Low-rank factorization for rank minimization with nonconvex regularizers
COMPUTATIONAL OPTIMIZATION AND APPLICATIONS(2021)
摘要
Rank minimization is of interest in machine learning applications such as recommender systems and robust principal component analysis. Minimizing the convex relaxation to the rank minimization problem, the nuclear norm, is an effective technique to solve the problem with strong performance guarantees. However, nonconvex relaxations have less estimation bias than the nuclear norm and can more accurately reduce the effect of noise on the measurements. We develop efficient algorithms based on iteratively reweighted nuclear norm schemes, while also utilizing the low rank factorization for semidefinite programs put forth by Burer and Monteiro. We prove convergence and computationally show the advantages over convex relaxations and alternating minimization methods. Additionally, the computational complexity of each iteration of our algorithm is on par with other state of the art algorithms, allowing us to quickly find solutions to the rank minimization problem for large matrices.
更多查看译文
关键词
Rank minimization, Matrix completion, Nonconvex regularizers, Semidefinite programming
AI 理解论文
溯源树
样例
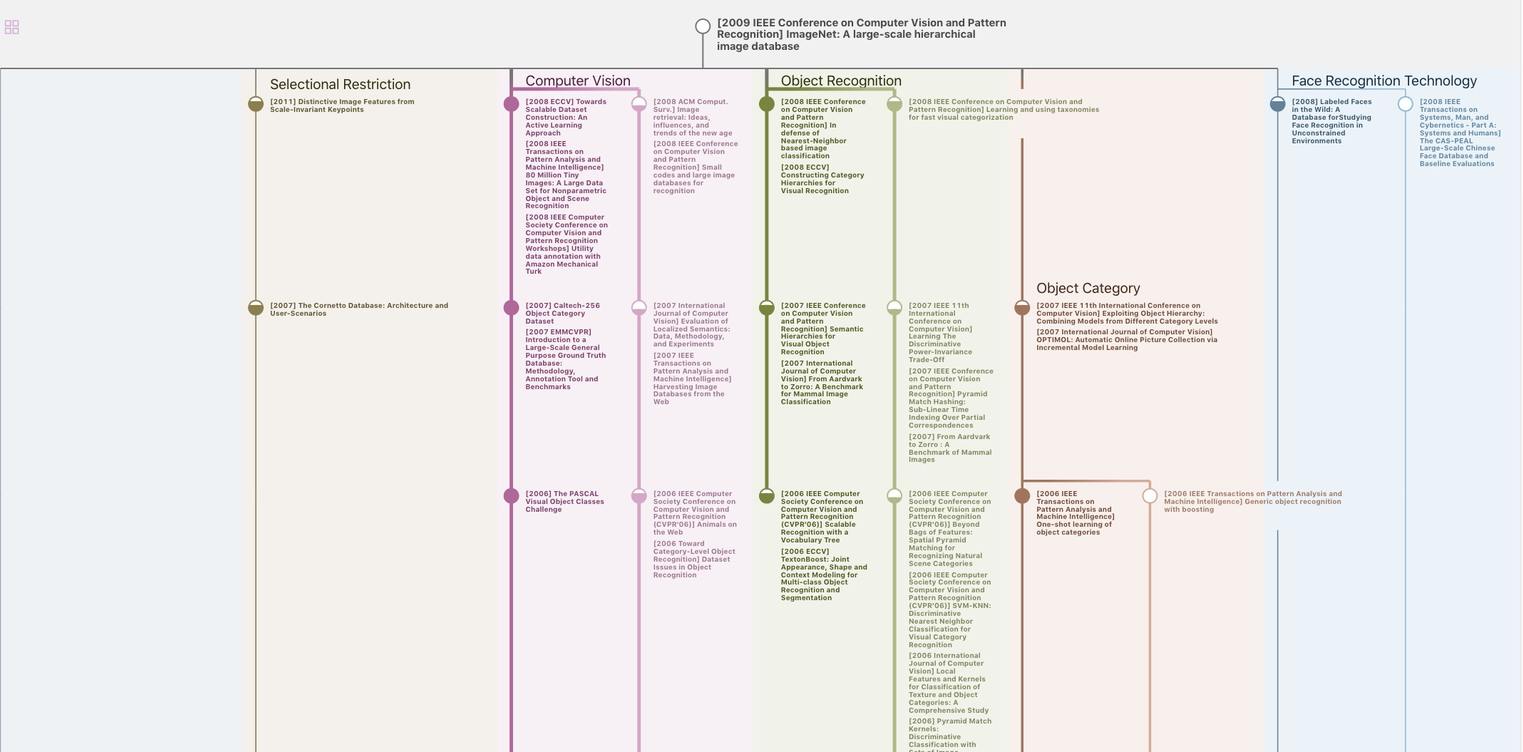
生成溯源树,研究论文发展脉络
Chat Paper
正在生成论文摘要