICAL MECHANICS-THEORY AND EXPERIMENT(2021)
摘要
The properties of flat minima in the empirical risk landscape of neural networks have been debated for some time. Increasing evidence suggests they possess better generalization capabilities with respect to sharp ones. In this work we first discuss the relationship between alternative measures of flatness: The local entropy, which is useful for analysis and algorithm development, and the local energy, which is easier to compute and was shown empirically in extensive tests on state-of-the-art networks to be the best predictor of generalization capabilities. We show semi-analytically in simple controlled scenarios that these two measures correlate strongly with each other and with generalization. Then, we extend the analysis to the deep learning scenario by extensive numerical validations. We study two algorithms, Entropy-SGD and Replicated-SGD, that explicitly include the local entropy in the optimization objective. We devise a training schedule by which we consistently find flatter minima (using both flatness measures), and improve the generalization error for common architectures (e.g. ResNet, EfficientNet).
更多查看译文
关键词
deep learning,machine learning,message-passing algorithms
AI 理解论文
溯源树
样例
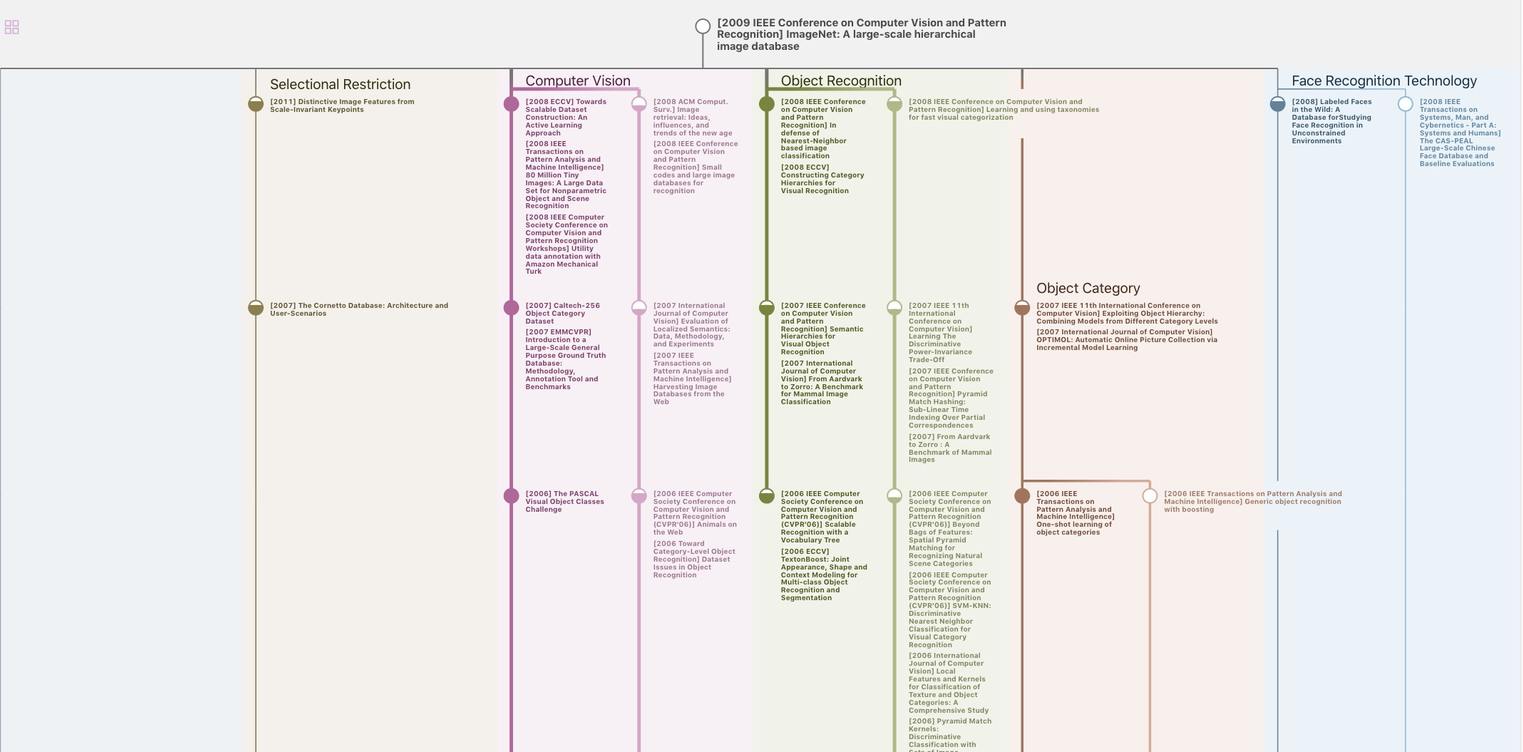
生成溯源树,研究论文发展脉络
Chat Paper
正在生成论文摘要