Hyperspectral image denoising via global spatial-spectral total variation regularized nonconvex local low-rank tensor approximation
SIGNAL PROCESSING(2021)
摘要
Hyperspectral image (HSI) denoising aims to restore clean HSI from the noise-contaminated one which is usually caused during data acquisition and conversion. In this paper, we propose a novel spatial-spectral total variation (SSTV) regularized nonconvex local low-rank (LR) tensor approximation method to remove mixed noise in HSIs. From one aspect, the clean HSI data have its underlying local LR tensor property, even though the real HSI data is not globally low-rank due to the non-independent and non-identically distributed noise and out-hers. According to this fact, we propose a novel tensor L-gamma-norm to formulate the local LR prior. From another aspect, HSIs are assumed to be piecewisely smooth in the global spatial and spectral domains. Instead of traditional bandwise total variation, we use the SSTV regularization to simultaneously consider global spatial and spectral smoothness. Results on simulated and real HSI datasets indicate that the use of local LR tensor penalty and global SSTV can boost the preserving of local details and overall structural information in HSIs. (C) 2020 Elsevier B.V. All rights reserved.
更多查看译文
关键词
Hyperspectral images,Denoising,Mixed noise,Nonconvex,Local low-rank,Spatial-spectral total variation
AI 理解论文
溯源树
样例
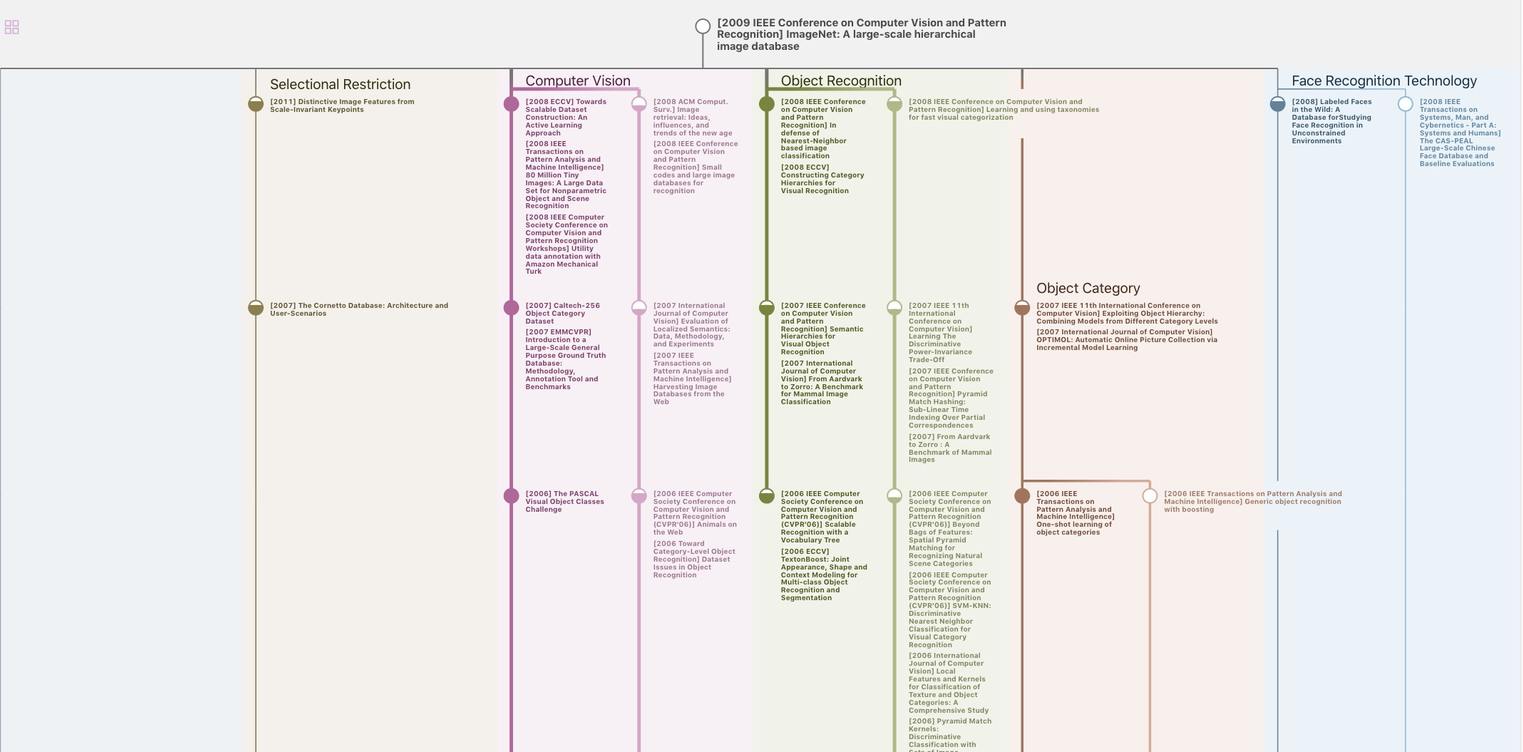
生成溯源树,研究论文发展脉络
Chat Paper
正在生成论文摘要