A Stochastic Subgradient Method for Distributionally Robust Non-convex and Non-smooth Learning
CoRR(2022)
Abstract
We consider a distributionally robust formulation of stochastic optimization problems arising in statistical learning, where robustness is with respect to ambiguity in the underlying data distribution. Our formulation builds on risk-averse optimization techniques and the theory of coherent risk measures. It uses mean–semideviation risk for quantifying uncertainty, allowing us to compute solutions that are robust against perturbations in the population data distribution. We consider a broad class of generalized differentiable loss functions that can be non-convex and non-smooth, involving upward and downward cusps, and we develop an efficient stochastic subgradient method for distributionally robust problems with such functions. We prove that it converges to a point satisfying the optimality conditions. To our knowledge, this is the first method with rigorous convergence guarantees in the context of generalized differentiable non-convex and non-smooth distributionally robust stochastic optimization. Our method allows for the control of the desired level of robustness with little extra computational cost compared to population risk minimization with stochastic gradient methods. We also illustrate the performance of our algorithm on real datasets arising in convex and non-convex supervised learning problems.
MoreTranslated text
Key words
Robust learning, Risk measures, Stochastic subgradient method, Non-smooth optimization, Composition optimization, 90C15, 90C48
AI Read Science
Must-Reading Tree
Example
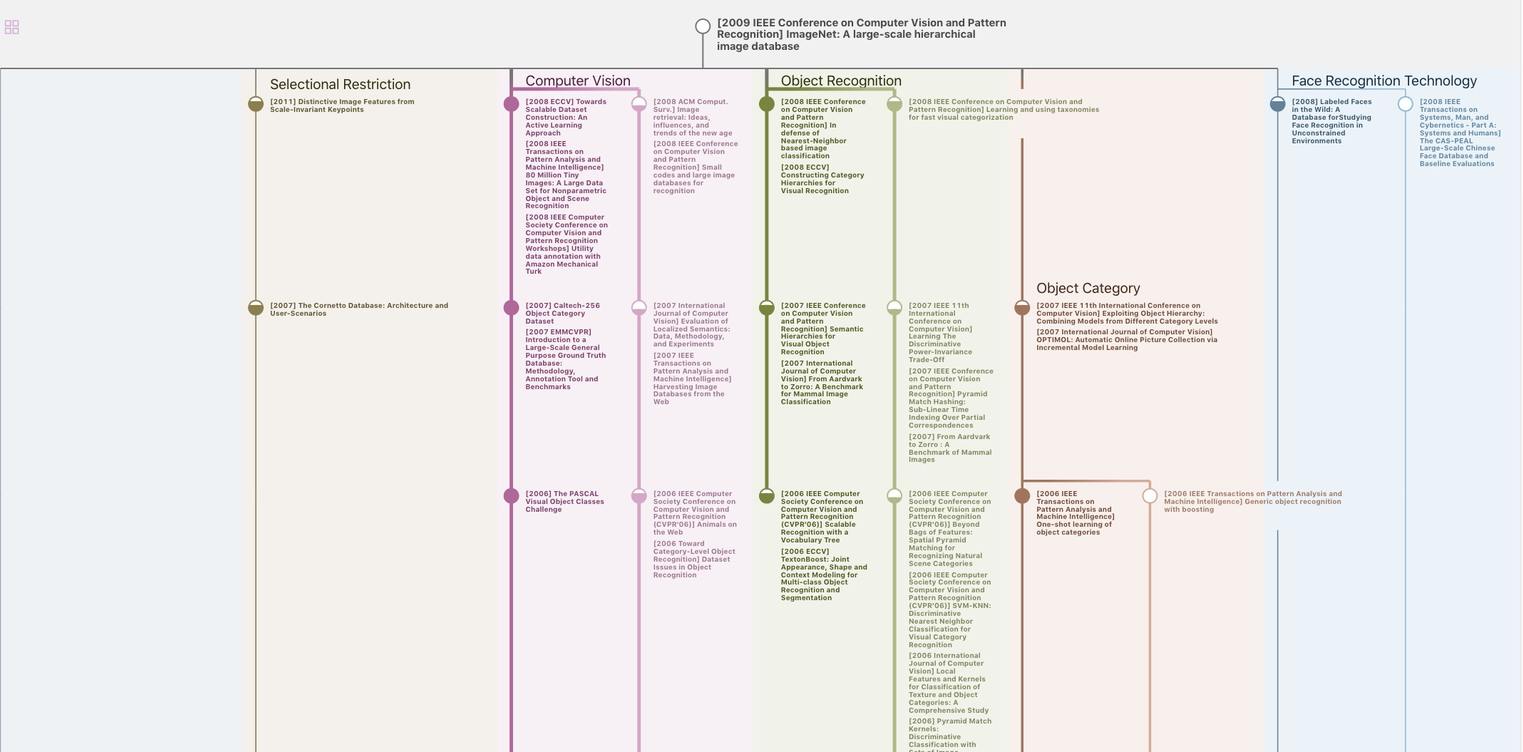
Generate MRT to find the research sequence of this paper
Chat Paper
Summary is being generated by the instructions you defined