Cautious Bayesian MPC: Regret Analysis and Bounds on the Number of Unsafe Learning Episodes.
arxiv(2023)
摘要
This article investigates the combination of model predictive control (MPC) concepts and posterior sampling techniques and proposes a simple constraint tightening technique to introduce cautiousness during explorative learning episodes. The provided theoretical analysis in terms of cumulative regret focuses on previously stated sufficient conditions of the resulting "Cautious Bayesian MPC" algorithm and shows Lipschitz continuity of the future reward function in the case of linear MPC problems. In the case of nonlinear MPC problems, it is shown that commonly required assumptions for nonlinear MPC optimization techniques provide sufficient criteria for model-based reinforcement learning using posterior sampling. Furthermore, it is shown that the proposed constraint tightening implies a bound on the expected number of unsafe learning episodes in the linear and nonlinear case using a soft-constrained MPC formulation. The efficiency of the method is illustrated using numerical examples.
更多查看译文
关键词
Constrained control, nonlinear predictive control, predictive control for linear systems, reinforcement learning (RL)
AI 理解论文
溯源树
样例
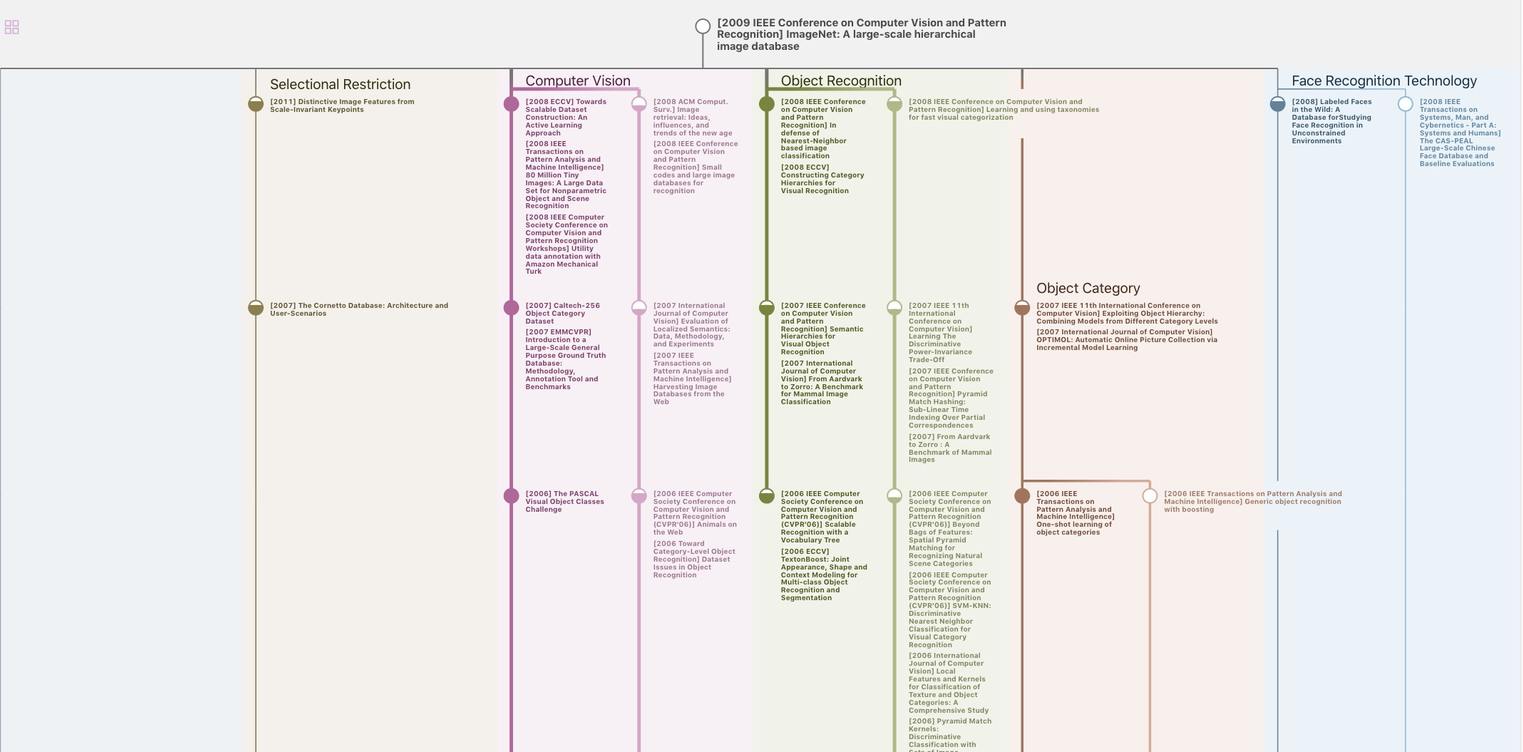
生成溯源树,研究论文发展脉络
Chat Paper
正在生成论文摘要