Content-Equivalent Translated Parallel News Corpus and Extension of Domain Adaptation for Neural Machine Translation
LREC(2020)
摘要
In this paper, we deal with two problems in Japanese-English machine translation of news articles. The first problem is the quality of parallel corpora. Neural machine translation (NMT) systems suffer degraded performance when trained with noisy data. Because there is no clean Japanese-English parallel data for news articles, we build a novel parallel news corpus consisting of Japanese news articles translated into English in a content-equivalent manner. This is the first content-equivalent Japanese-English news corpus translated specifically for training NMT systems. The second problem involves the domain-adaptation technique. NMT systems suffer degraded performance when trained with mixed data having different features, such as noisy data and clean data. Though existing domainadaptation methods try to overcome this problem by using tags to distinguish the differences between corpora, it is not sufficient. We thus extend a domain-adaptation method by using multiple tags to train an NMT model effectively with both the clean corpus and existing parallel news corpora with some types of noise. Experimental results show that our corpus increases the translation quality, and that our domain-adaptation method is more effective for learning with multiple types of corpora than existing domain-adaptation methods are.
更多查看译文
关键词
Parallel News Corpus, Japanese-English, Machine Translation, Domain Adaptation, Back-Translation
AI 理解论文
溯源树
样例
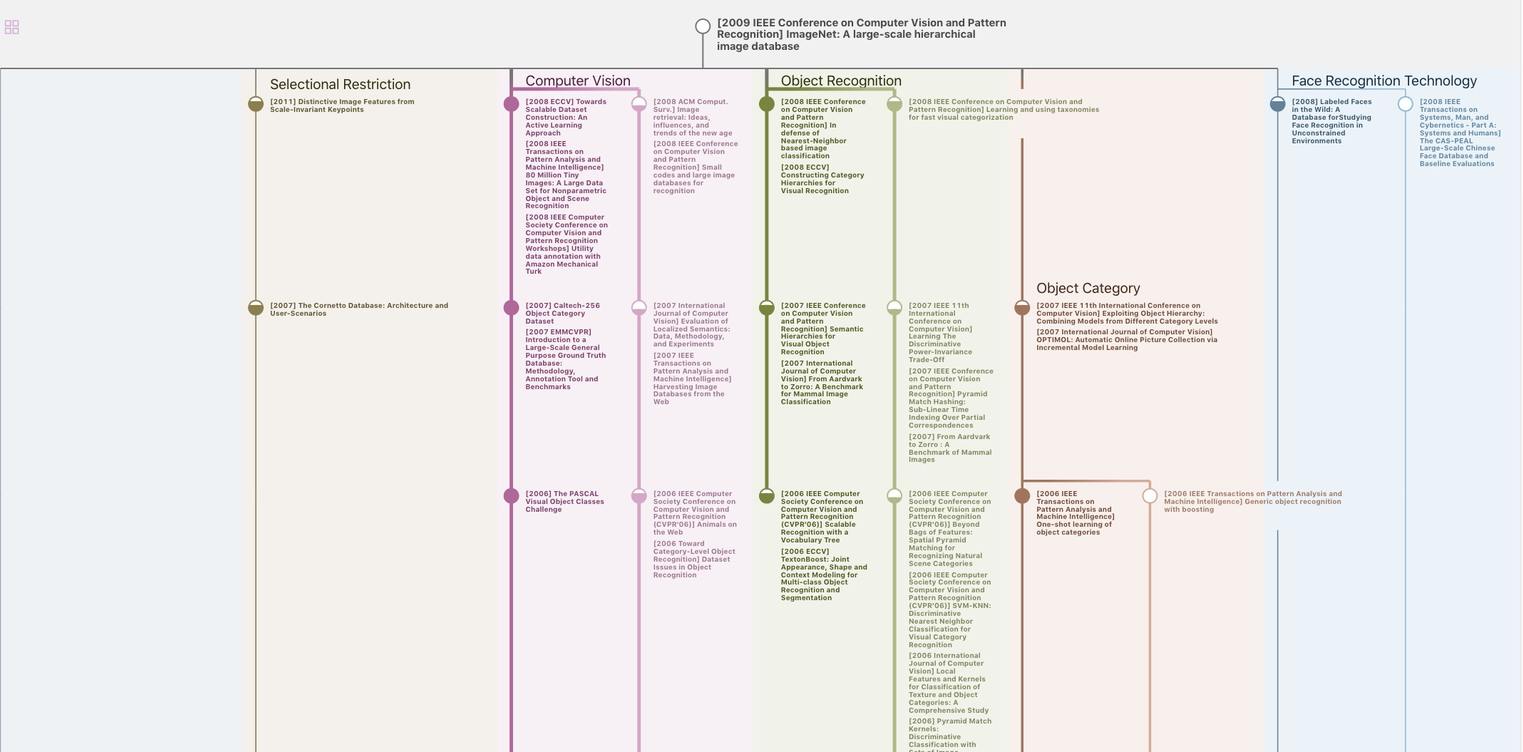
生成溯源树,研究论文发展脉络
Chat Paper
正在生成论文摘要