Ranking via partial ordering for answer selection
Information Sciences(2020)
摘要
Answer selection with deep neural networks has been studied extensively owing to its strong capability of encoding semantic features. Most previous work has treated the candidates as independent individuals for ranking but ignored the relations between these. In this study, we propose a ranking method via partial ordering for answer selection, which is applicable to various tasks, such as question answering and reading comprehension. First, we propose a comparative network, namely Candidate vs. Candidate, which aims to discover the possible partial order relations between (candidate, candidate) pairs. Thereafter, a multi-task learning framework is constructed for the answer selection problem, in which the main task is representing the relevance between (question, answer) pairs, while the auxiliary sub-task is learning the partial order relations between (answer, answer) pairs. By jointly training the networks with abundant supervision information, a reasonable relevance function and a comparison function can be approximated for these tasks. The experimental results on four benchmarks indicate that ranking candidate answers via partial ordering can significantly improve the answer selection performance.
更多查看译文
关键词
Answer selection,Ranking,Partial order relation,Multi-task learning,Question answering
AI 理解论文
溯源树
样例
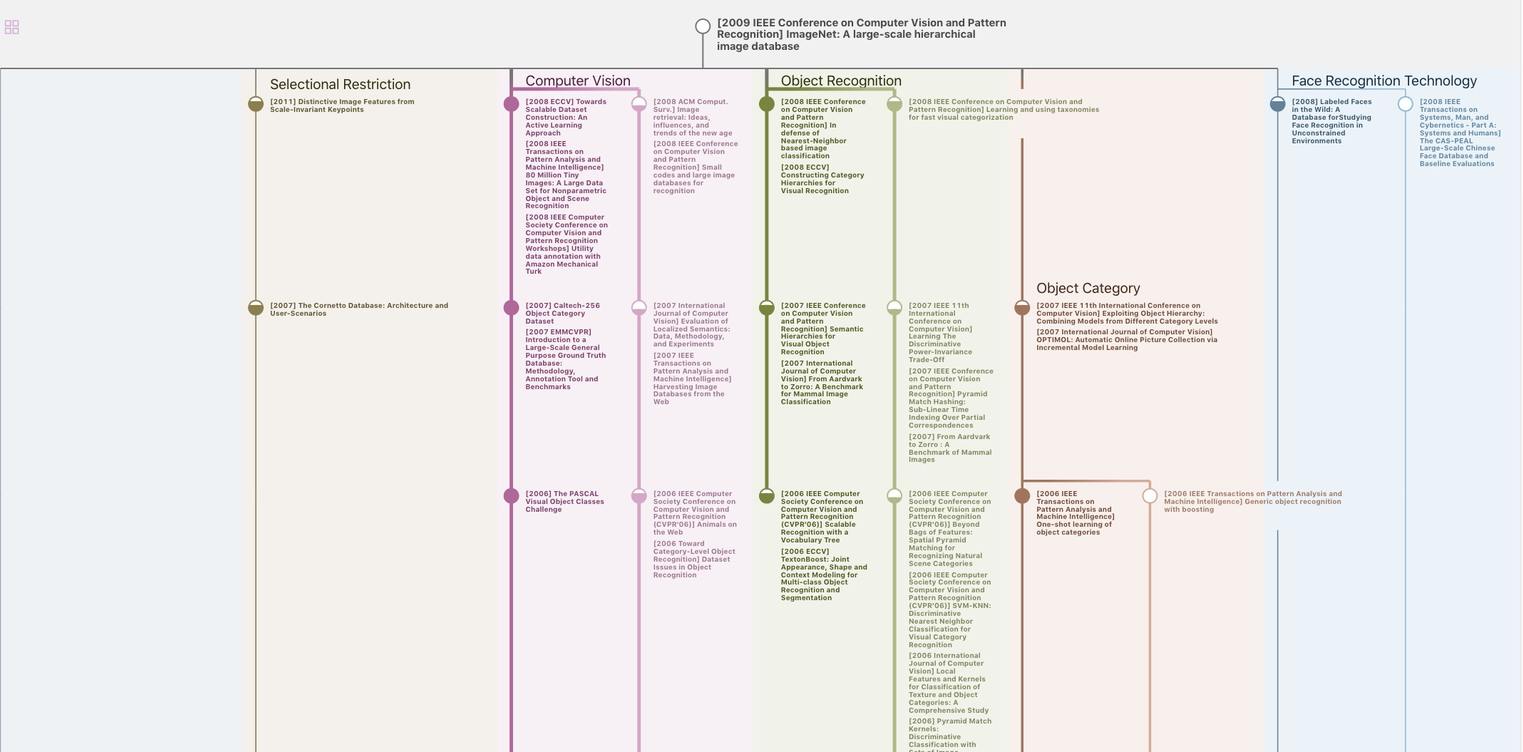
生成溯源树,研究论文发展脉络
Chat Paper
正在生成论文摘要