On the Robustness of Causal Discovery with Additive Noise Models on Discrete Data
2020 Data Compression Conference (DCC)(2020)
摘要
The Additive Noise Models (ANMs) framework for causal discovery has gained much attention due to its strong theoretical guarantees, as well as superior empirical performances on a wide range of real-world data. For observational data, however, quantization (or discretization) is often an inevitable preprocessing step depending on measurement precision requirements. It is thus crucial to understand how sensitive the ANMs are with respect to quantization. In this work, we study the robustness of ANMs framework (via both uniform and Lloyd's quantizers), with a particular focus on its discrete variants. Instead of applying small perturbations on data, we adopt a more aggressive approach by empirically evaluating the methods over various discretization levels that are potentially much smaller than the support of the original data. Surprisingly, the discrete variants are outperformed by the original ANM method developed for continuous data, which inspired us to design a simple yet e ective discrete method that is relatively robust compared with existing discrete methods on various synthetic and real-world data.
更多查看译文
关键词
additive noise models framework,Lloyds quantizers,discrete data,discrete methods,continuous data,original ANM method,discretization levels,discrete variants,ANM framework,measurement precision requirements,inevitable preprocessing step,observational data,real-world data,superior empirical performances,strong theoretical guarantees,causal discovery
AI 理解论文
溯源树
样例
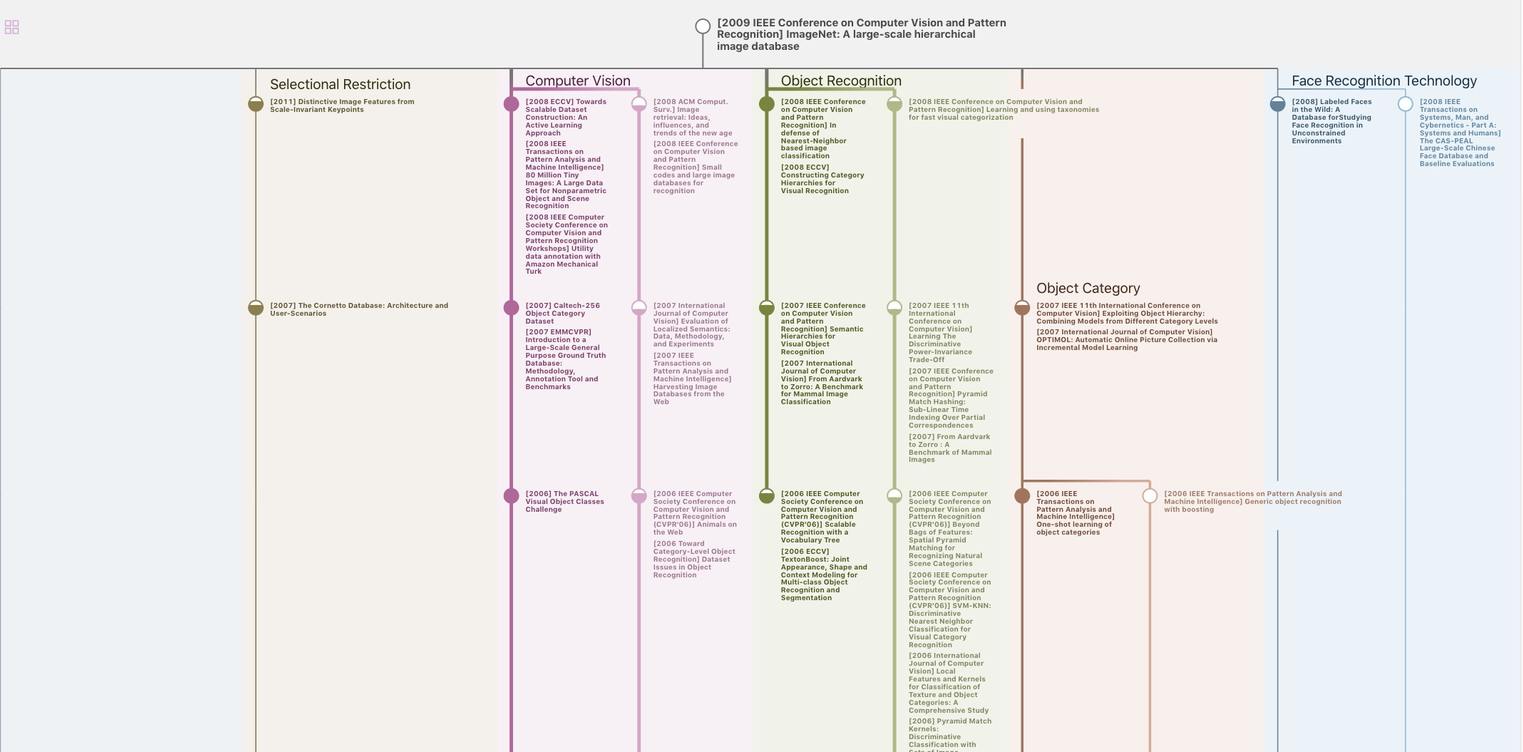
生成溯源树,研究论文发展脉络
Chat Paper
正在生成论文摘要