Flexible Text Modeling with Semi-Implicit Latent Representations
NeurIPS workshop, Bayesian Deep Learning(2019)
摘要
Variational autoencoders (VAEs) have been used recently to learn probabilistic representations in many natural language processing (NLP) applications. Training deficiency has been witnessed when an auto-regressive decoder is used: the learned latent codes becomes almost identical to the prior distribution (termed “posterior collapse,” resulting in a vanishing of the Kullback-Leibler term in the variational expression). We hypothesize that the source of deficiency is due partially to the approximate Gaussian posterior often used in variational inference. We use semiimplicit (SI) representations for the latent distributions of the natural languages. It extends the commonly used Gaussian distribution family, by mixing the variational parameter with a flexible implicit distribution. The increasing representational power of SI is demonstrated on two NLP tasks, showing that it provides more informative latent codes in language modeling, and generates more diverse dialog responses.
更多查看译文
AI 理解论文
溯源树
样例
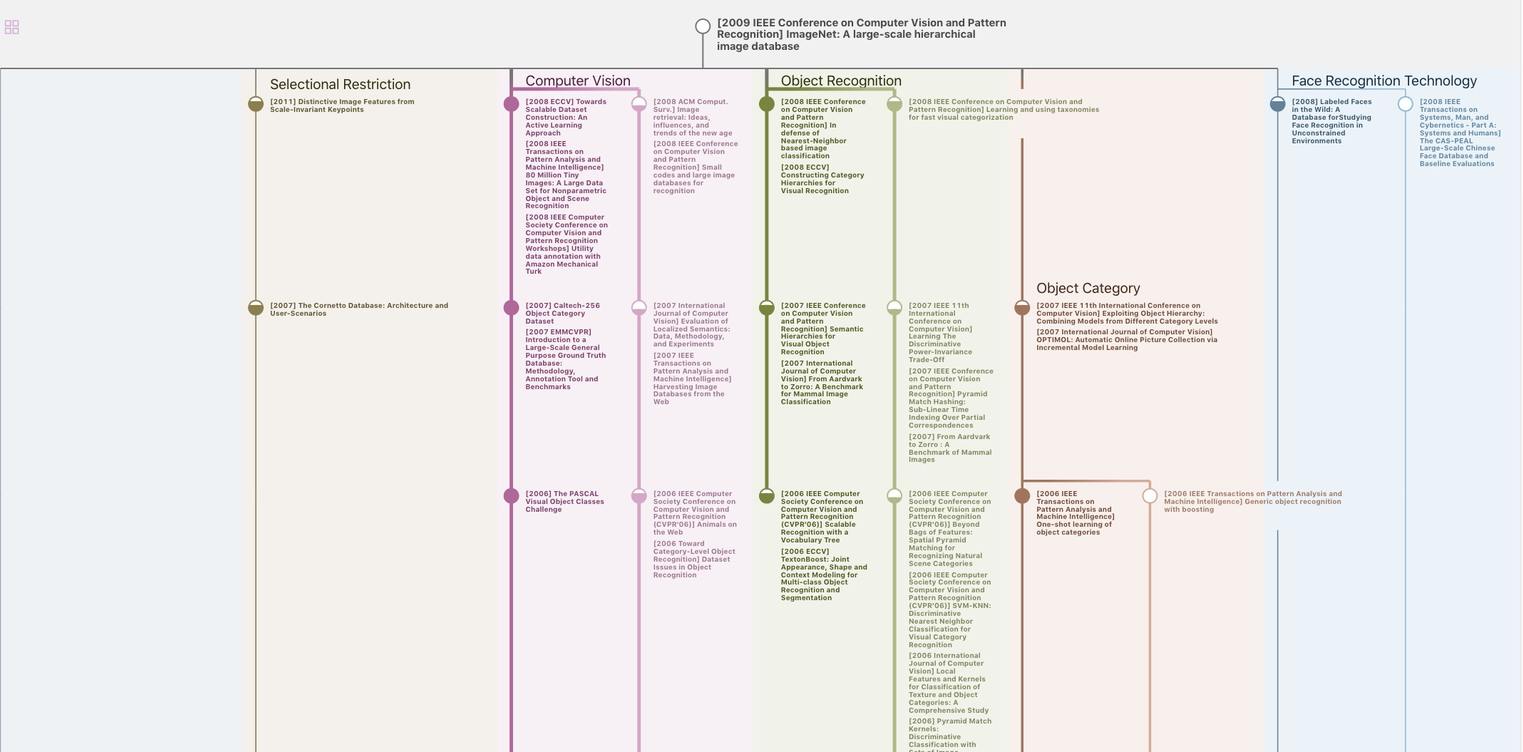
生成溯源树,研究论文发展脉络
Chat Paper
正在生成论文摘要