UCLID-Net: Single View Reconstruction in Object Space
NIPS 2020(2020)
摘要
Most state-of-the-art deep geometric learning single-view reconstruction approaches rely on encoder-decoder architectures that output either shape parametrizations or implicit representations. However, these representations rarely preserve the Euclidean structure of the 3D space objects exist in. In this paper, we show that building a geometry preserving 3-dimensional latent space helps the network concurrently learn global shape regularities and local reasoning in the object coordinate space and, as a result, boosts performance. We demonstrate both on ShapeNet synthetic images, which are often used for benchmarking purposes, and on real-world images that our approach outperforms state-of-the-art ones. Furthermore, the single-view pipeline naturally extends to multi-view reconstruction, which we also show.
更多查看译文
关键词
single view reconstruction,uclid-net
AI 理解论文
溯源树
样例
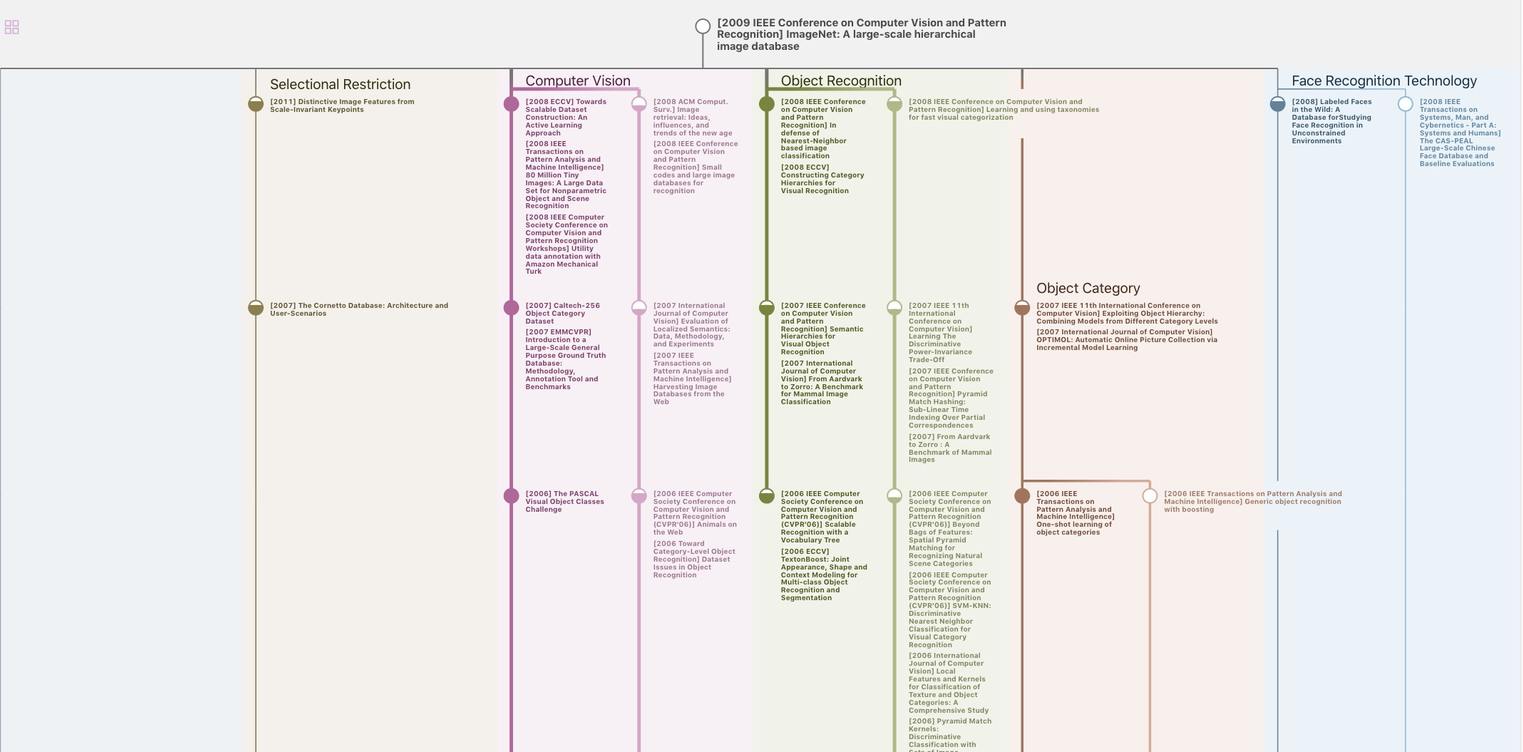
生成溯源树,研究论文发展脉络
Chat Paper
正在生成论文摘要