Object Cosegmentation In Noisy Videos With Multilevel Hypergraph
IEEE TRANSACTIONS ON MULTIMEDIA(2021)
摘要
With the target of simultaneously segmenting semantically related videos to identify the common objects, video object cosegmentation has attracted the attention of researchers in recent years. Existing methods are primarily based on pair-wise relations between adjacent pixels and regions, which are susceptible to performance degradation from object entries/exists or occlusions. Specifically, we refer these video frames without the common objects present as the "empty" frames. In this paper, we propose a multilevel hypergraph-based full Video object CoSegmentation (VCS) method, which incorporates high-level semantics and low-level appearance/motion/saliency to construct the hyperedge among multiple spatially and temporally adjacent regions. Specifically, the high-level semantic model fuses multiple object proposals from each frame instead of relying on a single object proposal per frame. A hypergraph cut is subsequently utilized to calculate the object cosegmentation. Experiments on four video object segmentation/cosegmentation datasets against state-of-the-art methods with both objective and subjective results manifest the effectiveness of the proposed VCS method, including the SegTrack and VCoSeg datasets without "empty" frames, the XJTU-Stevens dataset with 3.7% "empty" frames, and the Noisy-ViCoSeg dataset proposed together with our method with 30.3% "empty" frames.
更多查看译文
关键词
Videos, Proposals, Computational modeling, Object segmentation, Noise measurement, Semantics, Task analysis, Object cosegmentation, hypergraph cut, object model, fully convolutional network
AI 理解论文
溯源树
样例
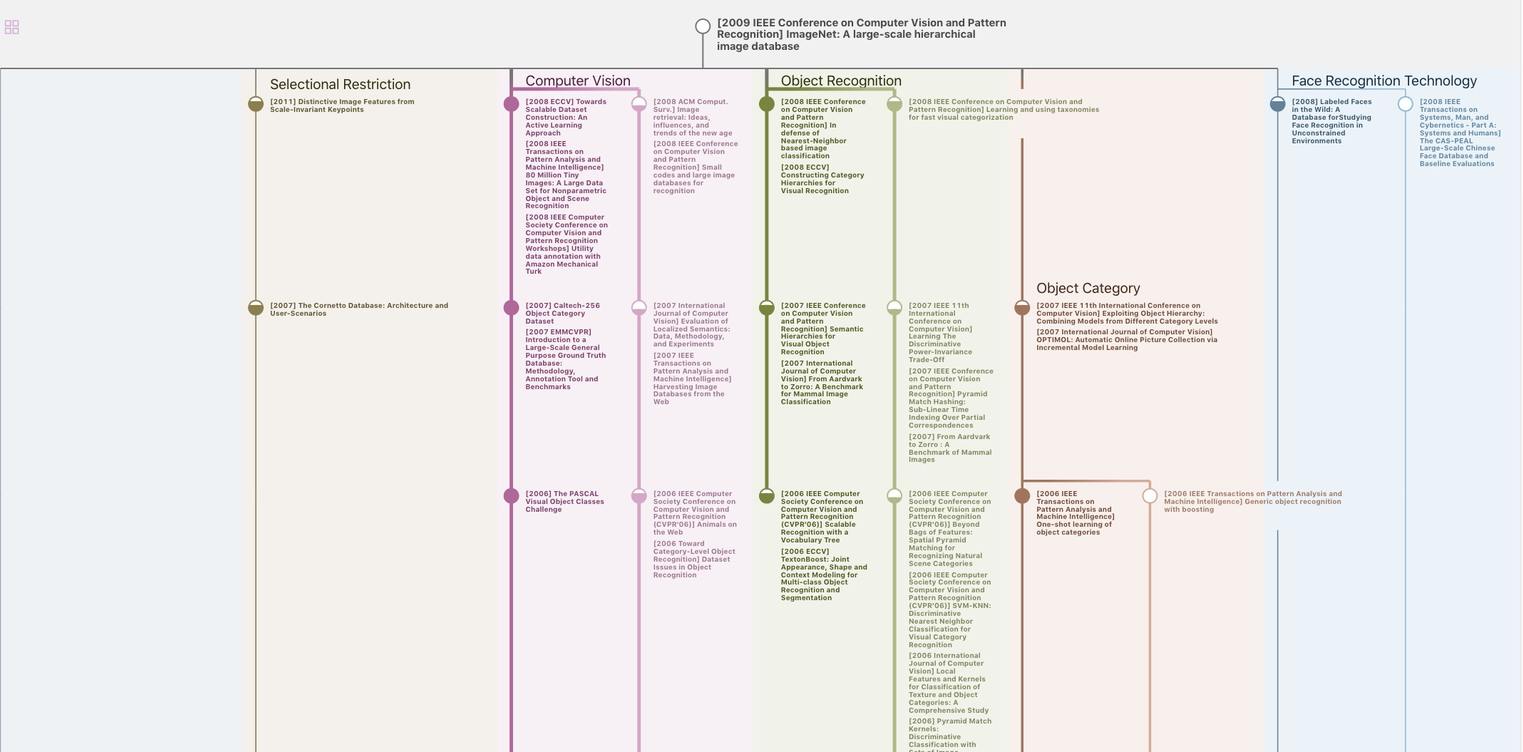
生成溯源树,研究论文发展脉络
Chat Paper
正在生成论文摘要