Single Image Deraining with Continuous Rain Density Estimation
arxiv(2020)
摘要
Single image deraining (SIDR) often suffers from over/under deraining due to the nonuniformity of rain densities and the variety of raindrop scales. In this paper, we propose a \textbf{\it co}ntinuous \textbf{\it de}nsity guided network (CODE-Net) for SIDR. Particularly, it is composed of { a rain {\color{black}streak} extractor and a denoiser}, where the convolutional sparse coding (CSC) is exploited to filter out noises from the extracted rain streaks. Inspired by the reweighted iterative soft-threshold for CSC, we address the problem of continuous rain density estimation by learning the weights with channel attention blocks from sparse codes. We further {\color{black}develop} a multiscale strategy to depict rain streaks appearing at different scales. Experiments on synthetic and real-world data demonstrate the superiority of our methods over recent {\color{black}state of the arts}, in terms of both quantitative and qualitative results. Additionally, instead of quantizing rain density with several levels, our CODE-Net can provide continuous-valued estimations of rain densities, which is more desirable in real applications.
更多查看译文
关键词
Convolutional sparse coding,image deraining,rain density estimation
AI 理解论文
溯源树
样例
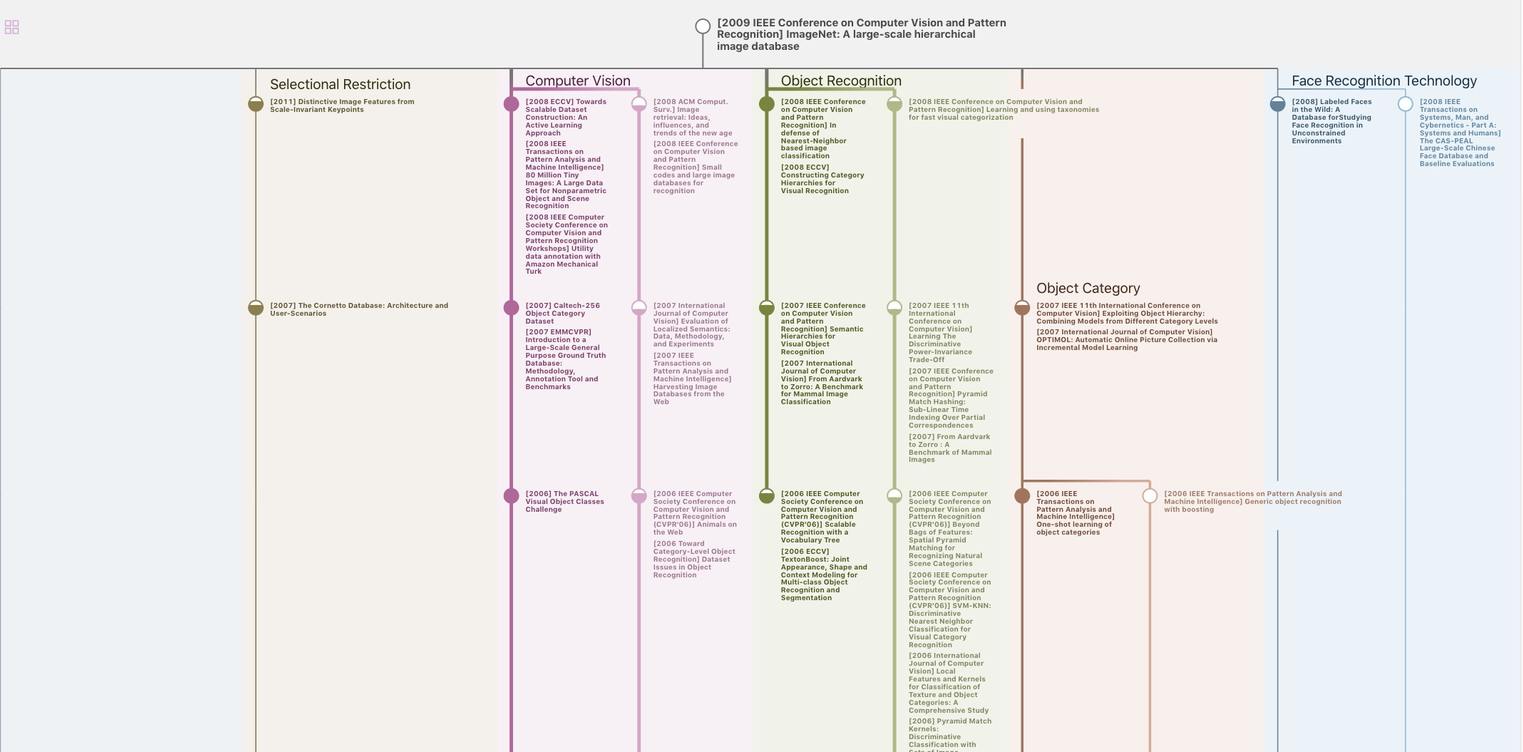
生成溯源树,研究论文发展脉络
Chat Paper
正在生成论文摘要