Deep Reinforcement Learning for Accelerating the Convergence Rate
user-5ebe282a4c775eda72abcdce(2016)
摘要
In this paper, we propose a principled deep reinforcement learning (RL) approach that is able to accelerate the convergence rate of general deep neural networks (DNNs). With our approach, a deep RL agent (synonym for optimizer in this work) is used to automatically learn policies about how to schedule learning rates during the optimization of a DNN. The state features of the agent are learned from the weight statistics of the optimizee during training. The reward function of this agent is designed to learn policies that minimize the optimizee’s training time given a certain performance goal. The actions of the agent correspond to changing the learning rate for the optimizee during training. As far as we know, this is the first attempt to use deep RL to learn how to optimize a large-sized DNN. We perform extensive experiments on a standard benchmark dataset and demonstrate the effectiveness of the policies learned by our approach. All source code for reproducing the experiments can be downloaded from https://github. com/bigaidreamprojects/qan.
更多查看译文
AI 理解论文
溯源树
样例
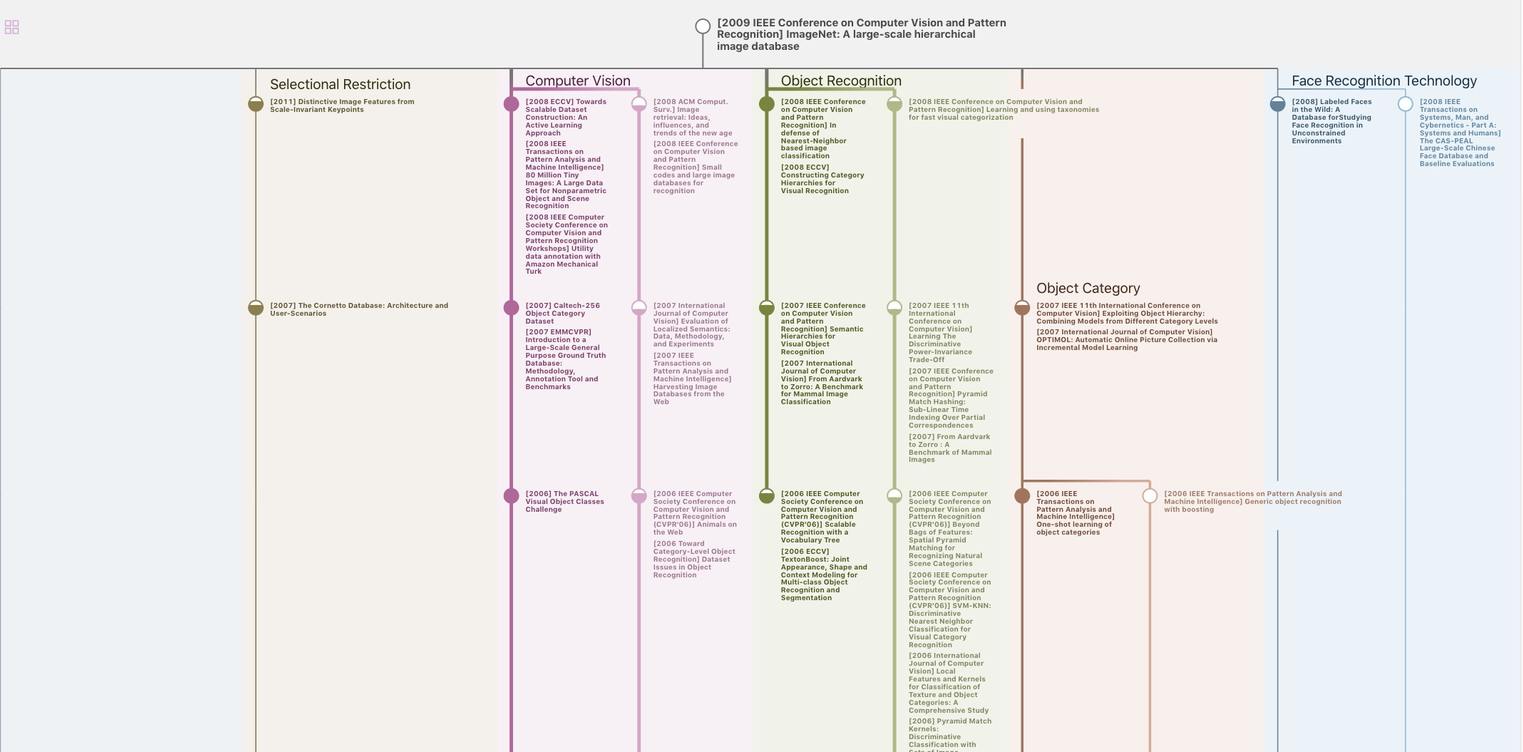
生成溯源树,研究论文发展脉络
Chat Paper
正在生成论文摘要