rknn: an R Package for Parallel Random KNN Classification with Variable Selection
The R User Conference, useR! 2013 July 10-12 2013 University of Castilla-La Mancha, Albacete, Spain(2013)
摘要
Random KNN (RKNN) is a novel generalization of traditional nearest-neighbor modeling. Random KNN consists of an ensemble of base k-nearest neighbor models, each constructed from a random subset of the input variables. A collection of r such base classifiers is combined to build the final Random KNN classifier. Since the base classifiers can be computed independently of one another, the overall computation is embarrassingly parallel.Random KNN can be used to select important features using the RKNN-FS algorithm. RKNN-FS is an innovative feature selection procedure for “small n, large p problems.” Empirical results on microarray data sets with thousands of variables and relatively few samples show that RKNN-FS is an effective feature selection approach for high-dimensional data. RKNN is similar to Random Forests (RF) in terms of classification accuracy without feature selection. However, RKNN provides much better classification accuracy than RF when each method incorporates a feature-selection step. RKNN is significantly more stable and robust than Random Forests for feature selection when the input data are noisy and/or unbalanced. Further, RKNN-FS is much faster than the Random Forests feature selection method (RF-FS), especially for large scale problems involving thousands of variables and/or multiple classes.
更多查看译文
AI 理解论文
溯源树
样例
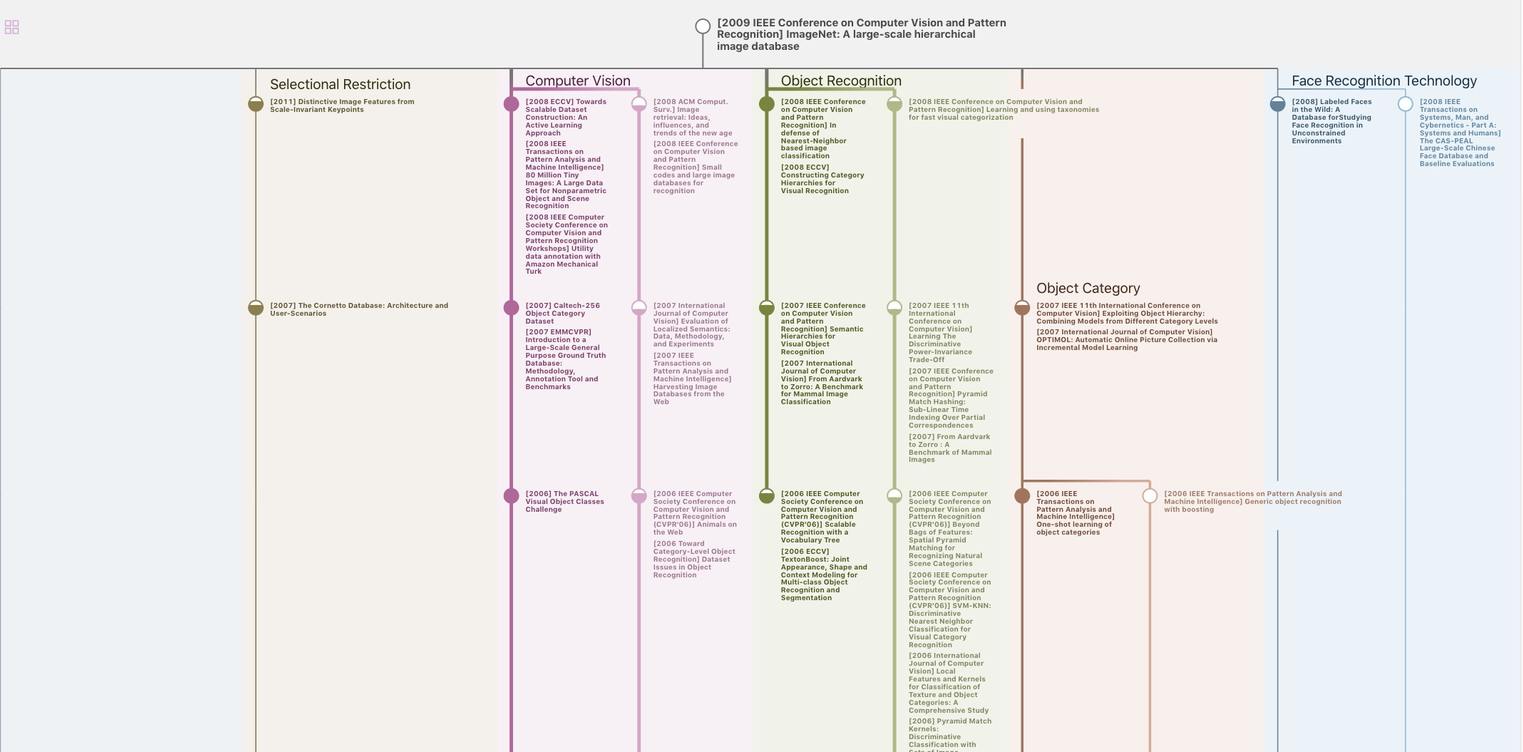
生成溯源树,研究论文发展脉络
Chat Paper
正在生成论文摘要