Hybrid Safe-Strong Rules For Efficient Optimization In Lasso-Type Problems
COMPUTATIONAL STATISTICS & DATA ANALYSIS(2021)
摘要
The lasso model has been widely used for model selection in data mining, machine learning, and high-dimensional statistical analysis. However, with the ultrahigh-dimensional, large-scale data sets now collected in many real-world applications, it is important to develop algorithms to solve the lasso that efficiently scale up to problems of this size. Discarding features from certain steps of the algorithm is a powerful technique for increasing efficiency and addressing the Big Data challenge. This paper proposes a family of hybrid safe-strong rules (HSSR) which incorporate safe screening rules into the sequential strong rule (SSR) to remove unnecessary computational burden. Two instances of HSSR are presented, SSR-Dome and SSR-BEDPP, for the standard lasso problem. SSR-BEDPP is further extended to the elastic net and group lasso problems to demonstrate the generalizability of the hybrid screening idea. Extensive numerical experiments with synthetic and real data sets are conducted for both the standard lasso and the group lasso problems. Results show that the proposed hybrid rules can substantially outperform existing state-of-the-art rules. (C) 2020 Elsevier B.V. All rights reserved.
更多查看译文
关键词
Lasso screening, strong rules, pathwise coordinate descent, large-scale sparse learning
AI 理解论文
溯源树
样例
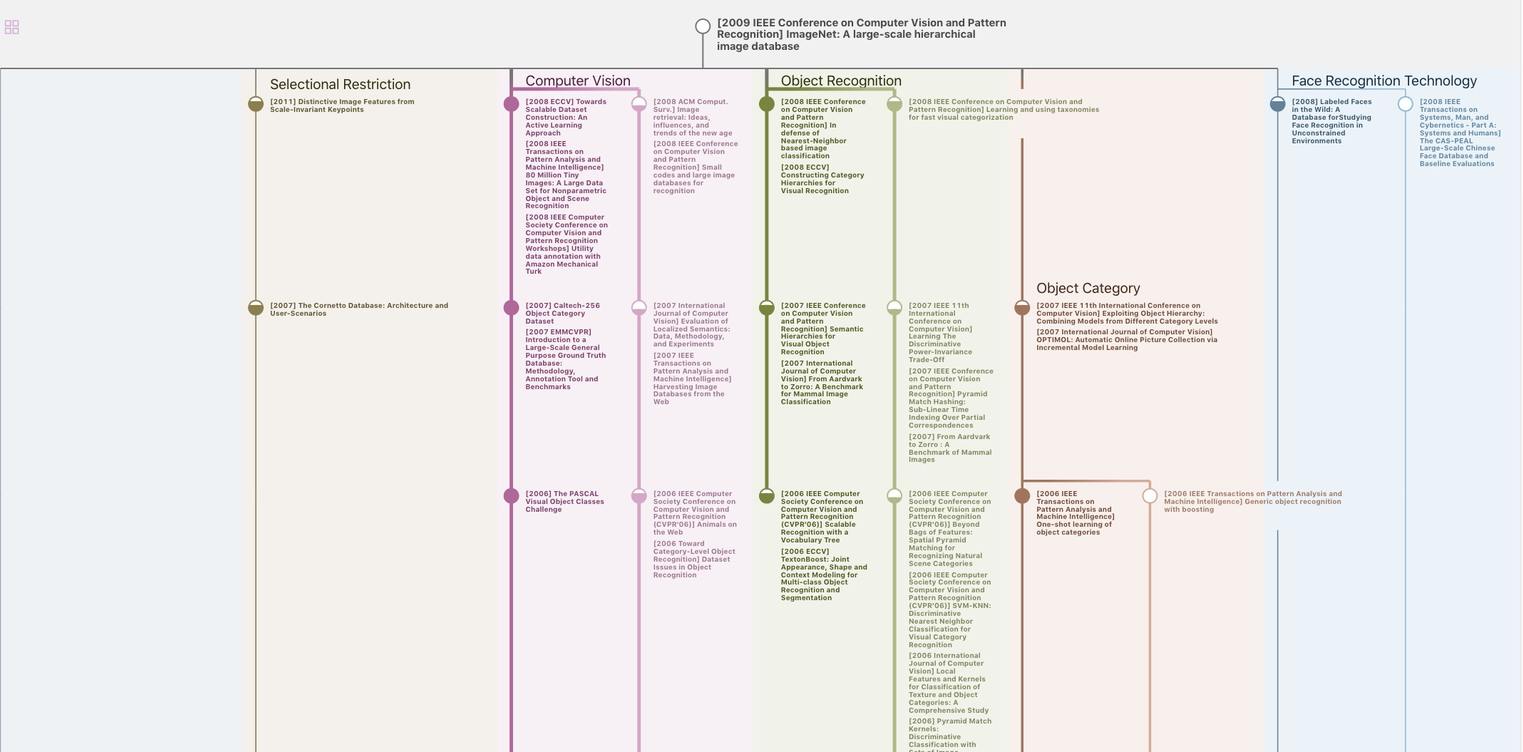
生成溯源树,研究论文发展脉络
Chat Paper
正在生成论文摘要