Dual-Level Semantic Transfer Deep Hashing for Efficient Social Image Retrieval
IEEE Transactions on Circuits and Systems for Video Technology(2021)
摘要
Social network stores and disseminates a tremendous amount of user shared images. Deep hashing is an efficient indexing technique to support large-scale social image retrieval, due to its deep representation capability, fast retrieval speed and low storage cost. Particularly, unsupervised deep hashing has well scalability as it does not require any manually labelled data for training. However, owing to the lacking of label guidance, existing methods suffer from severe semantic shortage when optimizing a large amount of deep neural network parameters. Differently, in this paper, we propose a
Dual-level Semantic Transfer Deep Hashing
(DSTDH) method to alleviate this problem with a unified deep hash learning framework. Our model targets at learning the semantically enhanced deep hash codes by specially exploiting the user-generated tags associated with the social images. Specifically, we design a complementary dual-level semantic transfer mechanism to efficiently discover the potential semantics of tags and seamlessly transfer them into binary hash codes. On the one hand, instance-level semantics are directly preserved into hash codes from the associated tags with adverse noise removing. Besides, an image-concept hypergraph is constructed for indirectly transferring the latent high-order semantic correlations of images and tags into hash codes. Moreover, the hash codes are obtained simultaneously with the deep representation learning by the discrete hash optimization strategy. Extensive experiments on two public social image retrieval datasets validate the superior performance of our method compared with state-of-the-art hashing methods. The source codes of our method can be obtained at
https://github.com/research2020-1/DSTDH
更多查看译文
关键词
Semantics,Optimization,Image retrieval,Visualization,Hash functions,Neural networks,Quantization (signal)
AI 理解论文
溯源树
样例
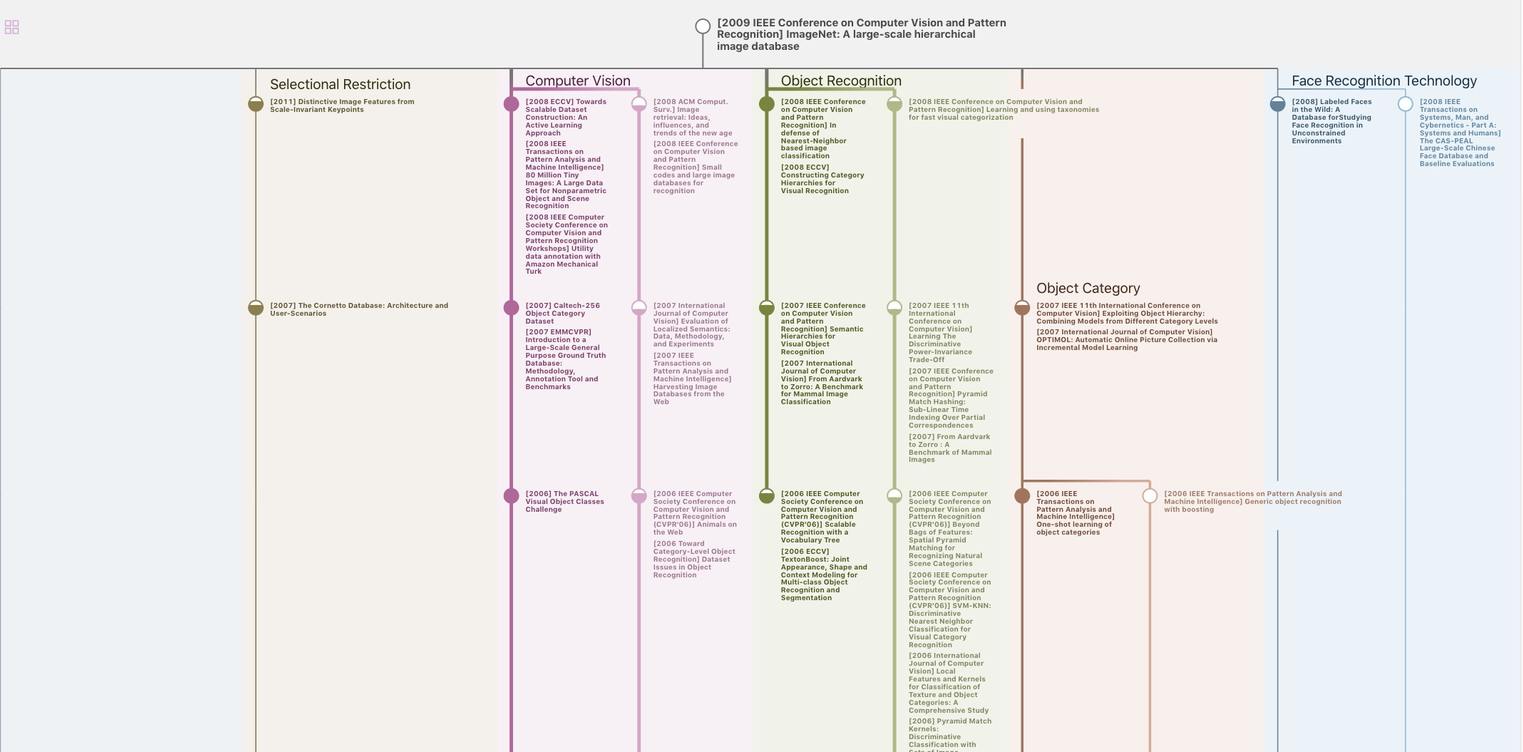
生成溯源树,研究论文发展脉络
Chat Paper
正在生成论文摘要