Bayesian Hidden Physics Models: Uncertainty Quantification for Discovery of Nonlinear Partial Differential Operators from Data
arXiv (Cornell University)(2020)
Abstract
What do data tell us about physics-and what don't they tell us? There has been a surge of interest in using machine learning models to discover governing physical laws such as differential equations from data, but current methods lack uncertainty quantification to communicate their credibility. This work addresses this shortcoming from a Bayesian perspective. We introduce a novel model comprising "leaf" modules that learn to represent distinct experiments' spatiotemporal functional data as neural networks and a single "root" module that expresses a nonparametric distribution over their governing nonlinear differential operator as a Gaussian process. Automatic differentiation is used to compute the required partial derivatives from the leaf functions as inputs to the root. Our approach quantifies the reliability of the learned physics in terms of a posterior distribution over operators and propagates this uncertainty to solutions of novel initial-boundary value problem instances. Numerical experiments demonstrate the method on several nonlinear PDEs.
MoreTranslated text
Key words
nonlinear partial differential operators,uncertainty quantification,models,discovery
AI Read Science
Must-Reading Tree
Example
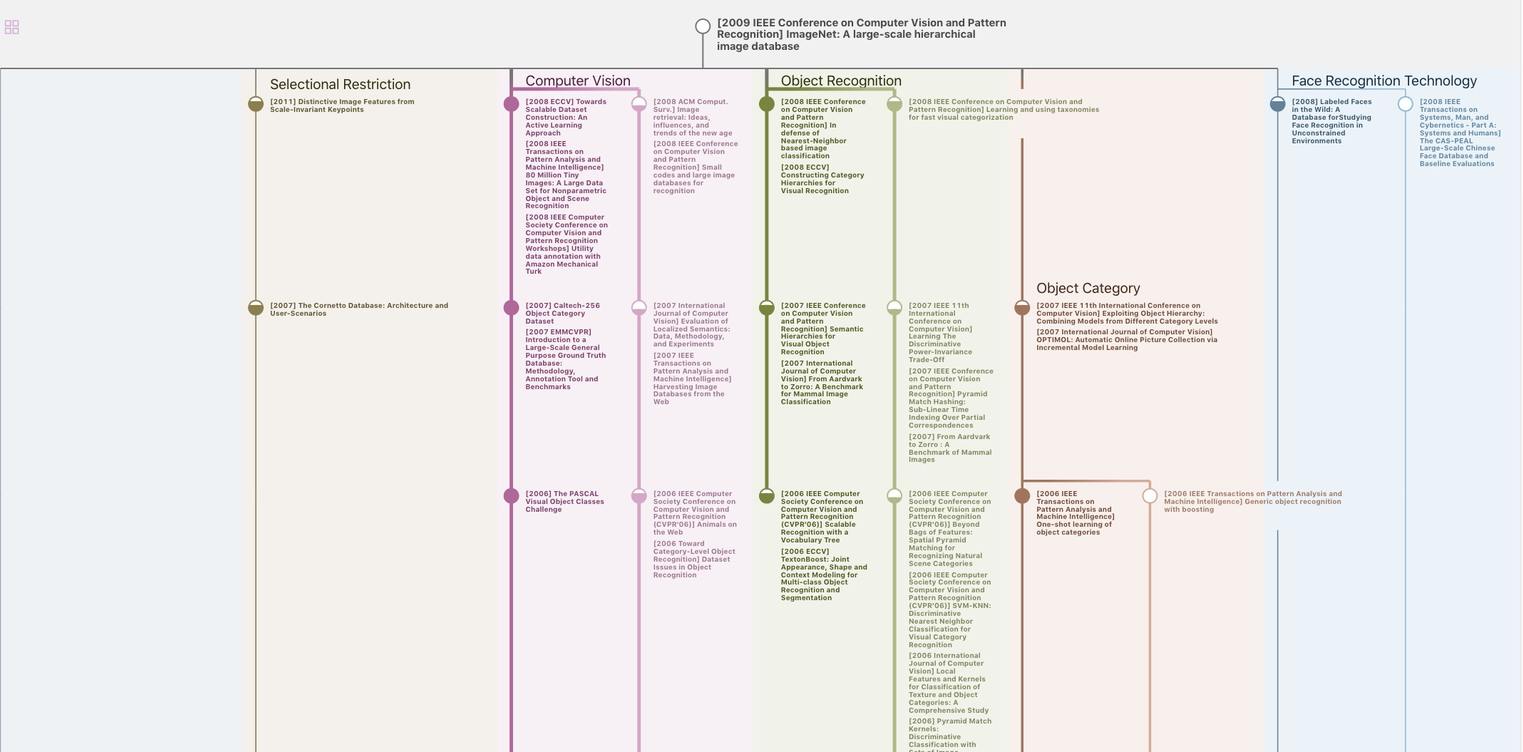
Generate MRT to find the research sequence of this paper
Chat Paper
Summary is being generated by the instructions you defined