Supervised Learning For Segmenting Open Boundaries In Medical Images
2020 IEEE 17TH INTERNATIONAL SYMPOSIUM ON BIOMEDICAL IMAGING (ISBI 2020)(2020)
摘要
Image segmentation is one of the most important building blocks in many medical imaging applications. Often, it is the first step in any artificial intelligence (AI) assisted diagnosis system.Most convolutional neural network image-to-image segmentation algorithms compute a binary mask segmentation and extract the object boundary as the edge of the binary mask always leading to a closed boundary.In this paper, we present a novel image-to-image segmentation algorithm that learns open boundaries. The object delineation is directly learnt by training a U-Net like network on a distance map representation of the boundary without any constraints on its shape or topology. We validate the proposed approach on the segmentation of the left atrium in intra-cardiac echocardiography images. For this application, it is important to produce segmentations only where a strong evidence of the anatomy exists.To our knowledge, this is the first work to train a U-Net on a distance map ground truth representation for open boundary segmentation.
更多查看译文
关键词
supervised learning,segmenting open boundaries,medical images,building blocks,medical imaging applications,artificial intelligence assisted diagnosis system,convolutional neural network image-to-image segmentation algorithms,binary mask segmentation,object boundary,closed boundary,image-to-image segmentation algorithm,intra-cardiac echocardiography images,open boundary segmentation
AI 理解论文
溯源树
样例
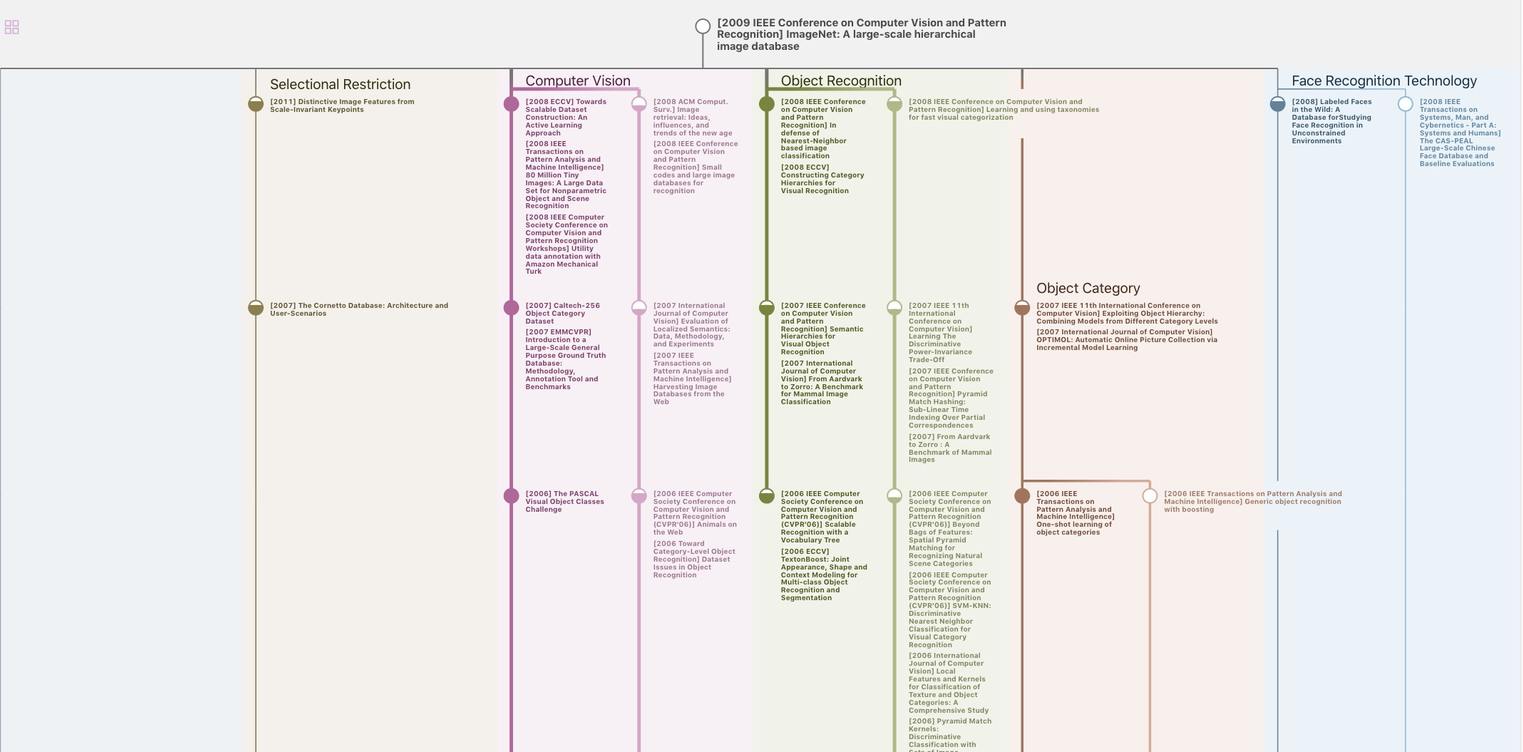
生成溯源树,研究论文发展脉络
Chat Paper
正在生成论文摘要