Understanding the Error Structure as a Key to Regularize Convolutional Neural Networks
user-5ebe28934c775eda72abcddd(2018)
摘要
In large-scale classification, classes that are frequently confused for each other usually exhibit high similarity on the sample level. The similarities define coarse-to-fine hierarchical structure over the classes. We developed a visual analytics system to reveal this structure and analyze how it emerges during the training of deep networks. We found that the network can perform coarse classification into few wide groups of classes early during the training, with subsequent epochs improving the separability between finer groups. Accordingly, we found that the features developed at early layers are capable of performing coarse classification, while the features developed at deeper layers specializing at separating finer groups. We extend the AlexNet network to enforce this behavior on the ImageNet ILSVRC dataset. In particular, we introduce an additional loss function at selected layers that explicitly requires its features to classify the input into class groups that we identified as separable at this level. This enables faster convergence and a reduction of the Top-1 error on ImageNet by more than 20% and of the Top-5 error by more than 30%.
更多查看译文
AI 理解论文
溯源树
样例
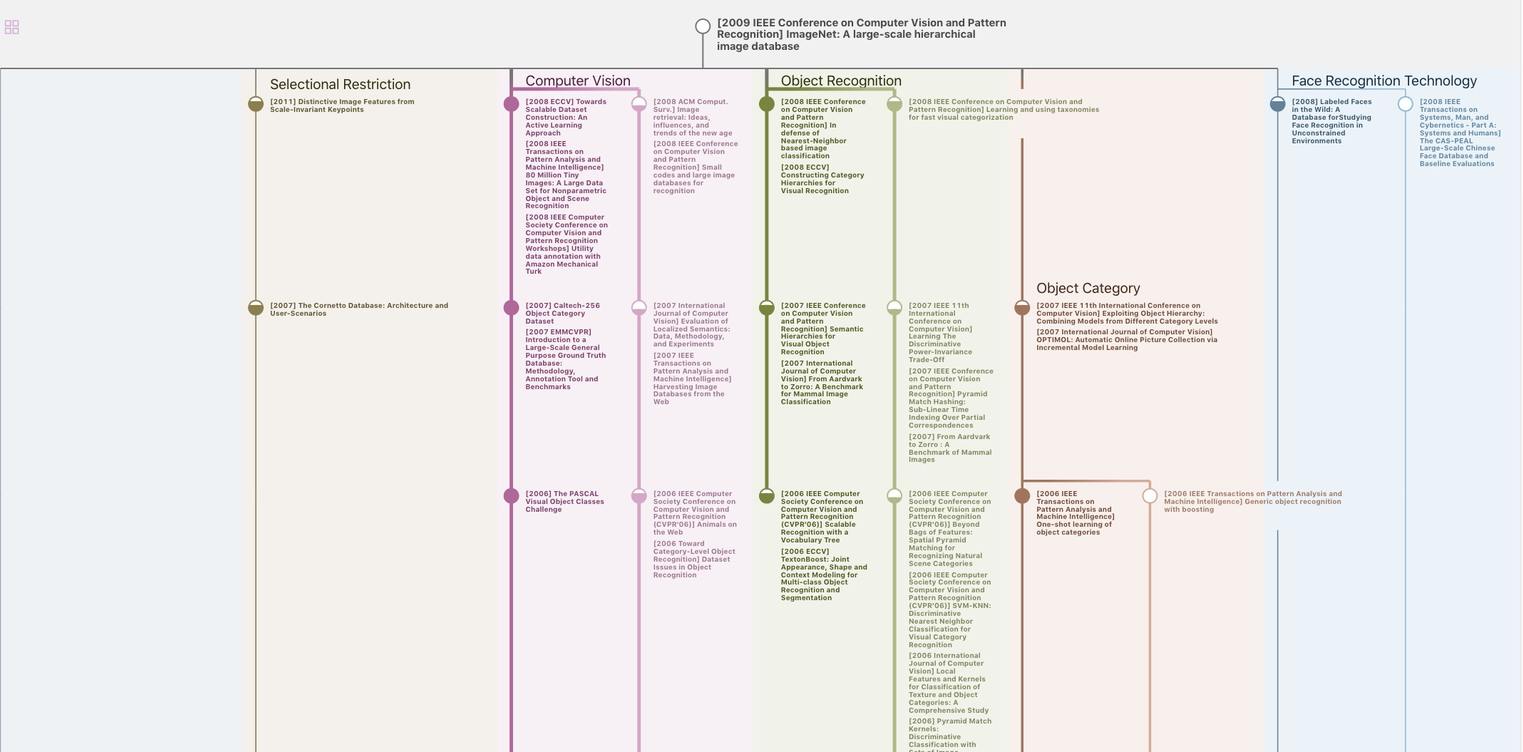
生成溯源树,研究论文发展脉络
Chat Paper
正在生成论文摘要