Hyper RPCA: Joint Maximum Correntropy Criterion and Laplacian Scale Mixture Modeling on-the-Fly for Moving Object Detection
IEEE Transactions on Multimedia(2023)
摘要
Moving object detection is critical for automated video analysis in many vision-related tasks, such as surveillance tracking, video compression coding, etc. Robust Principal Component Analysis (RPCA), as one of the most popular moving object modelling methods, aims to separate the temporally-varying (i.e., moving) foreground objects from the static background in video, assuming the background frames to be low-rank while the foreground to be spatially sparse. Classic RPCA imposes sparsity of the foreground component using
$\ell _1$
-norm, and minimizes the modeling error via
$\ell _2$
-norm. We show that such assumptions can be too restrictive in practice, which limits the effectiveness of the classic RPCA, especially when processing videos with dynamic background, camera jitter, camouflaged moving object, etc. In this paper, we propose a novel RPCA-based model, called Hyper RPCA, to detect moving objects on the fly. Different from classic RPCA, the proposed Hyper RPCA jointly applies the maximum correntropy criterion (MCC) for the modeling error, and Laplacian scale mixture (LSM) model for foreground objects. Extensive experiments have been conducted, and the results demonstrate that the proposed Hyper RPCA has competitive performance for foreground detection to the state-of-the-art algorithms on several well-known benchmark datasets.
更多查看译文
关键词
Moving object detection,background subtraction,maximum correntropy criterion,laplacian scale mixture model
AI 理解论文
溯源树
样例
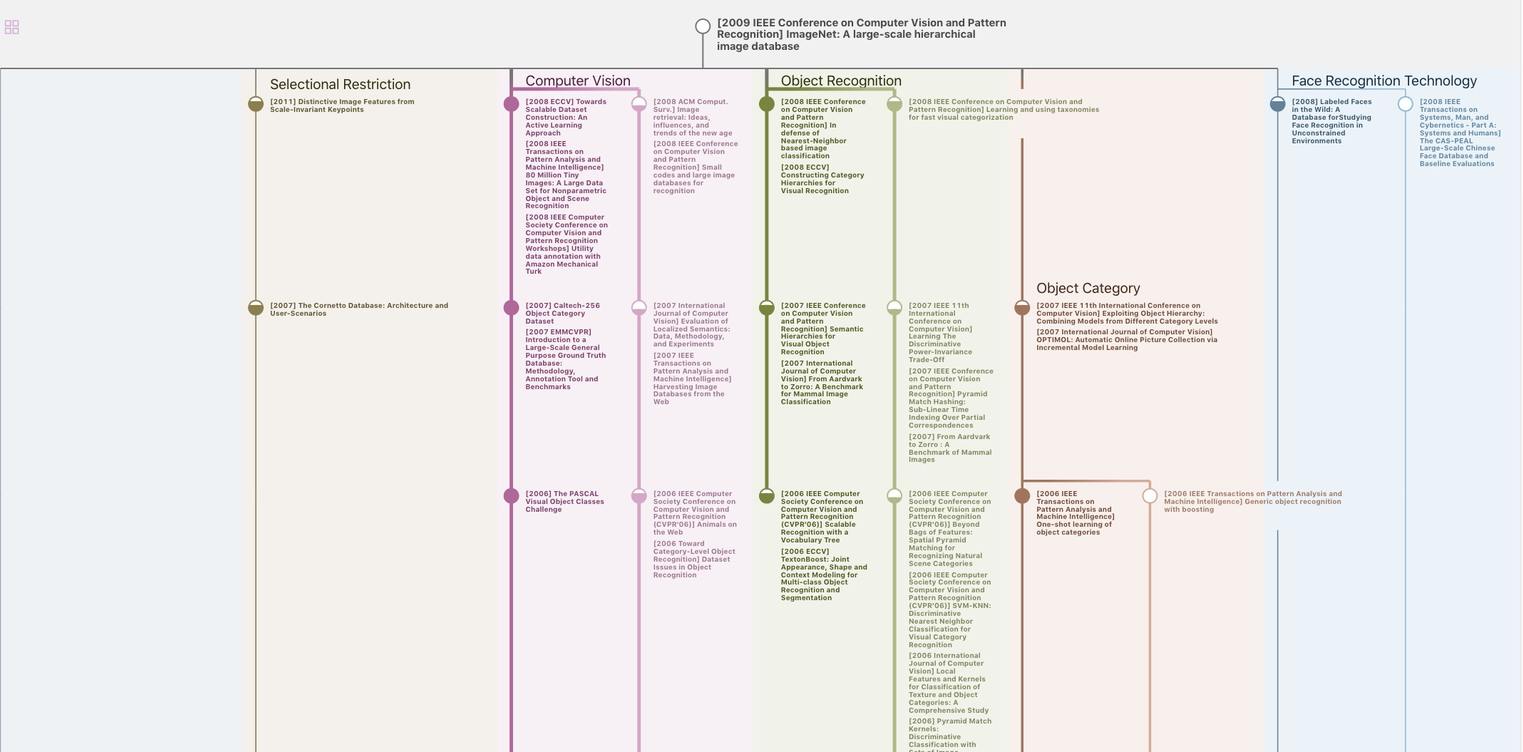
生成溯源树,研究论文发展脉络
Chat Paper
正在生成论文摘要