Structure learning for CTBN's via penalized maximum likelihood methods
CoRR(2020)
Abstract
The continuous-time Bayesian networks (CTBNs) represent a class of stochastic processes, which can be used to model complex phenomena, for instance, they can describe interactions occurring in living processes, in social science models or in medicine. The literature on this topic is usually focused on the case when the dependence structure of a system is known and we are to determine conditional transition intensities (parameters of the network). In the paper, we study the structure learning problem, which is a more challenging task and the existing research on this topic is limited. The approach, which we propose, is based on a penalized likelihood method. We prove that our algorithm, under mild regularity conditions, recognizes the dependence structure of the graph with high probability. We also investigate the properties of the procedure in numerical studies to demonstrate its effectiveness.
MoreTranslated text
AI Read Science
Must-Reading Tree
Example
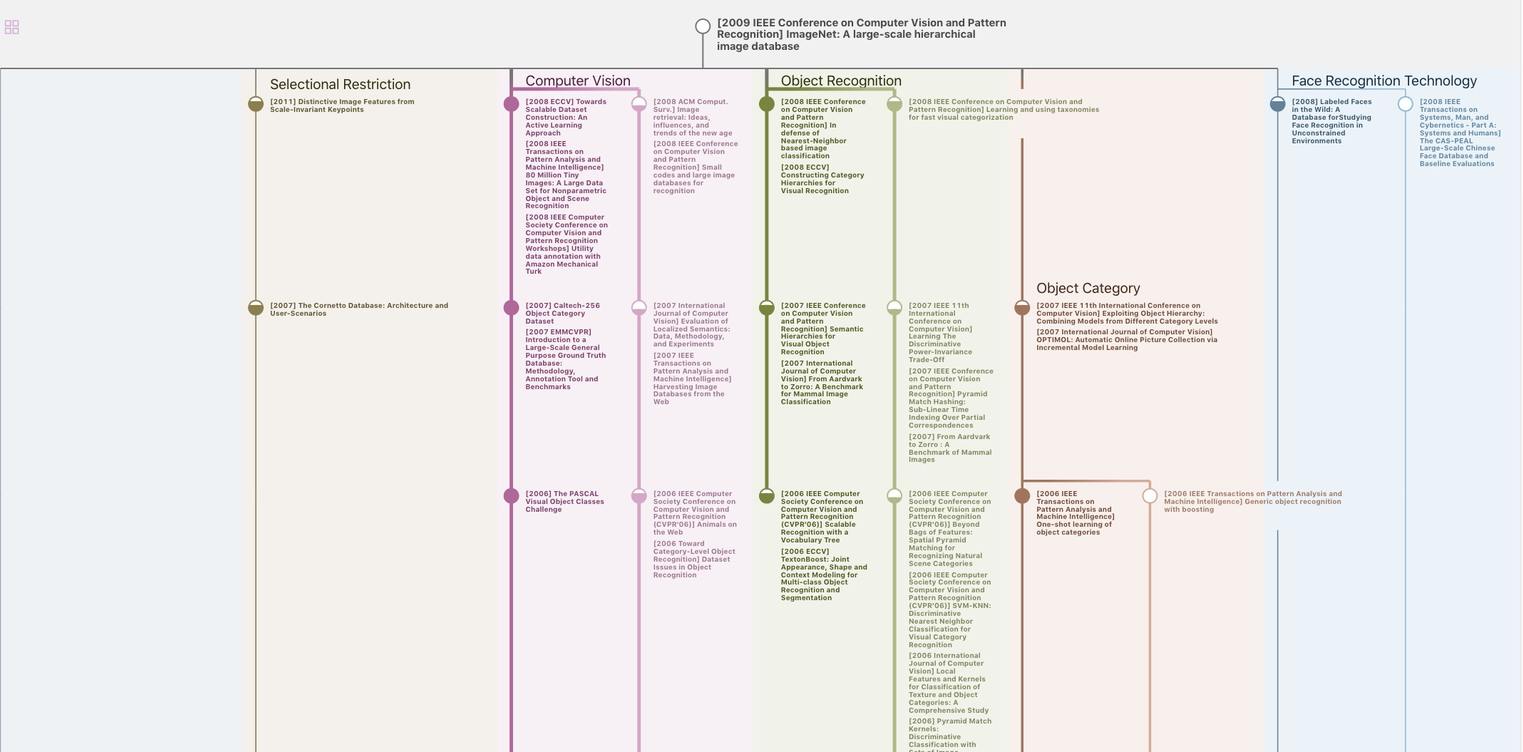
Generate MRT to find the research sequence of this paper
Chat Paper
Summary is being generated by the instructions you defined