Statistical Efficiency of Thompson Sampling for Combinatorial Semi-Bandits
CoRR(2020)
摘要
We investigate stochastic combinatorial multi-armed bandit with semi-bandit feedback (CMAB). In CMAB, the question of the existence of an efficient policy with an optimal asymptotic regret (up to a factor poly-logarithmic with the action size) is still open for many families of distributions, including mutually independent outcomes, and more generally the multivariate sub-Gaussian family. We propose to answer the above question for these two families by analyzing variants of the Combinatorial Thompson Sampling policy (CTS). For mutually independent outcomes in $[0,1]$, we propose a tight analysis of CTS using Beta priors. We then look at the more general setting of multivariate sub-Gaussian outcomes and propose a tight analysis of CTS using Gaussian priors. This last result gives us an alternative to the Efficient Sampling for Combinatorial Bandit policy (ESCB), which, although optimal, is not computationally efficient.
更多查看译文
关键词
thompson,statistical efficiency,semi-bandits
AI 理解论文
溯源树
样例
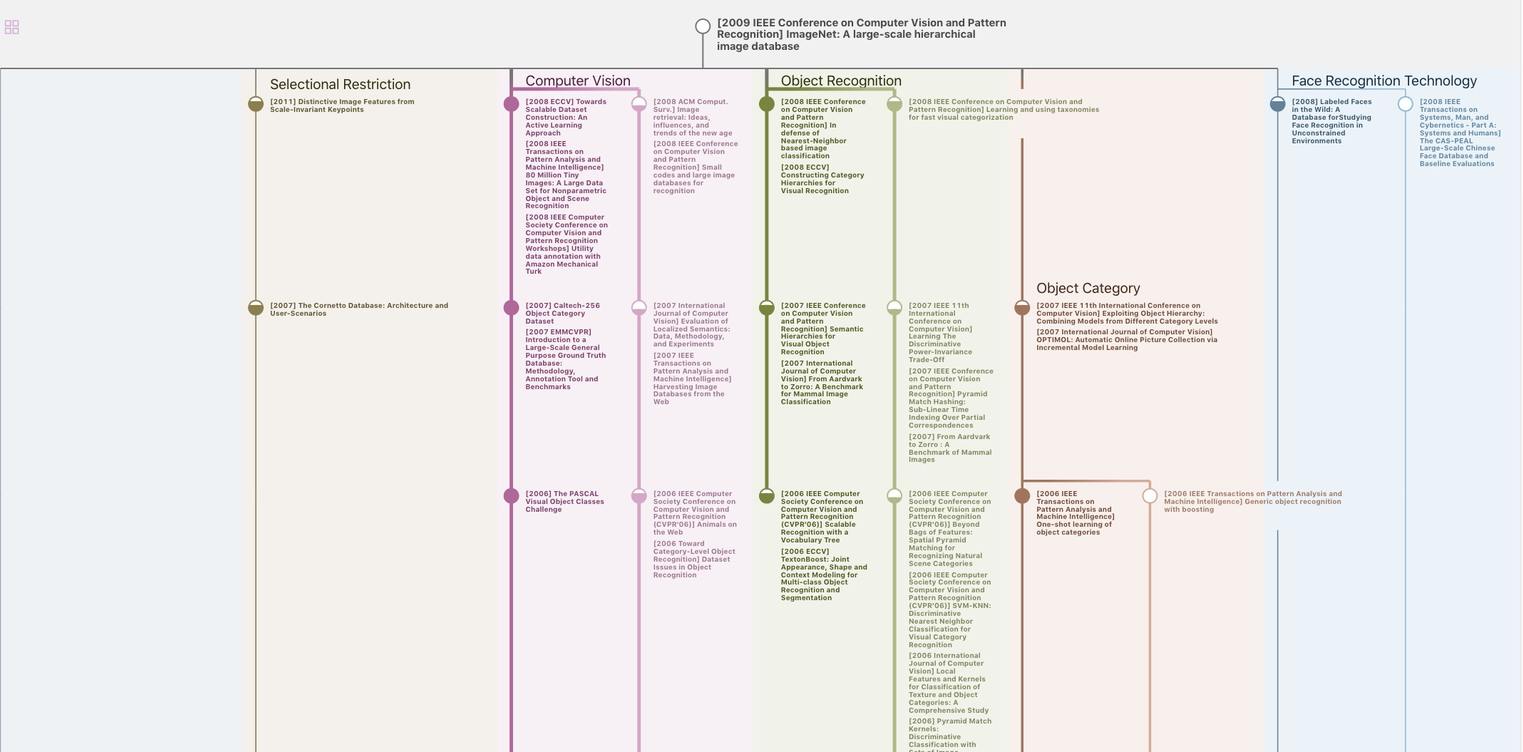
生成溯源树,研究论文发展脉络
Chat Paper
正在生成论文摘要