Characterizing Motor Control of Mastication With Soft Actor-Critic.
FRONTIERS IN HUMAN NEUROSCIENCE(2020)
摘要
The human masticatory system is a complex functional unit characterized by a multitude of skeletal components, muscles, soft tissues, and teeth. Muscle activation dynamics cannot be directly measured on live human subjects due to ethical, safety, and accessibility limitations. Therefore, estimation of muscle activations and their resultant forces is a longstanding and active area of research. Reinforcement learning (RL) is an adaptive learning strategy which is inspired by the behavioral psychology and enables an agent to learn the dynamics of an unknown system via policy-driven explorations. The RL framework is a well-formulated closed-loop system where high capacity neural networks are trained with the feedback mechanism of rewards to learn relatively complex actuation patterns. In this work, we are building on a deep RL algorithm, known as the Soft Actor-Critic, to learn the inverse dynamics of a simulated masticatory system, i.e., learn the activation patterns that drive the jaw to its desired location. The outcome of the proposed training procedure is a parametric neural model which acts as the brain of the biomechanical system. We demonstrate the model's ability to navigate the feasible three-dimensional (3D) envelope of motion with sub-millimeter accuracies. We also introduce a performance analysis platform consisting of a set of quantitative metrics to assess the functionalities of a given simulated masticatory system. This platform assesses the range of motion, metabolic efficiency, the agility of motion, the symmetry of activations, and the accuracy of reaching the desired target positions. We demonstrate how the model learns more metabolically efficient policies by integrating a force regularization term in the RL reward. We also demonstrate the inverse correlation between the metabolic efficiency of the models and their agility and range of motion. The presented masticatory model and the proposed RL training mechanism are valuable tools for the analysis of mastication and other biomechanical systems. We see this framework's potential in facilitating the functional analyses aspects of surgical treatment planning and predicting the rehabilitation performance in post-operative subjects.
更多查看译文
关键词
mastication modeling,reinforcement learning,soft actor-critic,inverse dynamics,jaw,motor control,musculoskeletal modeling,computational biomechanics
AI 理解论文
溯源树
样例
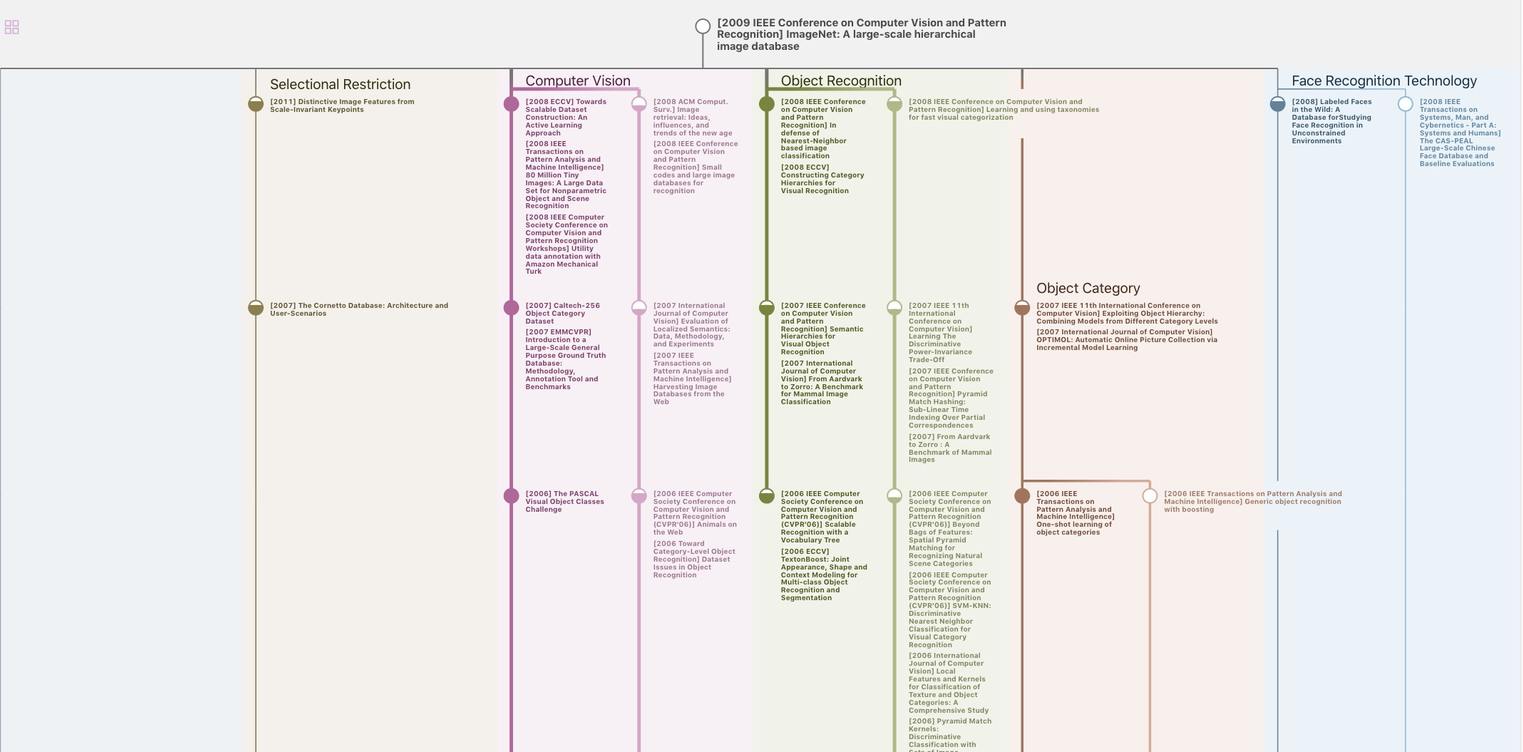
生成溯源树,研究论文发展脉络
Chat Paper
正在生成论文摘要