Toward Certified Robustness of Distance Metric Learning
IEEE TRANSACTIONS ON NEURAL NETWORKS AND LEARNING SYSTEMS(2024)
摘要
Metric learning aims to learn a distance metric such that semantically similar instances are pulled together while dissimilar instances are pushed away. Many existing methods consider maximizing or at least constraining a distance margin in the feature space that separates similar and dissimilar pairs of instances to guarantee their generalization ability. In this article, we advocate imposing an adversarial margin in the input space so as to improve the generalization and robustness of metric learning algorithms. We first show that the adversarial margin, defined as the distance between training instances and their closest adversarial examples in the input space, takes account of both the distance margin in the feature space and the correlation between the metric and triplet constraints. Next, to enhance robustness to instance perturbation, we propose to enlarge the adversarial margin through minimizing a derived novel loss function termed the perturbation loss. The proposed loss can be viewed as a data-dependent regularizer and easily plugged into any existing metric learning methods. Finally, we show that the enlarged margin is beneficial to the generalization ability by using the theoretical technique of algorithmic robustness. Experimental results on 16 datasets demonstrate the superiority of the proposed method over existing state-of-the-art methods in both discrimination accuracy and robustness against possible noise.
更多查看译文
关键词
Measurement,Robustness,Perturbation methods,Training,Learning systems,Artificial neural networks,Correlation,Adversarial perturbation,generalization ability,metric learning,nearest neighbor (NN),robustness
AI 理解论文
溯源树
样例
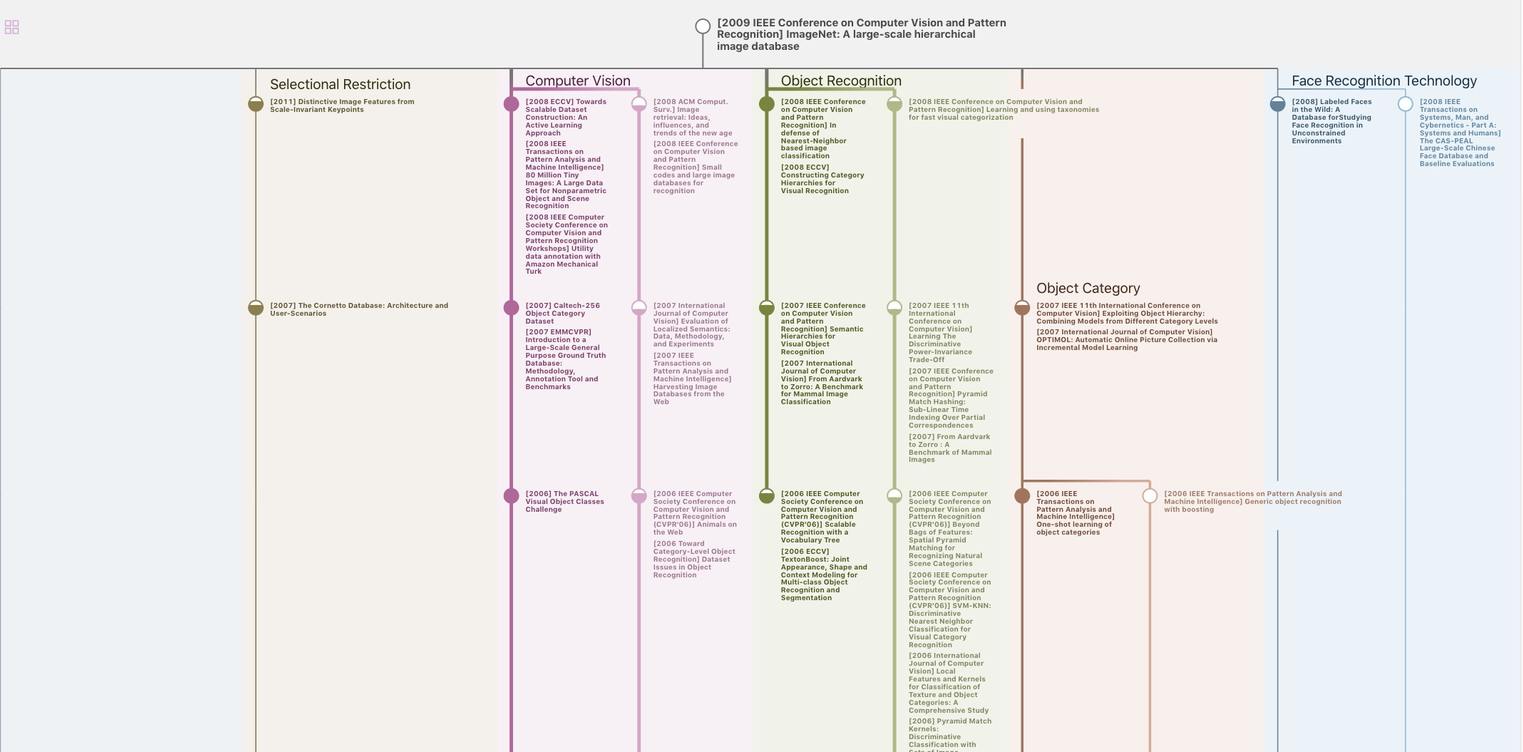
生成溯源树,研究论文发展脉络
Chat Paper
正在生成论文摘要