Deep Support Vector Machines For The Identification Of Stress Condition From Electrodermal Activity
INTERNATIONAL JOURNAL OF NEURAL SYSTEMS(2020)
摘要
Early detection of stress condition is beneficial to prevent long-term mental illness like depression and anxiety. This paper introduces an accurate identification of stress/calm condition from electrodermal activity (EDA) signals. The acquisition of EDA signals from a commercial wearable as well as their storage and processing are presented. Several time-domain, frequency-domain and morphological features are extracted over the skin conductance response of the EDA signals. Afterwards, a classification is undergone by using several classical support vector machines (SVMs) and deep support vector machines (D-SVMs). In addition, several binary classifiers are also compared with SVMs in the stress/calm identification task. Moreover, a series of video clips evoking calm and stress conditions have been viewed by 147 volunteers in order to validate the classification results. The highest F1-score obtained for SVMs and D-SVMs are 83% and 92%, respectively. These results demonstrate that not only classical SVMs are appropriate for classification of biomarker signals, but D-SVMs are very competitive in comparison to other classification techniques. In addition, the results have enabled drawing useful considerations for the future use of SVMs and D-SVMs in the specific case of stress/calm identification.
更多查看译文
关键词
Electrodermal activity, support vector machines, deep support vector machines, calm, stress
AI 理解论文
溯源树
样例
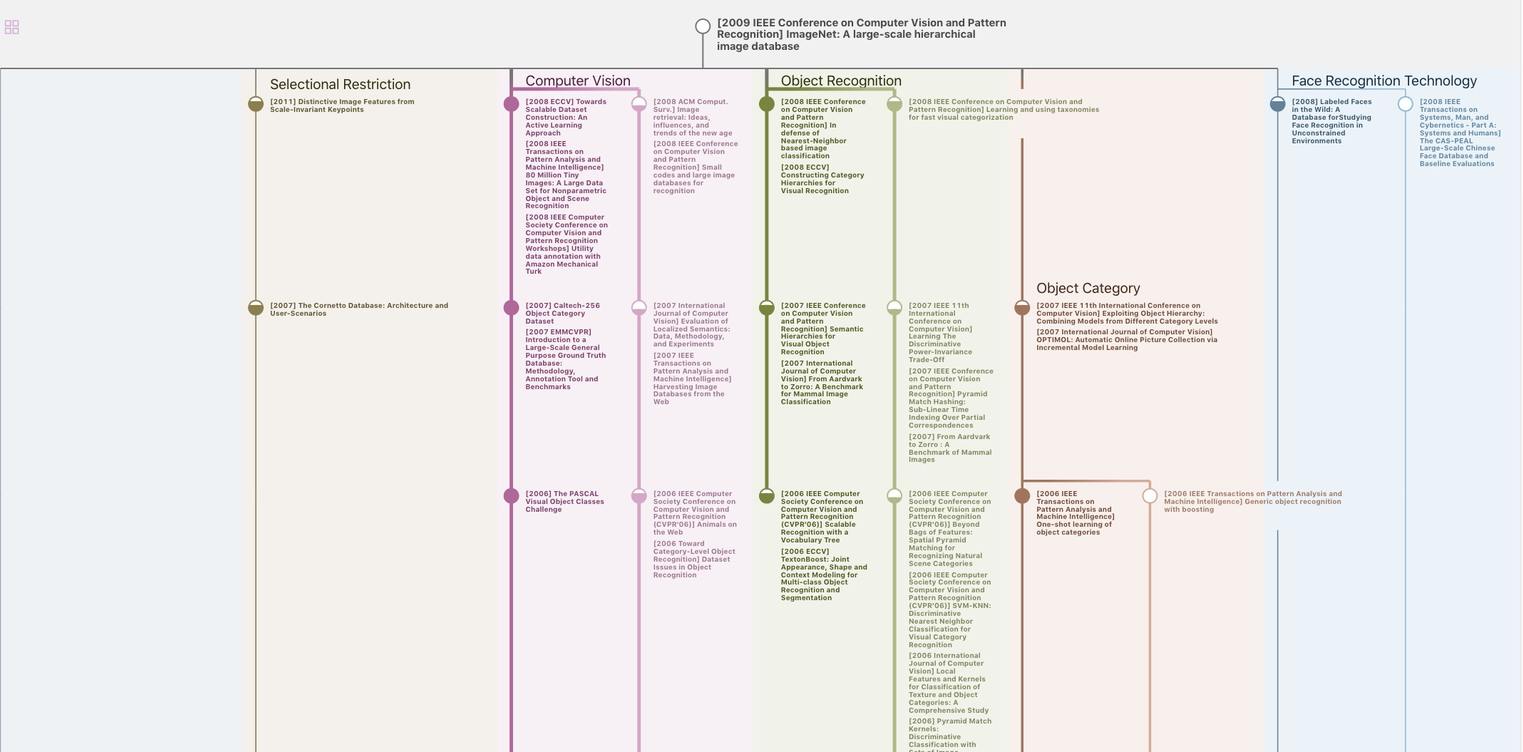
生成溯源树,研究论文发展脉络
Chat Paper
正在生成论文摘要