Bigan: Collaborative Filtering With Bidirectional Generative Adversarial Networks
PROCEEDINGS OF THE 2020 SIAM INTERNATIONAL CONFERENCE ON DATA MINING (SDM)(2020)
摘要
Recently, GAN-based collaborative filtering methods have gained increasing attention in recommendation tasks which can learn remarkable user and item representation. However, these existing GAN-based methods mainly suffer from two limitations: (1) Their trainings are not comprehensive given the fact that the discriminator may be trained misleadingly and over-early converging since the generator may accidentally sample real items as fake ones, resulting in the emergence of contradicting labels for the same items. (2) They fail to consider implicit friends (users with the same interests.), leading to severe limitations of recommendation performance. In this paper, we propose BiGAN, an innovative bidirectional adversarial recommendation model which can alleviate the limitations mentioned above in recommendation tasks. It consists of two GANs, namely ForwardGAN and BackwardGAN. Specifically, ForwardGAN learns to generate a group of possible interacted items given a specific user, it aims to ensure that the discriminator D-f can be trained effectively. Furthermore, BackwardGAN fully exploits implicit friends with similar behaviors, then propagates them back to ForwardGAN, where a similarity exploration strategy is implemented to gain more outstanding user representation. Therefore, two GANs are trained jointly in a circle, where the augment of one GAN will enhance another one, leading to the promising user and item representation. In the experimental part, we demonstrate that our model is superior to other state-of-the-art recommenders.
更多查看译文
AI 理解论文
溯源树
样例
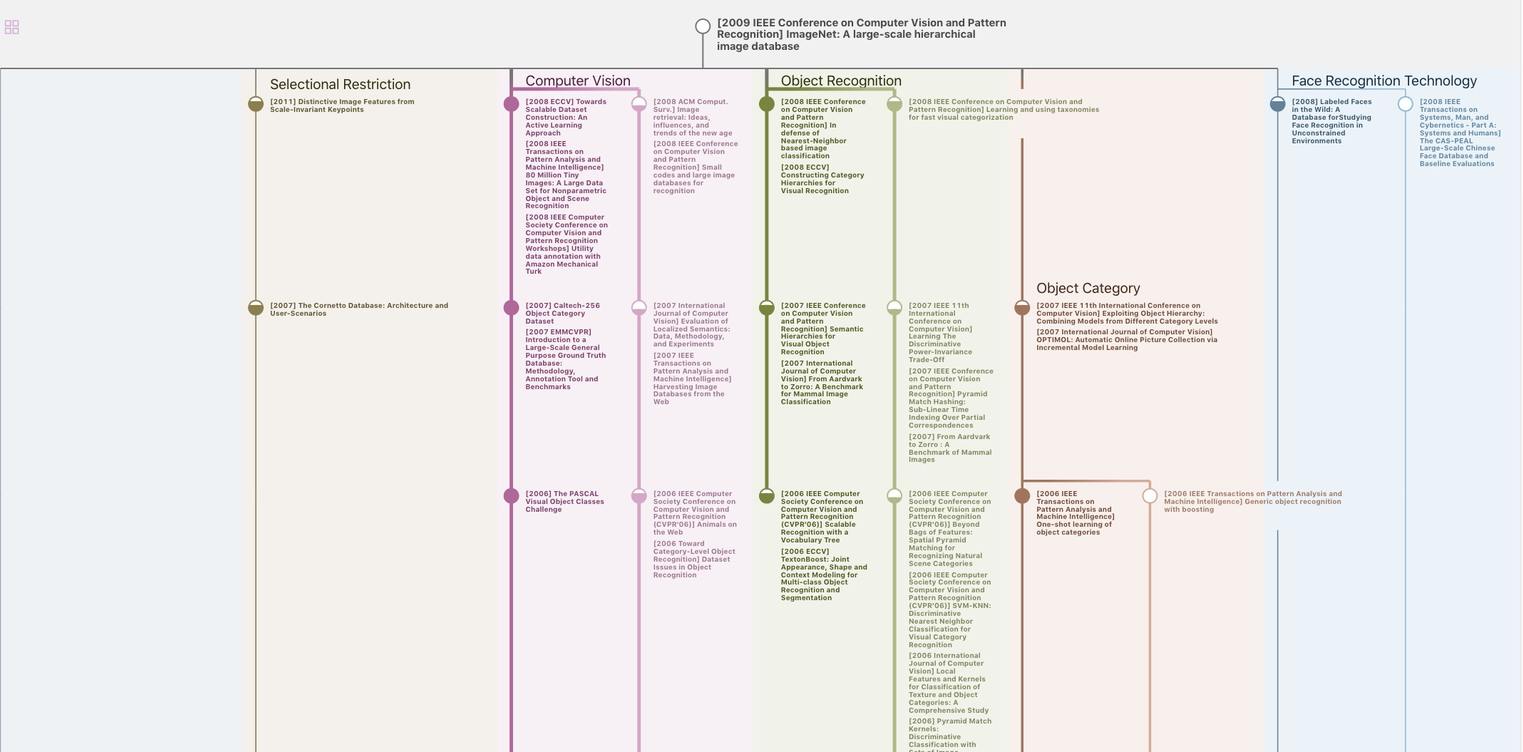
生成溯源树,研究论文发展脉络
Chat Paper
正在生成论文摘要