Error Metrics and Performance Fitness Indicators for Artificial Intelligence and Machine Learning in Engineering and Sciences
Architecture, Structures and Construction(2023)
摘要
Artificial intelligence (AI) and Machine learning (ML) train machines to achieve a high level of cognition and perform human-like analysis. Both AI and ML seemingly fit into our daily lives as well as complex and interdisciplinary fields. With the rise of commercial, open-source, and user-catered AI/ML tools, a key question often arises whenever AI/ML is applied to explore a phenomenon or a scenario: what constitutes a good AI/ML model? Keeping in mind that a proper answer to this question depends on various factors, this work presumes that a goodmodel optimally performs and best describes the phenomenon on hand . From this perspective, identifying proper assessment metrics to evaluate the performance of AI/ML models is not only necessary but is also warranted. As such, this paper examines 78 of the most commonly-used performance fitness and error metrics for regression and classification algorithms, with emphasis on engineering and sciences applications.
更多查看译文
关键词
Error metrics, Machine learning, Regression, Classification
AI 理解论文
溯源树
样例
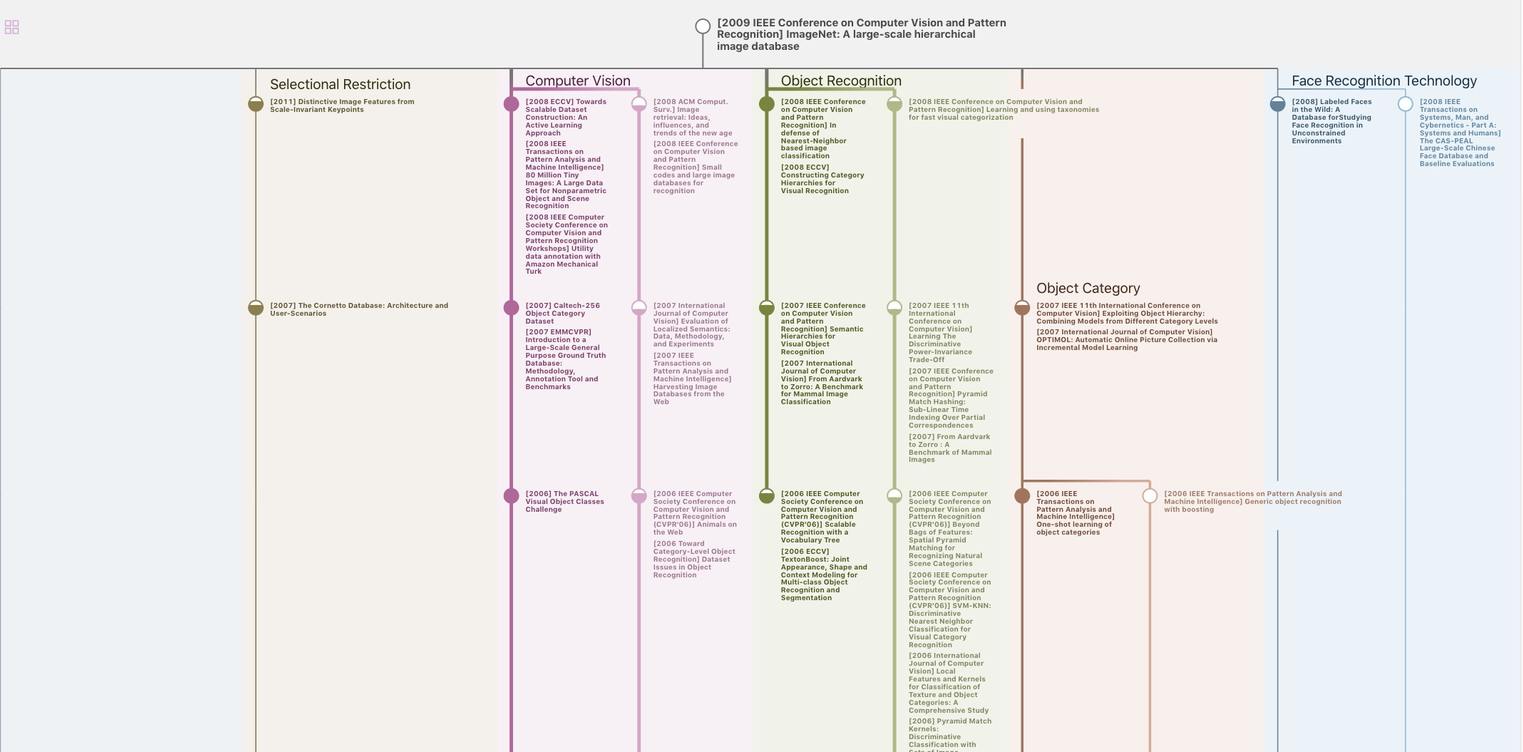
生成溯源树,研究论文发展脉络
Chat Paper
正在生成论文摘要