Self-tunable DBMS Replication with Reinforcement Learning.
DAIS(2020)
摘要
Fault-tolerance is a core feature in distributed database systems, particularly the ones deployed in cloud environments. The dependability of these systems often relies in middleware components that abstract the DBMS logic from the replication itself. The highly configurable nature of these systems makes their throughput very dependent on the correct tuning for a given workload. Given the high complexity involved, machine learning techniques are often considered to guide the tuning process and decompose the relations established between tuning variables. This paper presents a machine learning mechanism based on reinforcement learning that attaches to a hybrid replication middleware connected to a DBMS to dynamically live-tune the configuration of the middleware according to the workload being processed. Along with the vision for the system, we present a study conducted over a prototype of the self-tuned replication middleware, showcasing the achieved performance improvements and showing that we were able to achieve an improvement of 370.99% on some of the considered metrics.
更多查看译文
关键词
reinforcement learning,self-tunable
AI 理解论文
溯源树
样例
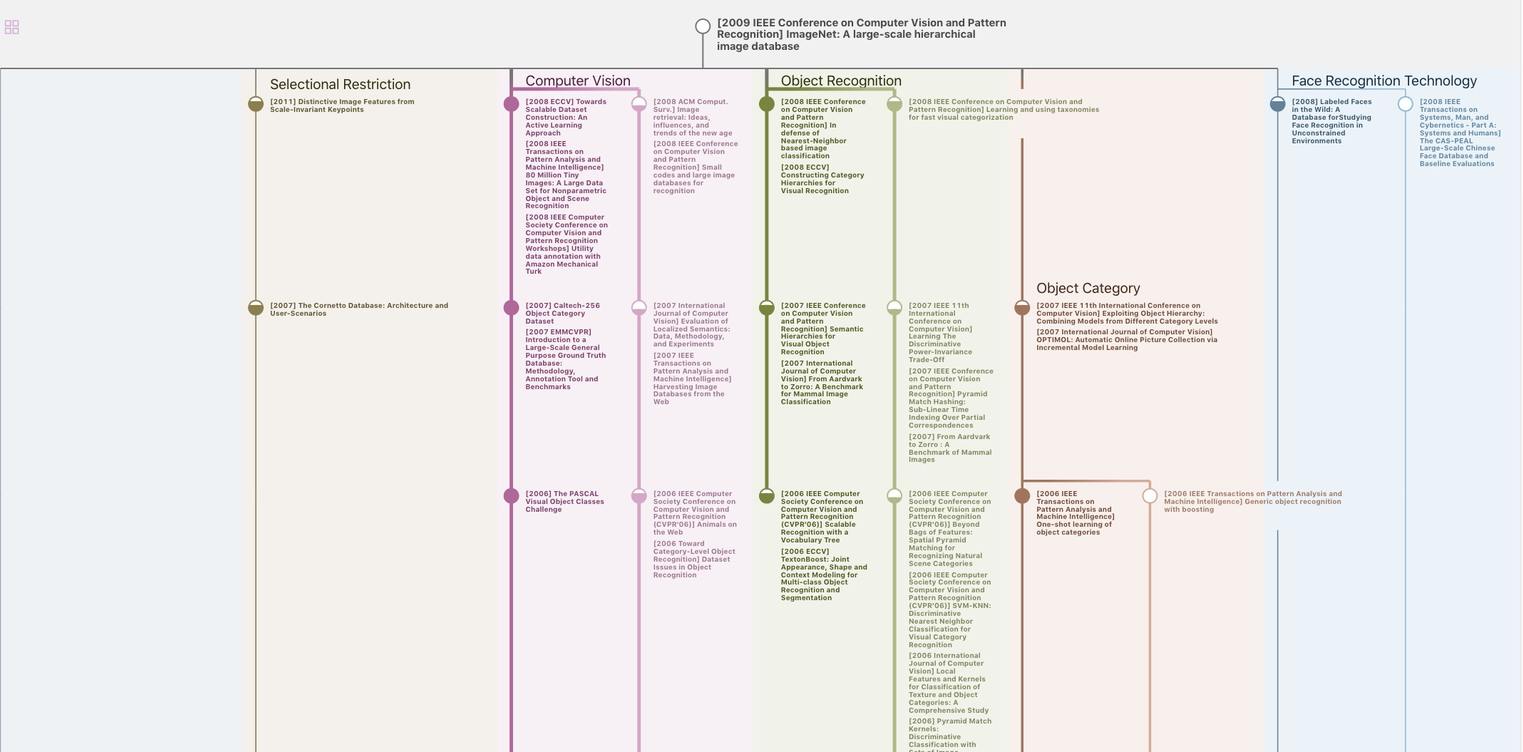
生成溯源树,研究论文发展脉络
Chat Paper
正在生成论文摘要