Self-Supervised, Semi-Supervised, Multi-Context Learning for the Combined Classification and Segmentation of Medical Images
AAAI Conference on Artificial Intelligence(2020)
摘要
To tackle the problem of limited annotated data, semi-supervised learning is attracting attention as an alternative to fully supervised models. Moreover, optimizing a multiple-task model to learn "multiple contexts" can provide better generalizability compared to single-task models. We propose a novel semi-supervised multiple-task model leveraging self-supervision and adversarial training-namely, self-supervised, semi-supervised, multi-context learning ((SMCL)-M-4)-and apply it to two crucial medical imaging tasks, classification and segmentation. Our experiments on spine X-rays reveal that the (SMCL)-M-4 model significantly outperforms semi-supervised single-task, semi-supervised multi-context, and fully-supervised single-task models, even with a 50% reduction of classification and segmentation labels.
更多查看译文
AI 理解论文
溯源树
样例
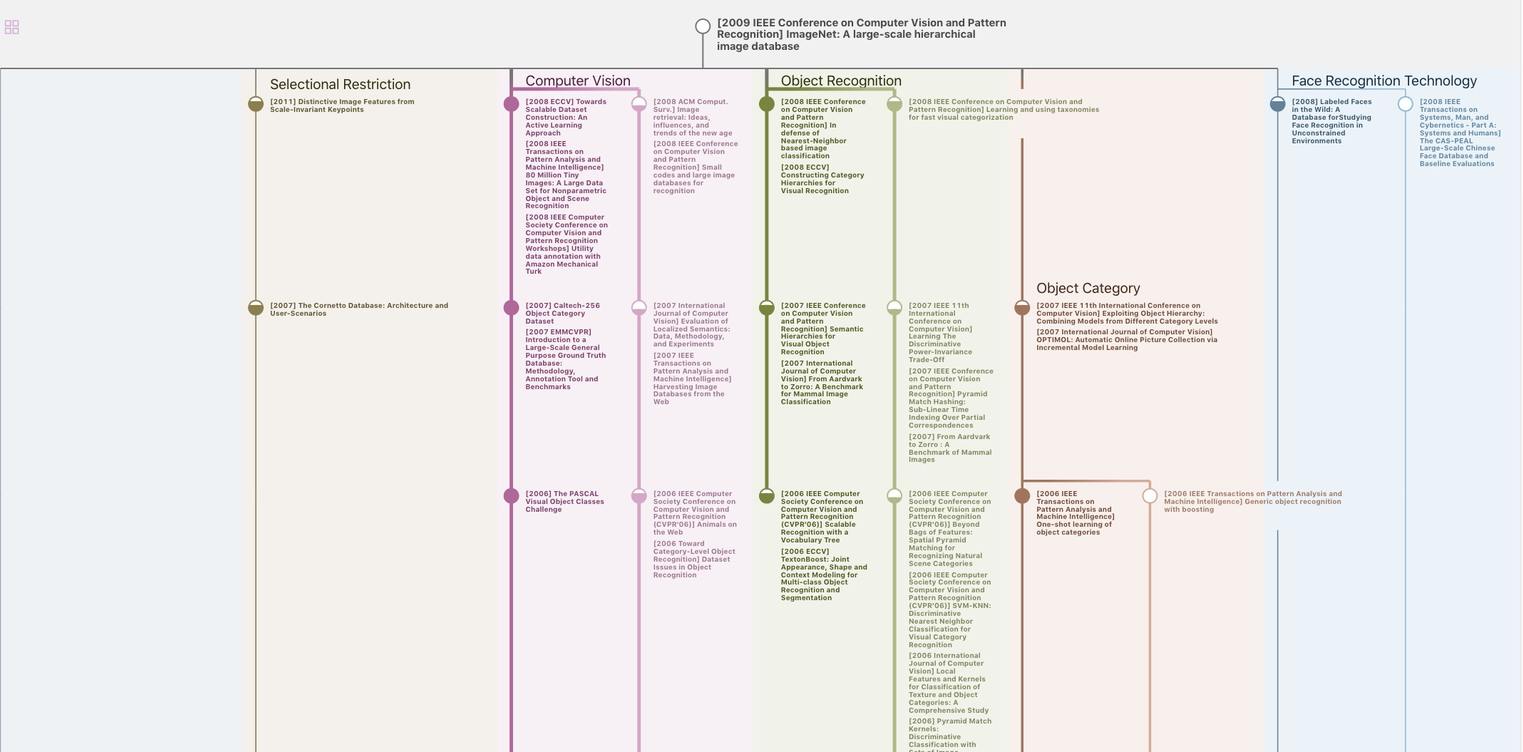
生成溯源树,研究论文发展脉络
Chat Paper
正在生成论文摘要