Motion Prediction using Trajectory Sets and Self-Driving Domain Knowledge
arxiv(2020)
摘要
Predicting the future motion of vehicles has been studied using various techniques, including stochastic policies, generative models, and regression. Recent work has shown that classification over a trajectory set, which approximates possible motions, achieves state-of-the-art performance and avoids issues like mode collapse. However, map information and the physical relationships between nearby trajectories is not fully exploited in this formulation. We build on classification-based approaches to motion prediction by adding an auxiliary loss that penalizes off-road predictions. This auxiliary loss can easily be \emph{pretrained} using only map information (e.g., off-road area), which significantly improves performance on small datasets. We also investigate weighted cross-entropy losses to capture spatial-temporal relationships among trajectories. Our final contribution is a detailed comparison of classification and ordinal regression on two public self-driving datasets.
更多查看译文
关键词
trajectory sets,domain knowledge,motion,prediction,self-driving
AI 理解论文
溯源树
样例
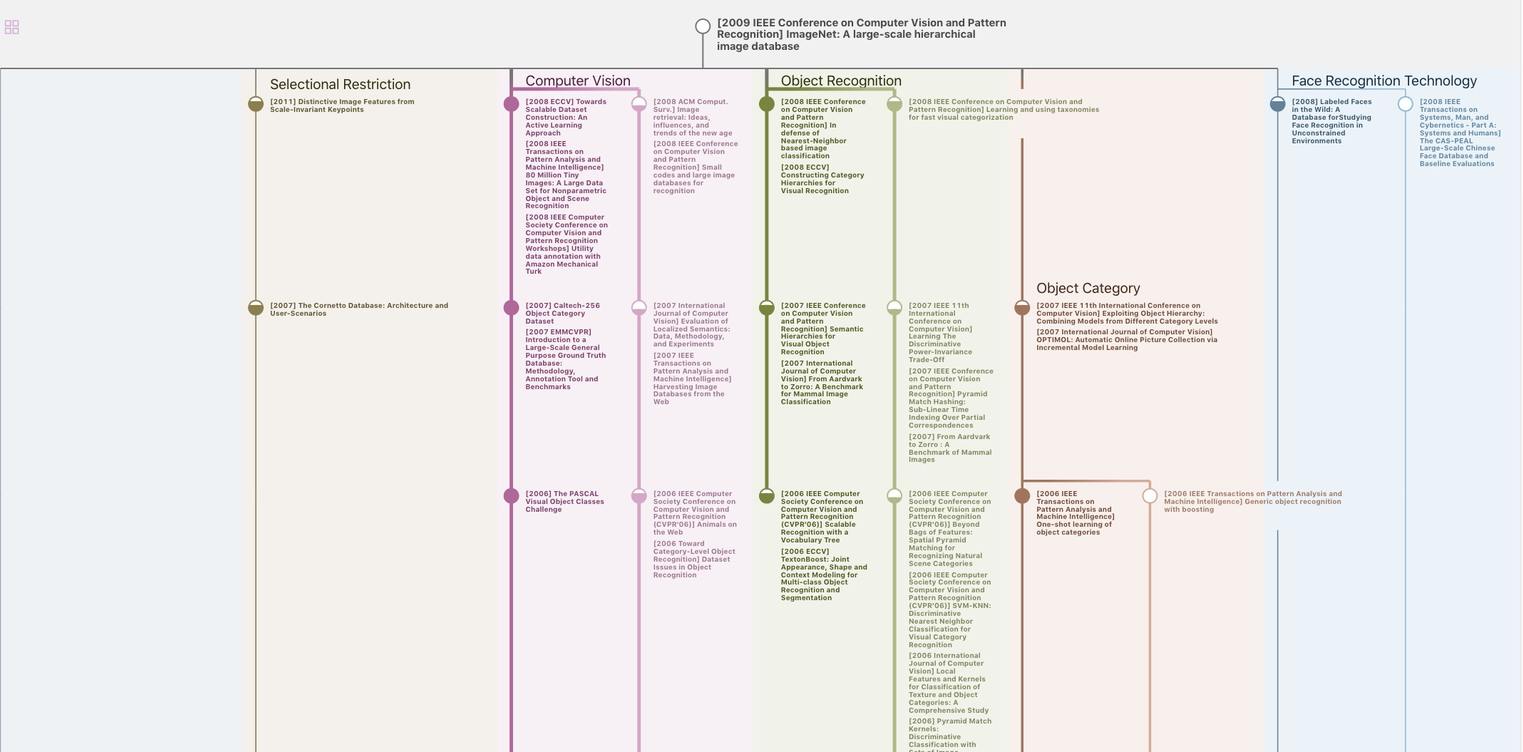
生成溯源树,研究论文发展脉络
Chat Paper
正在生成论文摘要