A Characteristic-Featured Shock Wave Indicator for Conservation Laws Based on Training an Artificial Neuron
Journal of Scientific Computing(2020)
摘要
In this work, we use exact solutions of one-dimensional Burgers equation to train an artificial neuron as a shock wave detector. The expression of the artificial neuron detector is then modified into a practical form to reflect admissible jump of eigenvalues. We show the working mechanism of the practical form is consistent with compressing or intersecting of characteristic curves. In addition, we prove there is indeed a discontinuity inside the cell detected by the practical form, and smooth extrema and large gradient regions are never marked. As a result, we apply the practical form to numerical schemes as a shock wave indicator with its easy extension to multi-dimensional conservation laws. Numerical results are present to demonstrate the robustness of the present indicator under Runge–Kutta Discontinuous Galerkin framework, its performance is generally compared to TVB-based indicators more efficiently and accurately. To treat the initial inadmissible jumps, including linear contact discontinuities and those evolving into rarefaction waves, a preliminary strategy of combining a traditional indicator in the beginning with the present indicator is suggested. We believe the present indicator can be applied to unstructured mesh in the future.
更多查看译文
关键词
Hyperbolic conservation laws,Characteristic curve,Discontinuous Galerkin,Troubled-cell indicator,Artificial neural networks,65M60,68Q32
AI 理解论文
溯源树
样例
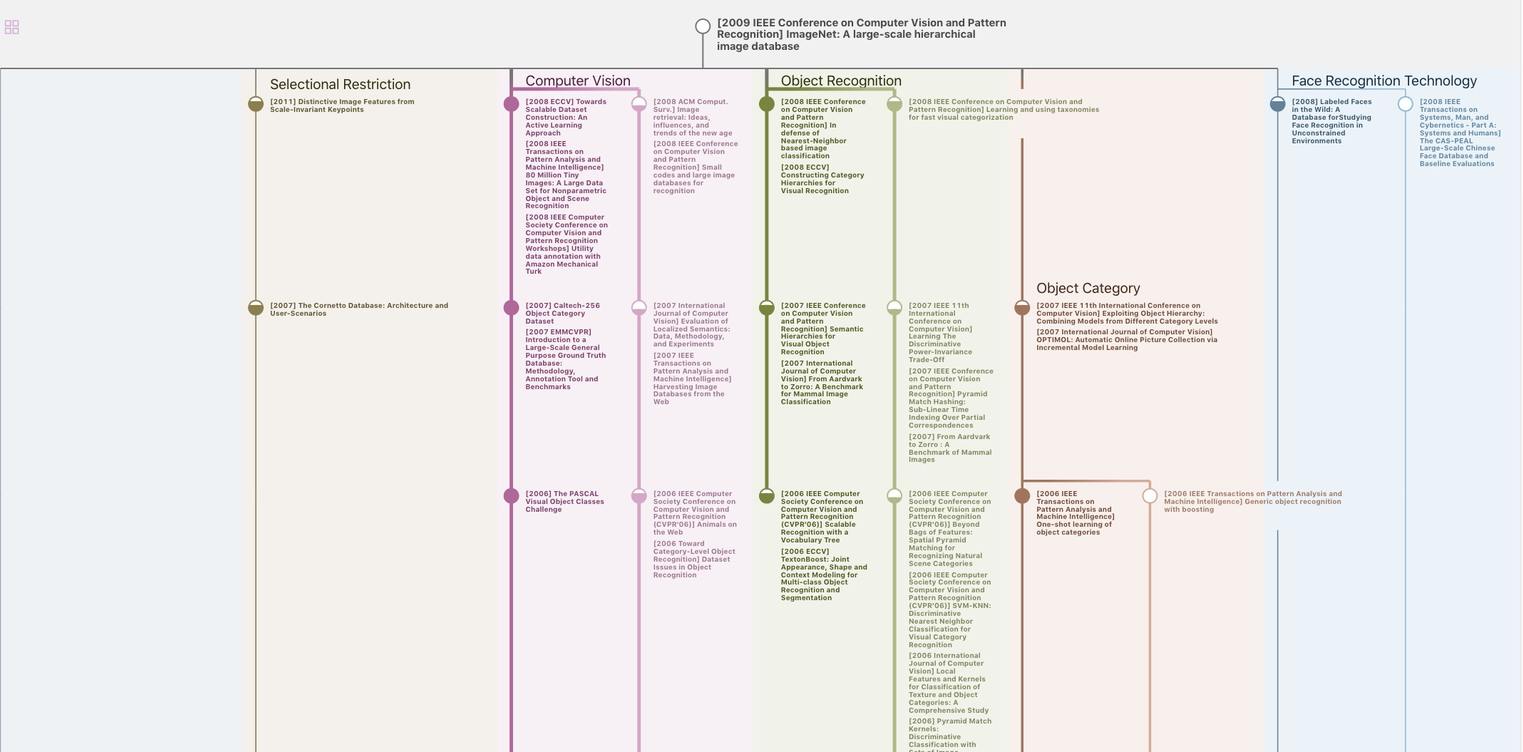
生成溯源树,研究论文发展脉络
Chat Paper
正在生成论文摘要