PLG-IN: Pluggable Geometric Consistency Loss with Wasserstein Distance in Monocular Depth Estimation
2021 IEEE INTERNATIONAL CONFERENCE ON ROBOTICS AND AUTOMATION (ICRA 2021)(2021)
摘要
We propose a novel objective for penalizing geometric inconsistencies and improving the depth and pose estimation performance of monocular camera images. Our objective is designed using the Wasserstein distance between two point clouds, estimated from images with different camera poses. The Wasserstein distance can impose a soft and symmetric coupling between two point clouds, which suitably maintains geometric constraints and results in a differentiable objective. By adding our objective to those of other state-of-the-art methods, we can effectively penalize geometric inconsistencies and obtain highly accurate depth and pose estimations. Our proposed method was evaluated using the KITTI dataset.
更多查看译文
关键词
pluggable geometric consistency loss,Wasserstein distance,monocular depth estimation,monocular camera images,point clouds,symmetric coupling,highly accurate depth
AI 理解论文
溯源树
样例
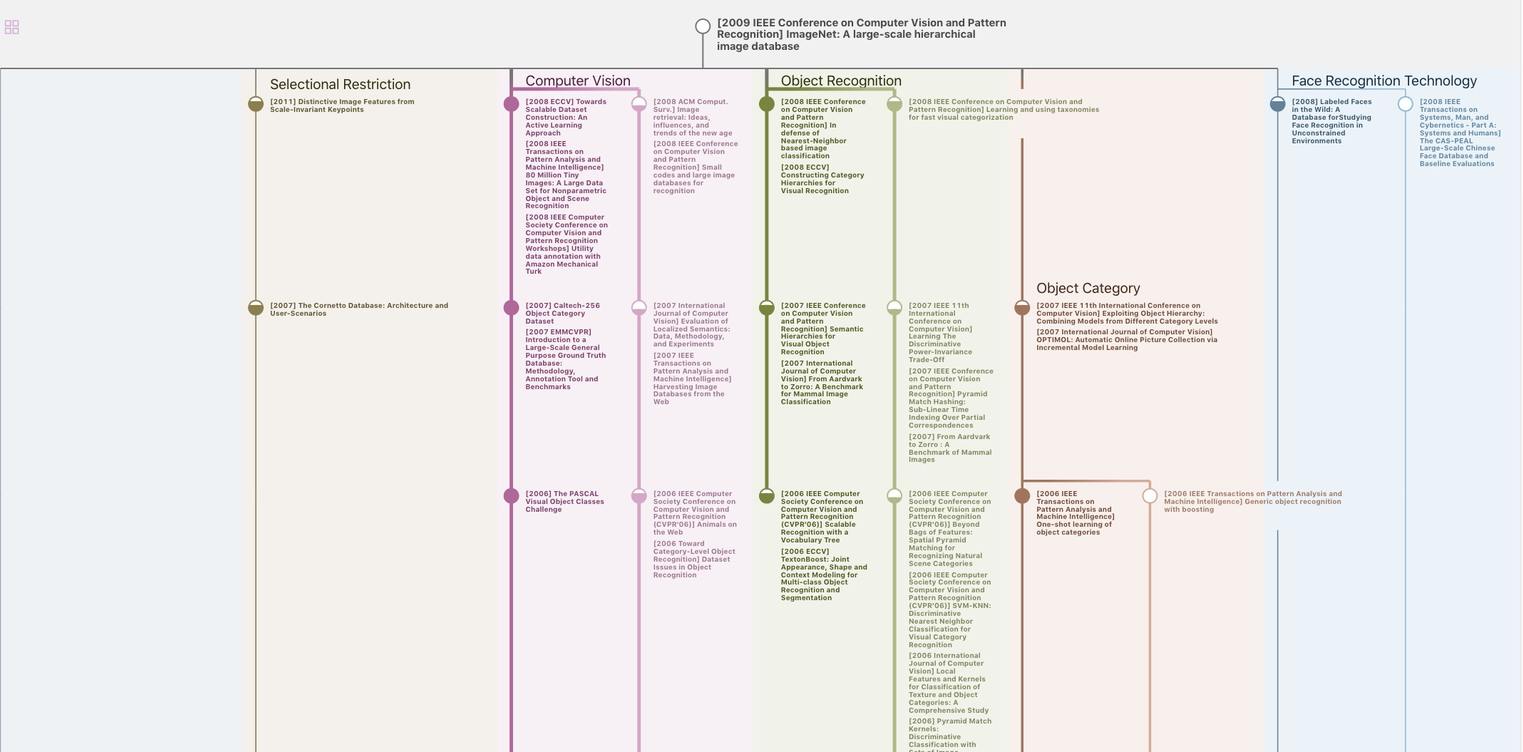
生成溯源树,研究论文发展脉络
Chat Paper
正在生成论文摘要