The Limits to Learning an SIR Process: Granular Forecasting for Covid-19
arxiv(2020)
摘要
A multitude of forecasting efforts have arisen to support management of the ongoing COVID-19 epidemic. These efforts typically rely on a variant of the SIR process and have illustrated that building effective forecasts for an epidemic in its early stages is challenging. This is perhaps surprising since these models rely on a small number of parameters and typically provide an excellent retrospective fit to the evolution of a disease. So motivated, we provide an analysis of the limits to estimating an SIR process. We show that no unbiased estimator can hope to learn this process until observing enough of the epidemic so that one is approximately two-thirds of the way to reaching the peak for new infections. Our analysis provides insight into a regularization strategy that permits effective learning across simultaneously and asynchronously evolving epidemics. This strategy has been used to produce accurate, granular predictions for the COVID-19 epidemic that has found large-scale practical application in a large US state.
更多查看译文
关键词
granular forecasting,sir process,learning
AI 理解论文
溯源树
样例
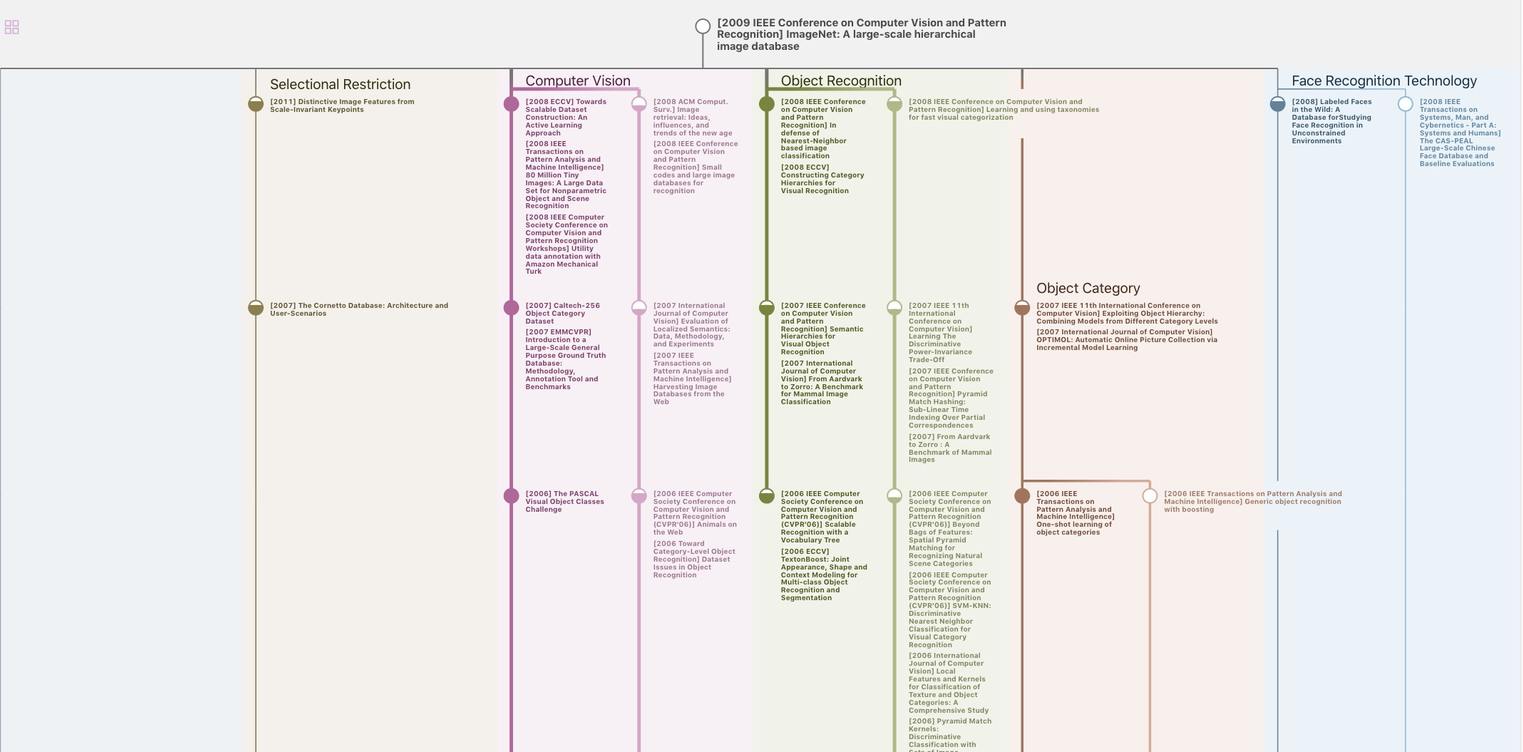
生成溯源树,研究论文发展脉络
Chat Paper
正在生成论文摘要