Combinatorial Black-Box Optimization with Expert Advice
KDD '20: The 26th ACM SIGKDD Conference on Knowledge Discovery and Data Mining Virtual Event CA USA July, 2020(2020)
摘要
We consider the problem of black-box function optimization over the Boolean hypercube. Despite the vast literature on black-box function optimization over continuous domains, not much attention has been paid to learning models for optimization over combinatorial domains until recently. However, the computational complexity of the recently devised algorithms are prohibitive even for moderate numbers of variables; drawing one sample using the existing algorithms is more expensive than a function evaluation for many black-box functions of interest. To address this problem, we propose a computationally efficient model learning algorithm based on multilinear polynomials and exponential weight updates. In the proposed algorithm, we alternate between simulated annealing with respect to the current polynomial representation and updating the weights using monomial experts' advice. Numerical experiments on various datasets in both unconstrained and sum-constrained Boolean optimization indicate the competitive performance of the proposed algorithm, while improving the computational time up to several orders of magnitude compared to state-of-the-art algorithms in the literature.
更多查看译文
关键词
optimization,expert advice,black-box
AI 理解论文
溯源树
样例
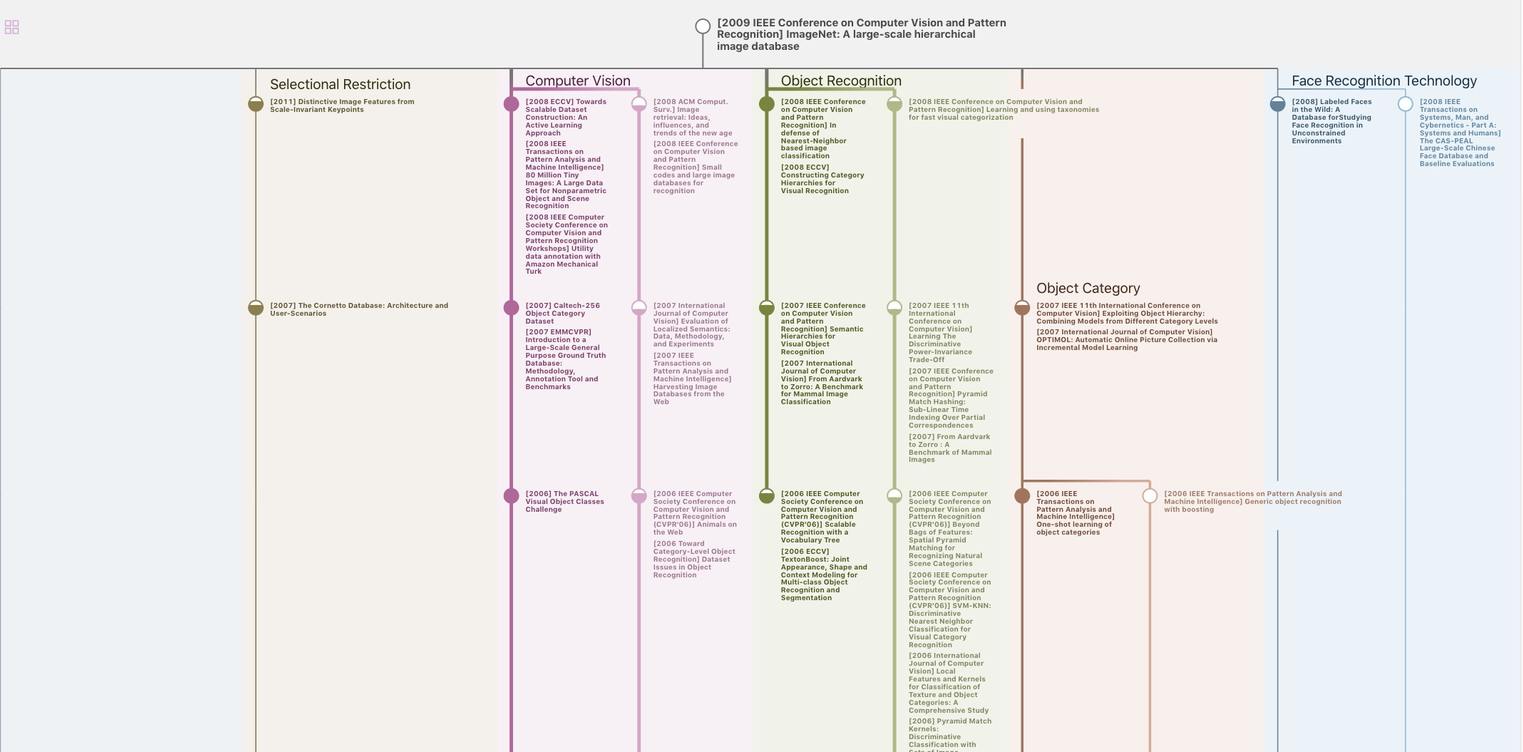
生成溯源树,研究论文发展脉络
Chat Paper
正在生成论文摘要