Estimating semantic structure for the VQA answer space
arxiv(2020)
摘要
Since its appearance, Visual Question Answering (VQA, i.e. answering a question posed over an image), has always been treated as a classification problem over a set of predefined answers. Despite its convenience, this classification approach poorly reflects the semantics of the problem limiting the answering to a choice between independent proposals, without taking into account the similarity between them (e.g. equally penalizing for answering cat or German shepherd instead of dog). We address this issue by proposing (1) two measures of proximity between VQA classes, and (2) a corresponding loss which takes into account the estimated proximity. This significantly improves the generalization of VQA models by reducing their language bias. In particular, we show that our approach is completely model-agnostic since it allows consistent improvements with three different VQA models. Finally, by combining our method with a language bias reduction approach, we report SOTA-level performance on the challenging VQAv2-CP dataset.
更多查看译文
关键词
vqa answer space,semantic structure
AI 理解论文
溯源树
样例
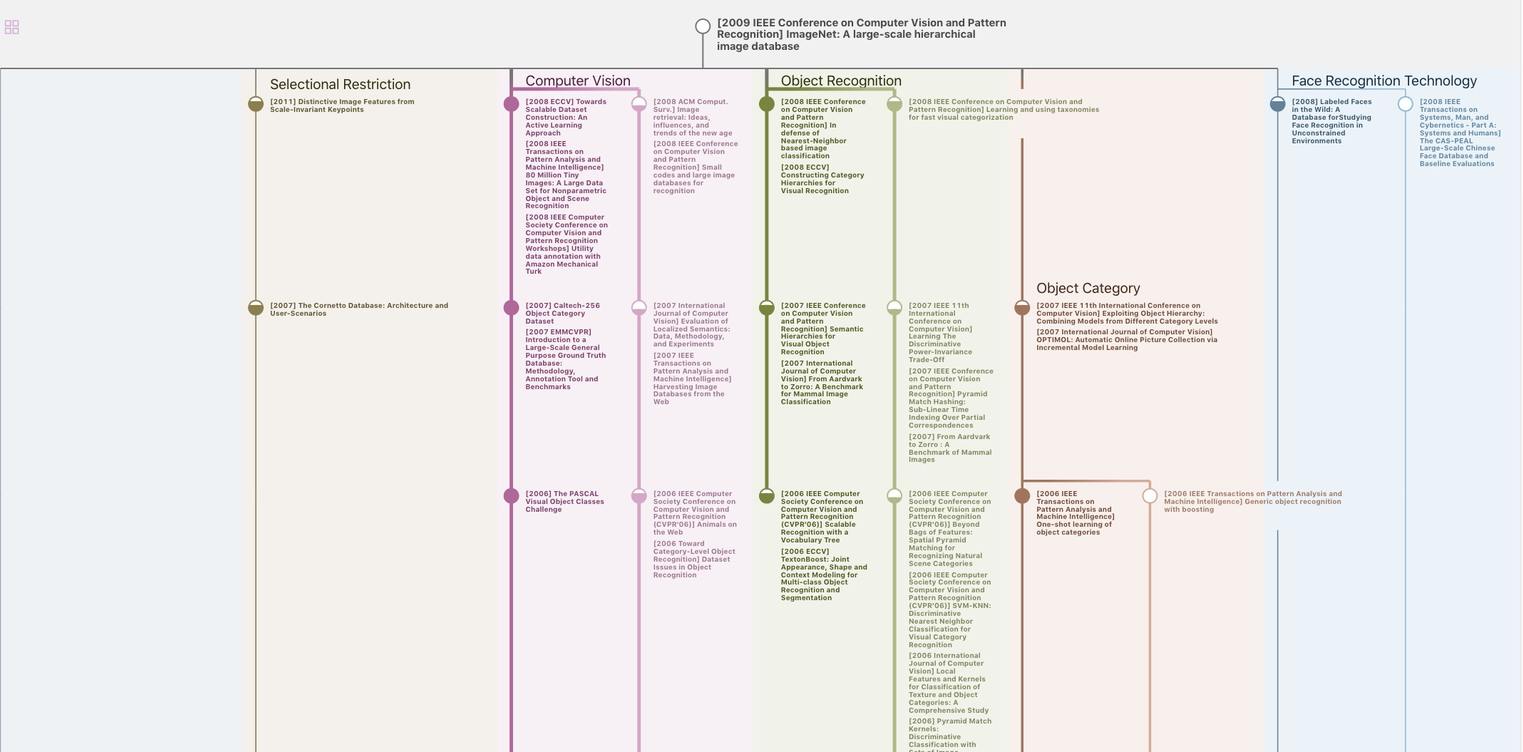
生成溯源树,研究论文发展脉络
Chat Paper
正在生成论文摘要