Self-Supervised Dynamic Networks for Covariate Shift Robustness
arxiv(2020)
摘要
As supervised learning still dominates most AI applications, test-time performance is often unexpected. Specifically, a shift of the input covariates, caused by typical nuisances like background-noise, illumination variations or transcription errors, can lead to a significant decrease in prediction accuracy. Recently, it was shown that incorporating self-supervision can significantly improve covariate shift robustness. In this work, we propose Self-Supervised Dynamic Networks (SSDN): an input-dependent mechanism, inspired by dynamic networks, that allows a self-supervised network to predict the weights of the main network, and thus directly handle covariate shifts at test-time. We present the conceptual and empirical advantages of the proposed method on the problem of image classification under different covariate shifts, and show that it significantly outperforms comparable methods.
更多查看译文
关键词
covariate shift robustness,dynamic networks,self-supervised
AI 理解论文
溯源树
样例
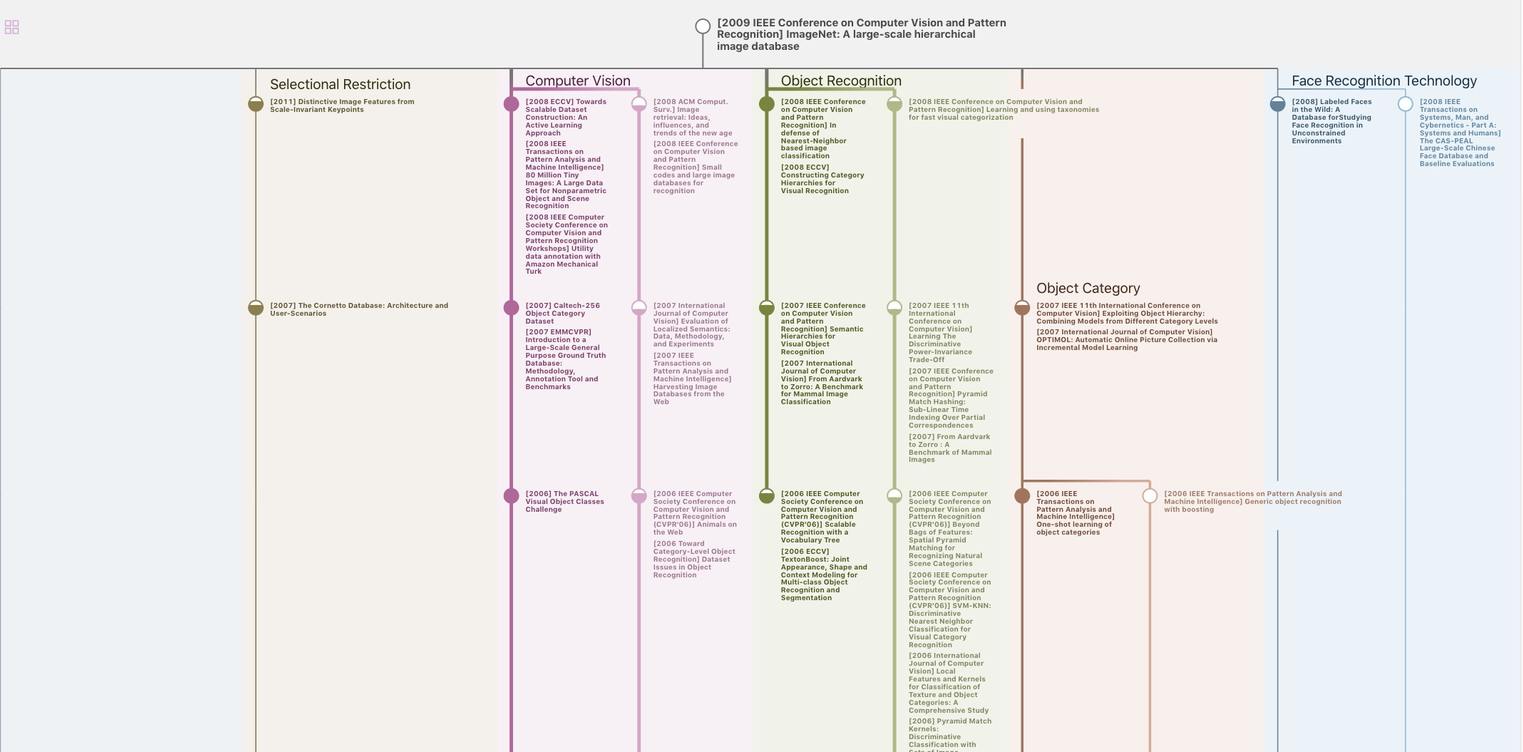
生成溯源树,研究论文发展脉络
Chat Paper
正在生成论文摘要