Supervised convolutional neural networks for classification of flaring and nonflaring active regions using line-of-sight magnetograms
ASTROPHYSICAL JOURNAL(2020)
摘要
Solar flares are explosions in the solar atmosphere that release intense bursts of short-wavelength radiation and are capable of producing severe space-weather. Flares release free energy built up in coronal fields, which are rooted in active regions (ARs) on the photosphere, via magnetic reconnection. The exact processes that lead to reconnection are not fully known and therefore reliable forecasting of flares is challenging. Recently, photospheric magnetic-field data has been extensively analyzed using machine learning (ML) and these studies suggest that flare-forecasting accuracy does not strongly depend on how long in advance flares are predicted. Here, we use ML to understand the evolution of AR magnetic fields before and after flares. We explicitly train convolutional neural networks (CNNs) to classify Solar Dynamics Observatory/Helioseismic and Magnetic Imager line-of-sight magnetograms into ARs producing at least one M- or X-class flare or as nonflaring. We find that flaring ARs remain in flare-productive states-marked by recall > 60% with a peak of similar to 80%-days before and after flares. We use occlusion maps and statistical analysis to show that the CNN pays attention to regions between the opposite polarities from ARs and the CNN output is dominantly decided by the total unsigned line-of-sight flux of ARs. Using synthetic bipole magnetograms, we find spurious dependencies of the CNN output on magnetogram dimensions for a given bipole size. Our results suggest that it is important to use CNN designs that eliminate such artifacts in CNN applications for processing magnetograms and, in general, solar image data.
更多查看译文
关键词
Convolutional neural networks,Solar flares,Astronomy data analysis
AI 理解论文
溯源树
样例
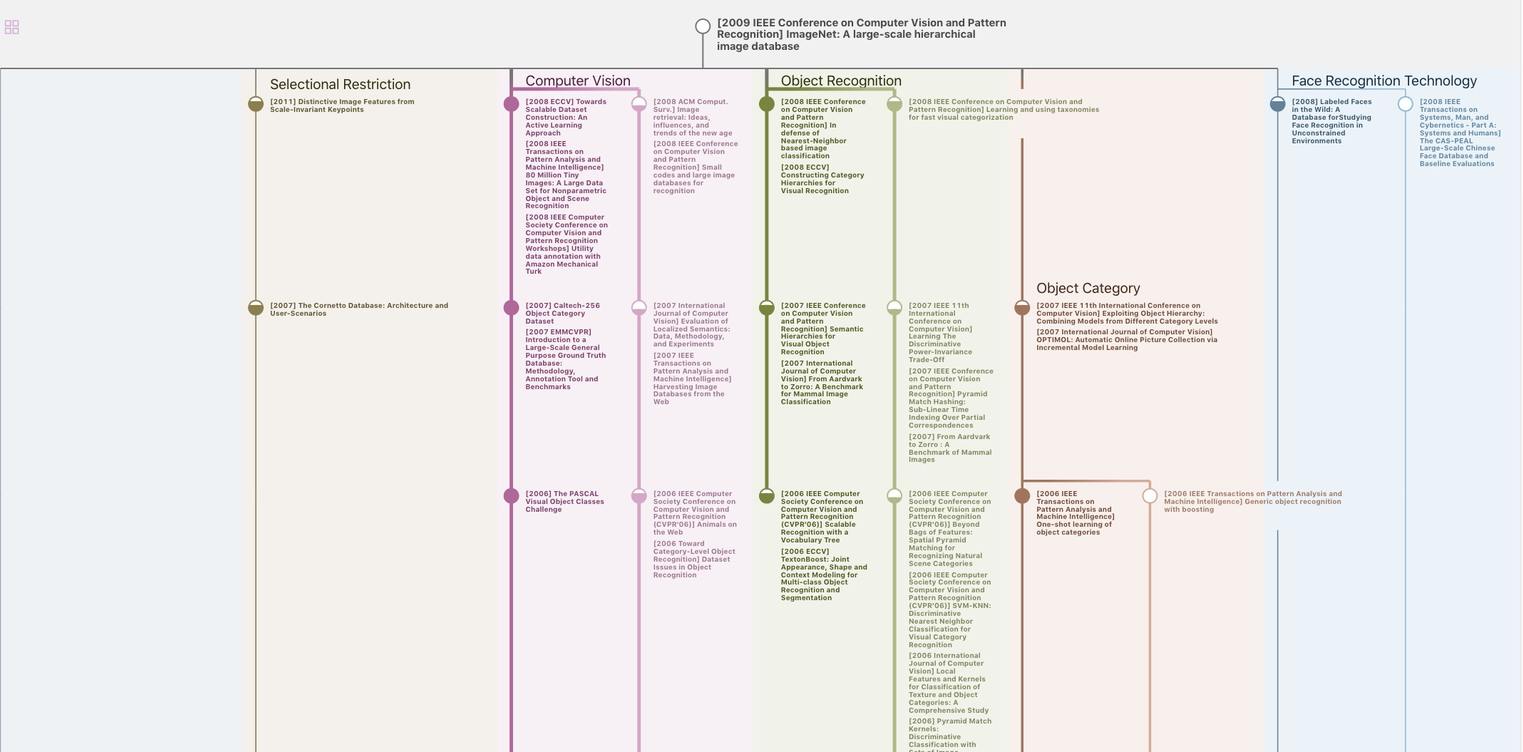
生成溯源树,研究论文发展脉络
Chat Paper
正在生成论文摘要