Dual-Attention Recurrent Networks For Affine Registration Of Neuroimaging Data
PROCEEDINGS OF THE 2020 SIAM INTERNATIONAL CONFERENCE ON DATA MINING (SDM)(2020)
摘要
Neuroimaging data typically undergoes several preprocessing steps before further analysis and mining can be done. Affine image registration is one of the important tasks during preprocessing. Recently, several image registration methods which are based on Convolutional Neural Networks have been proposed. However, due to the high computational and memory requirements of CNNs, these methods cannot be used in real-time for large neuroimaging data like fMRI. In this paper, we propose a Dual-Attention Recurrent Network (DRN) which uses a hard attention mechanism to allow the model to focus on small, but task-relevant, parts of the input image - thus reducing computational and memory costs. Furthermore, DRN naturally supports inhomogeneity between the raw input image (e.g., functional MRI) and the image we want to align it to (e.g., anatomical MRI) so it can be applied to harder registration tasks such as fMRI coregistration and normalization. Extensive experiments on two different datasets demonstrate that DRN significantly reduces the computational and memory costs compared with other neural network-based methods without sacrificing the quality of image registration.
更多查看译文
关键词
Attention Model, Recurrent Neural Network, Deep Learning, fMRI
AI 理解论文
溯源树
样例
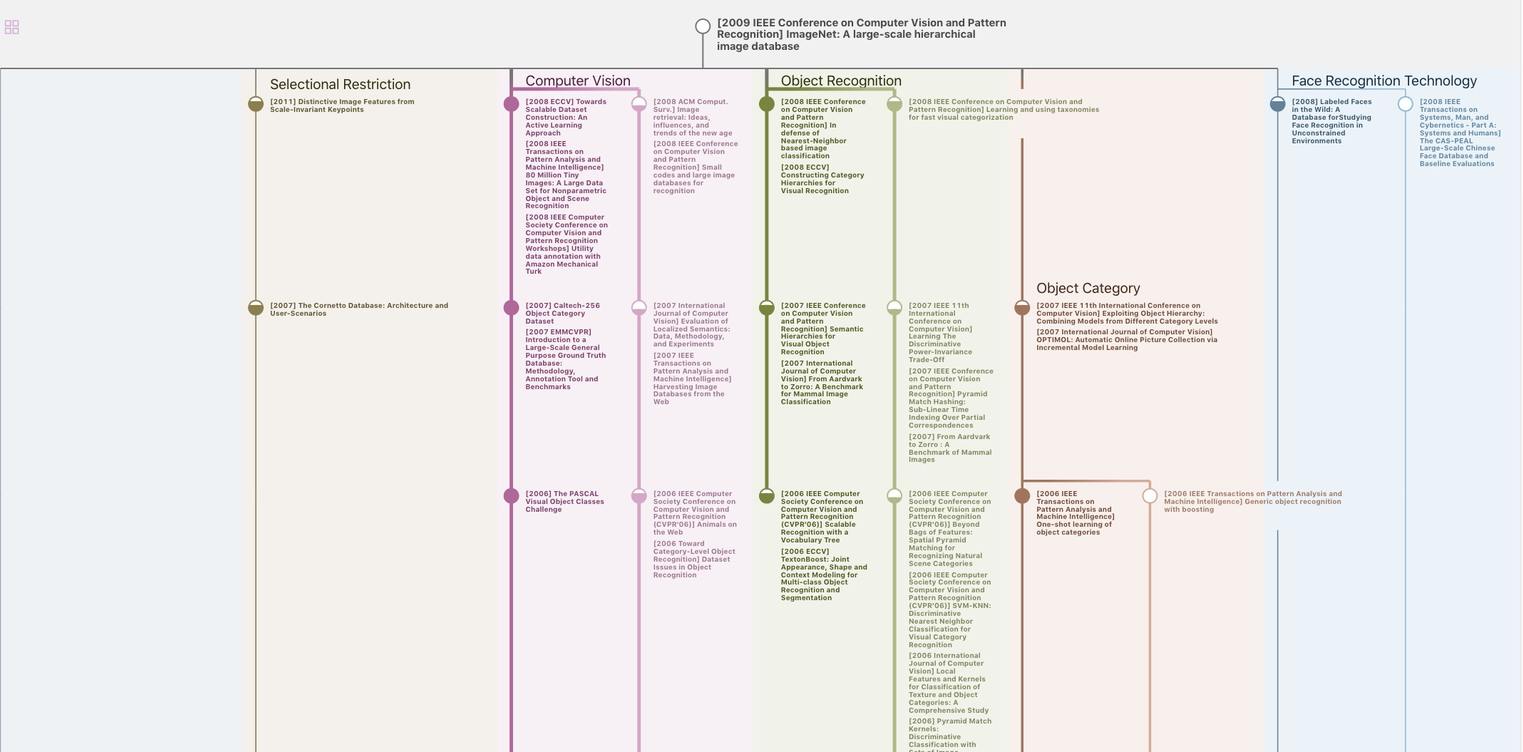
生成溯源树,研究论文发展脉络
Chat Paper
正在生成论文摘要