Anomaly Detection in Traffic Surveillance Videos with GAN-based Future Frame Prediction
ICMR '20: International Conference on Multimedia Retrieval Dublin Ireland June, 2020(2020)
摘要
It is essential to develop efficient methods to detect abnormal events, such as car-crashes or stalled vehicles, from surveillance cameras to provide in-time help. This motivates us to propose a novel method to detect traffic accidents in traffic videos. To tackle the problem where anomalies only occupy a small amount of data, we propose a semi-supervised method using Generative Adversarial Network trained on regular sequences to predict future frames. Our key idea is to model the ordinary world with a generative model, then compare a predicted frame with the real next frame to determine if an abnormal event occurs. We also propose a new idea of encoding motion descriptors and scaled intensity loss function to optimize GAN for fast-moving objects. Experiments on the Traffic Anomaly Detection dataset of AI City Challenge 2019 show that our method achieves the top 3 results with F1 score 0.9412 and RMSE 4.8088, and S3 score 0.9261. Our method can be applied to different related applications of anomaly and outlier detection in videos.
更多查看译文
AI 理解论文
溯源树
样例
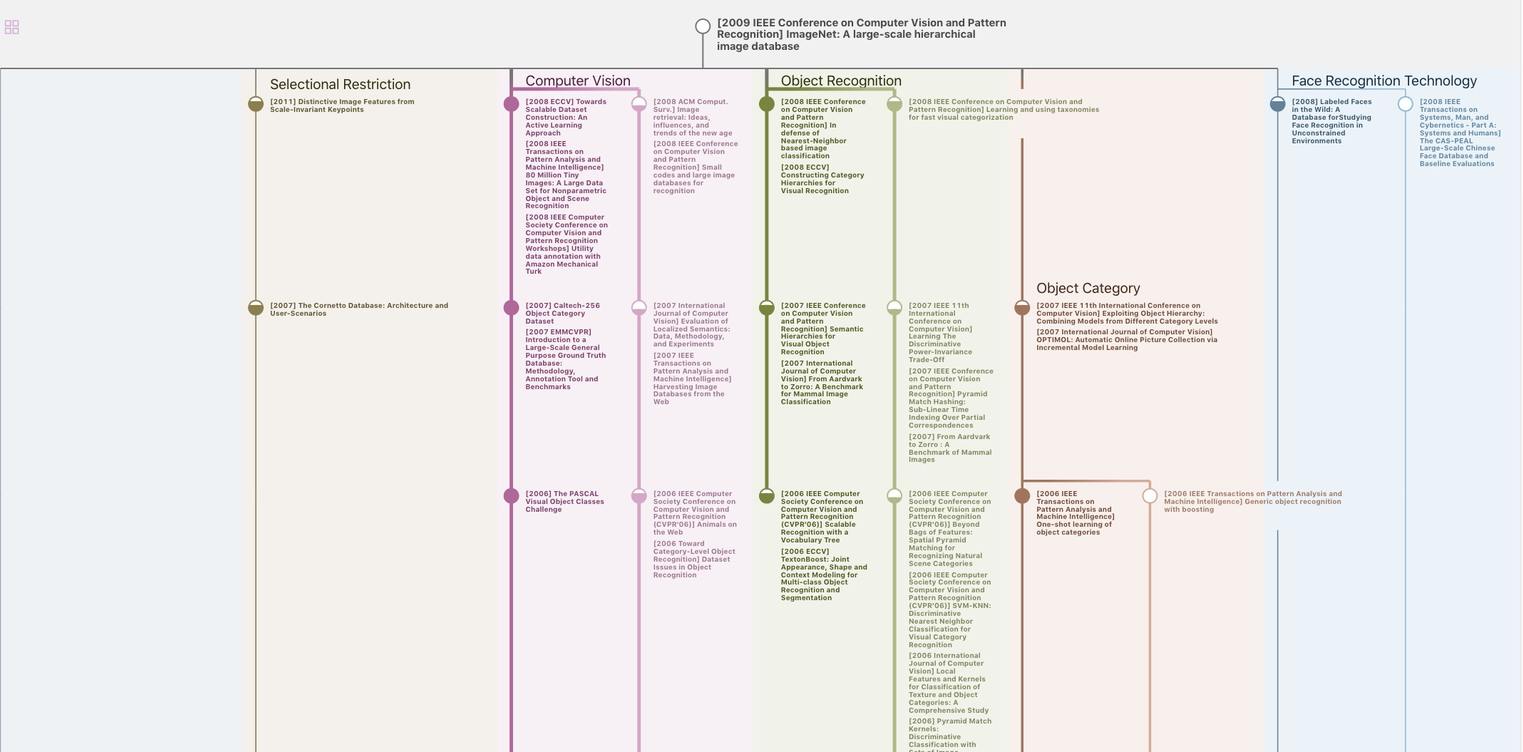
生成溯源树,研究论文发展脉络
Chat Paper
正在生成论文摘要