Region Proposal Network with Graph Prior and IoU-Balance Loss for Landmark Detection in 3D Ultrasound
2020 IEEE 17TH INTERNATIONAL SYMPOSIUM ON BIOMEDICAL IMAGING (ISBI 2020)(2020)
摘要
3D ultrasound (US) can facilitate detailed prenatal examinations for fetal growth monitoring. To analyze a 3D US volume, it is fundamental to identify anatomical landmarks of the evaluated organs accurately. Typical deep learning methods usually regress the coordinates directly or involve heatmap-matching. However, these methods struggle to deal with volumes with large sizes and the highly-varying positions and orientations of fetuses. In this work, we exploit an object detection framework to detect landmarks in 3D fetal facial US volumes. By regressing multiple parameters of the landmark-centered bounding box (B-box) with a strict criteria, the proposed model is able to pinpoint the exact location of the targeted landmarks. Specifically, the model uses a 3D region proposal network (RPN) to generate 3D candidate regions, followed by several 3D classification branches to select the best candidate. It also adopts an IoU-balance loss to improve communications between branches that benefits the learning process. Furthermore, it leverages a distance-based graph prior to regularize the training and helps to reduce false positive predictions. The performance of the proposed framework is evaluated on a 3D US dataset to detect five key fetal facial landmarks. Results showed the proposed method outperforms some of the state-of-the-art methods in efficacy and efficiency.
更多查看译文
关键词
3D ultrasound, Landmark detection, Region proposal network, Fetal face, Prior knowledge
AI 理解论文
溯源树
样例
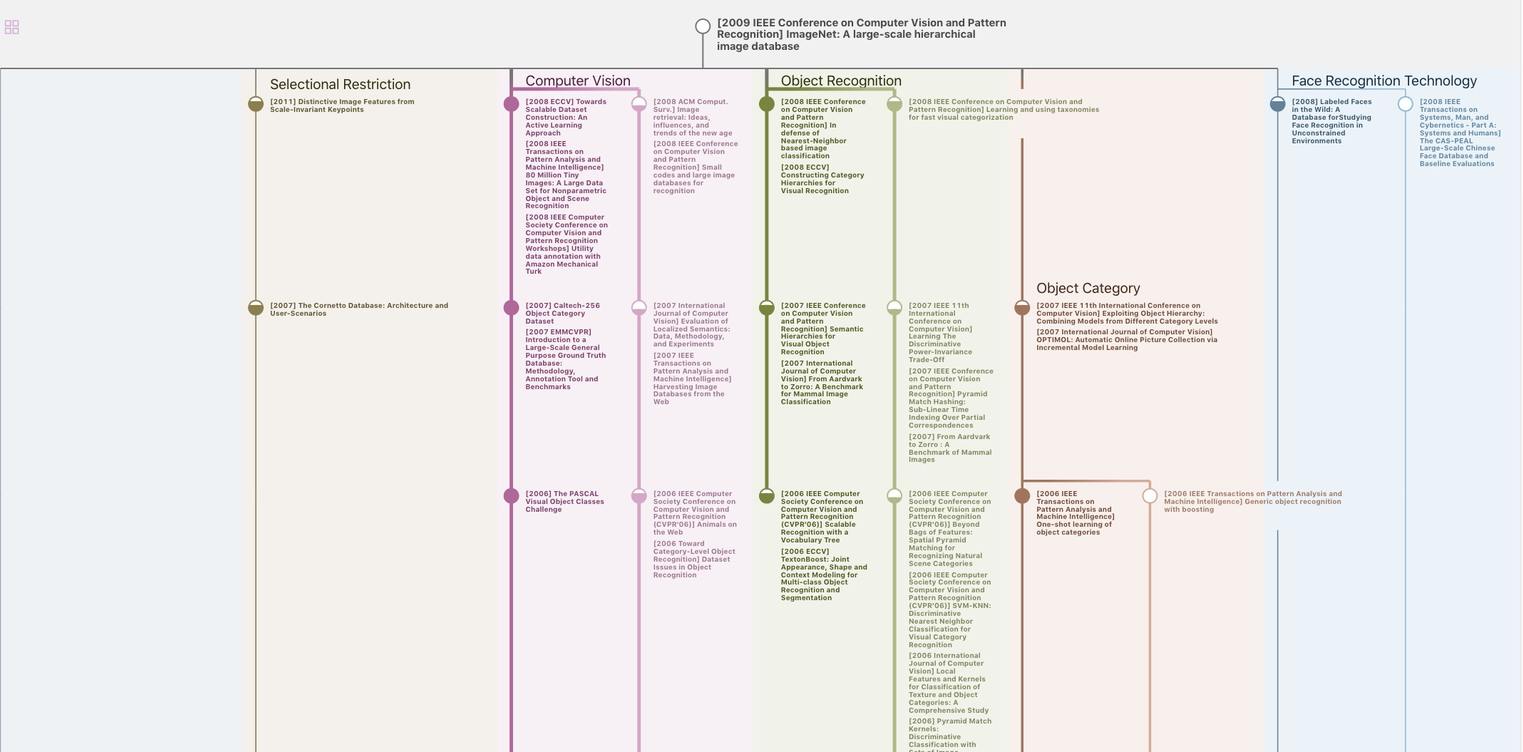
生成溯源树,研究论文发展脉络
Chat Paper
正在生成论文摘要