Hybrid deep neural network based prediction method for unsteady flows with moving boundary
Acta Mechanica Sinica(2021)
Abstract
Any motion, forced or free, of boundary affects the flow field around this boundary. A new kind of reduced order model (ROM) based on hybrid deep neural network is proposed to model flow field evolution process of unsteady flow around moving boundary. This hybrid deep neural network can map the relationship between the flow field at the next time step and the flow field and boundary positions at the previous time steps. Based on the learned information, the hybrid deep neural network can quickly and accurately predict the flow field. Unsteady flows around forced oscillation cylinder with various amplitudes, frequencies, and Reynolds numbers are simulated to establish the training and testing datasets. The prediction results of the hybrid deep neural network and the computational fluid dynamics (CFD) simulation results are consistent with high accuracy. The forces on the moving boundary can be integrated through the predicted flow field data. Good performance makes this new ROM method can be used in many fluid dynamics research fields, which needs fast and accurate simulation.
MoreTranslated text
Key words
Deep neural network,Flow fields prediction,Moving boundary
AI Read Science
Must-Reading Tree
Example
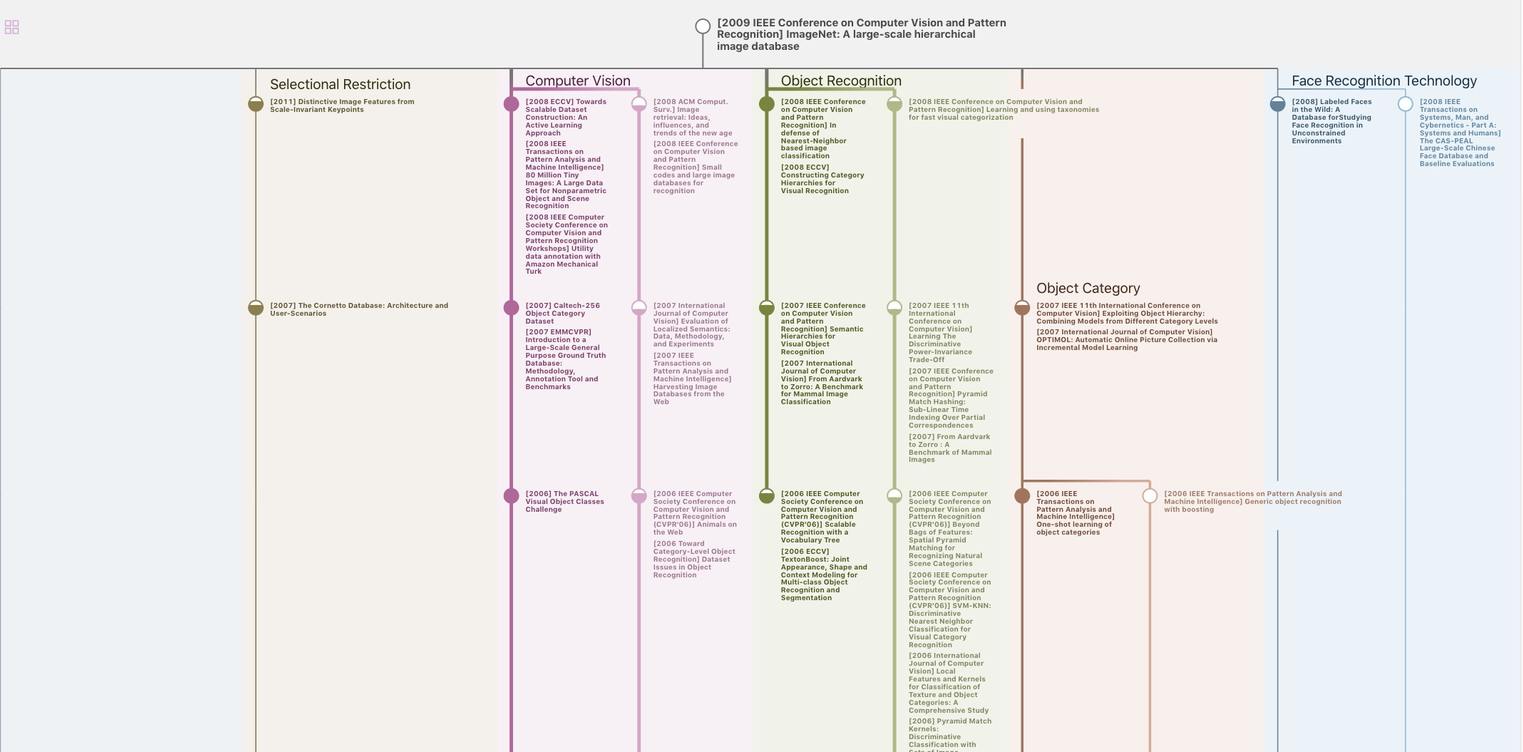
Generate MRT to find the research sequence of this paper
Chat Paper
Summary is being generated by the instructions you defined